C-01 Council Member Kitchen Memo — original pdf
Backup
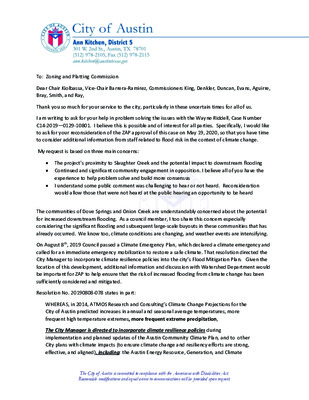
To: Zoning and Platting Commission Dear Chair Kiolbassa, Vice-Chair Barrera-Ramirez, Commissioners King, Denkler, Duncan, Evans, Aguirre, Bray, Smith, and Ray, Thank you so much for your service to the city, particularly in these uncertain times for all of us. I am writing to ask for your help in problem solving the issues with the Wayne Riddell, Case Number C14-2019—0129-10801. I believe this is possible and of interest for all parties. Specifically, I would like to ask for your reconsideration of the ZAP approval of this case on May 19, 2020, so that you have time to consider additional information from staff related to flood risk in the context of climate change. My request is based on three main concerns: • The project’s proximity to Slaughter Creek and the potential impact to downstream flooding • Continued and significant community engagement in opposition. I believe all of you have the • experience to help problem solve and build more consensus I understand some public comment was challenging to hear or not heard. Reconsideration would allow those that were not heard at the public hearing an opportunity to be heard The communities of Dove Springs and Onion Creek are understandably concerned about the potential for increased downstream flooding. As a council member, I too share this concern especially considering the significant flooding and subsequent large-scale buyouts in these communities that has already occurred. We know too, climate conditions are changing, and weather events are intensifying. On August 8th, 2019 Council passed a Climate Emergency Plan, which declared a climate emergency and called for an immediate emergency mobilization to restore a safe climate. That resolution directed the City Manager to incorporate climate resilience policies into the city’s Flood Mitigation Plan. Given the location of this development, additional information and discussion with Watershed Department would be important for ZAP to help ensure that the risk of increased flooding from climate change has been sufficiently considered and mitigated. Resolution No. 20190808-078 states in part: WHEREAS, in 2014, ATMOS Research and Consulting’s Climate Change Projections for the City of Austin predicted increases in annual and seasonal average temperatures, more frequent high temperature extremes, more frequent extreme precipitation, The City Manager is directed to incorporate climate resilience policies during implementation and planned updates of the Austin Community Climate Plan, and to other City plans with climate impacts (to ensure climate change and resiliency efforts are strong, effective, and aligned), including: the Austin Energy Resource, Generation, and Climate The City of Austin is committed to compliance with the Americans with Disabilities Act. Reasonable modifications and equal access to communications will be provided upon request. Protection Plan, the Zero Waste Master Plan, the Hazard Mitigation Plan and the Flood Mitigation plan Reconsideration will allow you to consider, during your deliberations and discussions with staff, the anticipated increased intensity of our region’s rain events and include the city’s future climate projections in estimates of runoff and risk of flooding. According to the climate change projections from the Atmos Study commissioned by the City of Austin, Dr Katharine Hayhoe noted “Heavy precipitation, measured in terms of days per year with more than 2 inches of rain and the amount of rainfall during the 5 consecutive wettest days of the year, is expected to increase”. I am also attaching a recently released Texas A&M report issued by the Office of the Texas State Climatologist that include on page 19, projections in frequency of extreme rainfall events and a subsequent expected frequency of increased runoff and urban flooding of 10-15% relative to 2000-2018. I would ask that during your deliberation you consider future climate projections in runoff estimates. Please also strive to identify and discuss strategies to best address flooding concerns to the Slaughter and Onion Creek watersheds and address those and other concerns with the community. Your ability to help resolve these kinds of complex cases and build consensus is invaluable to Council and very much appreciated. There is no doubt these are challenging times as we all strive to maintain our public services and processes. I appreciate the work that each of you do, and don’t normally request this type of action from our commission members. I am making this unusual request because of the importance of these concerns and your unique position in addressing these issues. I would appreciate your reconsideration of this matter. Highest Regards to you all for your service, Ann Kitchen Council Member, District 5 The City of Austin is committed to compliance with the Americans with Disabilities Act. Reasonable modifications and equal access to communications will be provided upon request. TEXAS A&M UNIVERSITY Office of the Texas State Climatologist ASSESSMENT of HISTORIC and FUTURE TRENDS of EXTREME WEATHER IN TEXAS, 1900-2036 1 Cover photo: The San Bernard River became a tree-lined field during the 2011 drought. Photo by John Nielsen-Gammon REPORT AUTHORS John Nielsen-Gammon, Department of Atmospheric Sciences, Texas A&M University Jacob Escobedo, Department of Atmospheric Sciences, Texas A&M University Catherine Ott, Environmental Programs, College of Geosciences, Texas A&M University Jeramy Dedrick, Department of Atmospheric Sciences, Texas A&M University Ali Van Fleet, Department of Atmospheric Sciences, Texas A&M University MARCH 5, 2020, DOCUMENT OSC-202001 INTRODUCTION Texas is vulnerable to a wide range of natural hazards, most of which are associated with weather and climate events. The natural environment has evolved partly in response to these natural hazards. For example, plant hardiness is largely determined by ability to survive extreme winter cold and drought. The built environment, including for example homes, roads, and power plants, is designed to a certain level of resiliency to natural hazards. Human activities as fundamental to survival as food production and water supply are tailored to the particular combination of weather and climate risks at play in a given location. The future of Texas depends on its resilience to the natural hazards of the future. It is up to Texans, both individually and collectively, to decide what level of resilience is appropriate, and at what cost, compared to the costs of damage and recovery on both an economic and societal level. Nobody knows which specific weather and climate events will befall Texas over the next couple of decades. But a wide variety of information can be used to estimate the risks of certain types of weather and climate events over that period. The standard practice for estimating the risk of natural hazards has been to assume that future risk is equal to historical risk. This practice works if the underlying climatic conditions are unchanging. However, Texas climate is affected by changing patterns of vegetation, irrigation, and urbanization. Texas climate is also embedded in the global climate system, which is itself changing. All of these factors have influenced historical trends in weather and climate extremes and will continue to influence trends in the future. Given a changing climate, historic trends may provide a better guide to future risk than mere historical averages. The sponsors of these projections requested that projections be based primarily on existing trends. Doing so makes sense only if the causes of those trends are understood and are expected to continue. The scientific understanding of the causes of trends draws upon a large body of research, utilizing both observations and experiments with global climate models. This report presents trends for a variety of historic periods, and the projections are based on historic trends that are expected to continue according to currently available science. 1 Several factors influence our ability to project historical trends into the future. First, historical data may not be sufficiently accurate or consistent over time to yield reliable trends. Second, natural climate variability and the randomness of extreme weather events can mask or even overwhelm any underlying long-term trends. Despite these limitations, there are sound reasons to expect continued change in a variety of aspects of extreme Texas weather, and knowledge of such likely changes can be very useful in a variety of planning contexts. Trends are not the only potentially useful historic information. Some natural climate variations that occur on multidecadal time scales have a substantial effect on Texas weather. The present-day scientific ability to accurately predict such variations twenty years into the future is quite limited. Nonetheless, knowledge of these variations informs the understanding of past trends and suggests whether recent weather patterns are representative of typical future conditions. This report addresses historical and future trends in extreme temperatures, extreme precipitation, severe thunderstorms, and hurricanes. It also addresses trends in drought, floods, wildfire, and coastal erosion, to the extent that these natural hazards are affected by changes in weather and climate. For each natural hazard, the report considers the quality of the historical data, the historical risk and trends (data permitting), the causes of any observed or expected trends, and the projection of trends of future risk. For context, this report also considers trends in annual average temperature and precipitation. Expected typical conditions in 2036 are expressed as a change compared to average conditions in 1950-1999 and 2000-2018. With all projections, there is considerable uncertainty as to how things will actually turn out. This report does not attempt to quantify that uncertainty; prudent planning recognizes that we cannot know whether reality will end up higher or lower than the best available present-day estimates. This report was commissioned and sponsored by Texas 2036. The report content is solely the responsibility of the authors. A previous version of this report has been peer-reviewed; reviewer comments and responses are available from the Office of the State Climatologist. The analyses that are original to this report are based on data from publicly accessible data sources; analysis spreadsheets and software are available from the Office of the State Climatologist. 3 AVERAGE TEMPERATURES While average temperatures do not themselves constitute weather and climate extremes, changes in average temperatures, either locally or globally, affect many aspects of extreme weather and climate trends. In addition, all else being equal, a change in average temperature would lead to a change in frequency of extreme temperatures, increasing hot extremes and decreasing cold extremes. The National Centers for Environmental Information maintain very good analyses of monthly averages of daily maximum and minimum temperatures from 1895 to present throughout the lower 48 states.2 The annual average temperature in Texas over the period 1895-2018 exhibits year-to-year variations of 2 °F or more. Broadly speaking, Texas temperatures climbed gradually until the mid-1950s, declined until the late-1970s, and rose thereafter. The rate of temperature increase since 1895 has averaged 0.11 °F per decade, less than the global average of 0.14 °F per decade.3 Indeed, the southeastern United States, including eastern Texas, is almost the only land area on the globe whose temperature increase over the 20th century was nearly zero.4 More recently, the Texas temperature trend has been larger. Since 1950, the trend is 0.26 °F per decade, and since 1975, 0.63 °F per decade. Recent temperatures have increased in all seasons and in all regions of Texas. The historic Texas temperature trend simulated by global climate models since 1950 is 0.29 °F per decade, and since 1975, 0.51 °F per decade.5 The simulated current rate of increase in Texas, based on climate model simulations and projections for 2000-2040 for the low-emissions pathway RCP 4.56, is around 0.64 °F per decade. Up to mid-century, climate projections are not very sensitive to the choice of emissions pathway.7 On the whole, the agreement between models and observations is decent. Factors that cause observed trends to differ from simulated trends include inadequacies in the models, inaccuracies in observations, natural variability, and local land surface changes such as irrigation and afforestation. Historical data and climate models lead to similar conclusions. If recent trends continue, as expected, a middle-of-the-road estimate of the overall rate of temperature increase in Texas would be about 0.6 °F per decade. This means that average Texas temperatures in 2036 should be expected to be about 1.6 °F warmer than the 2000-2018 average and 3.0 °F warmer than the 1950-1999 average. This would make a typical year around 2036 warmer than all but the absolute warmest year experienced in Texas during 1895-2018.8 Temperature trends since 1975 have been similar in all seasons except for summertime temperatures, which have been increasing at a slower rate than those in other seasons. The 1950-2018 July-August trend is 0.10 °F per decade, while the 1975-2018 July-August trend is 0.45 °F per decade. It is not known whether this slower trend is due to historical factors that will continue or that have already played out. Possibly the transition from open grassland to shrubland and forest across much of Texas over the past century has led to a reduced summertime warming trend.9 If so, since that transition seems largely complete, an assumed future summer trend similar to that of the other three seasons seems reasonable. 4 Right: Temperature trends since 1975 according to NCEI nClimDiv data. Bottom left: Texas annual average statewide temperatures (°F). Bottom right: Comparison of observed and simulated temperature trends in Texas. 68 67 66 65 64 63 62 Temperature Trends, 1975-2018 Temperature (°F Increase/Decade) ± 0.80 - 0.85 0.75 - 0.80 0.70 - 0.75 0.65 - 0.70 0.60 - 0.65 0.55 - 0.60 0.50 - 0.55 0.45 - 0.50 3 e d a c e D r e p t i e h n e r h a F s e e r g e D 1.2 0.9 0.6 0.3 0 -0.3 ANN Trend since 1950 Trend since 1975 Trend since 1895 Simulations Observations Texas Average Temperature Trends 1895 1915 1935 1955 1975 1995 Texas annual average statewide temperatures (°F) 2015 1895-2018 1950-2018 1975-2018 2000-2040 5 EXTREME TEMPERATURES The projected changes in average temperature imply changes in unusually high or low temperatures as well. This assessment of extreme temperatures relies on two aggregated sets of temperature data: a set of stable urban, semi-urban, and rural stations (hereafter referred to as index stations)10 and a set with one composite station per county.11 In Texas, a good benchmark for extreme heat is the number of 100+ °F days in a given year. The number of 100-degree days is closely related to the average summertime temperature. At rural and semi-urban index stations, where 2000-2018 July-August average temperatures average around 83 °F, there are typically about 12 days per year that reach or exceed 100 °F. If summertime temperatures rise at a similar rate as the projected annual Texas average, the typical number of 100-degree days would nearly double, to about 21 per year, by 2036. 60 45 30 15 s y a D F ° 0 0 1 f o r e b m u N e g a r e v A 0 78 80 82 84 86 Average July-August Texas Temperature 88 Historical relationship between summertime average temperature and number of 100°F days at selected long-term non-urban stations in Texas To analyze actual trends in 100 °F days in Texas, the index stations in each station class were grouped into four regions and conditions were averaged across stations within each class and region, then the values from each region were averaged together to obtain statewide averages by class.12 Because the above graphic shows that 100 °F day counts are a nonlinear function of temperature and because counts cannot be negative, trends were analyzed on the basis of a linear fit to the time series of the logarithm of annual 100 °F day counts. A plot of number of days reaching 100 °F at index stations shows some memorably hot summers: 1980, 1998, and 2011. Over the past 40 years, the linear trend shows a more than doubling of the number of triple-digit days at all types of stations, although the longer trend since 1950 generally has less than a doubling. Thus, a near-doubling of the number of 100-degree days between 2000-2018 and 2036 appears to be a reasonable projection. Urban stations, which are in major metropolitan areas, have seen a more dramatic increase in 100-degree days. The frequency has approximately tripled, whether one considers the shorter 1975-2018 period or the longer 1950-2018 period. There are presently more 100-degree days in major metropolitan areas than elsewhere. This suggests that the urban heat island effect has enhanced the risk of extreme heat in major cities in Texas, above and beyond the trend due to larger-scale climate change. 5 6 Days Over 100°F 37 33 57 44 38 30 25 20 15 10 5 0 1950 1960 1970 1980 1990 2000 2010 2020 Urban Stations Semi-Urban Stations Rural Stations Trend Since 1950 Trend Since 1950 Trend Since 1950 Trend Since 1975 Trend Since 1975 Trend Since 1975 Trends and historic variability in index temperature stations in Texas, grouped by amount of urbanization. Arrows at top indicate data points that are above the margin of the graph. Linear trend lines are fit to the logarithm of 100 °F day counts to ensure non-negative values. Since 1975, 100 °F days have increased at least as rapidly at rural stations as at urban stations. The excess heat at rural stations has primarily occurred during the most extreme summers (1998, 2000, 2009, 2011), while urban stations tend to have the most 100 °F days during less extreme summers. This is consistent with evidence suggesting that, as temperatures increase, the drying of rural soils can lead to an excessive increase in daytime temperatures,13 while urban moisture varies less due to large expanses of impervious surfaces. An alternative way of examining extreme heat trends is to consider the average hottest day in each month during June-September in each county in Texas.14 This metric is more sensitive to exceptional heat events than to sustained heat. It also enables us to use longer historical records, as this metric is not sensitive to changes in observing time. Looked at this way, there is a very slight downward trend in extreme heat over the long term. This trend reflects the general pattern over the continental United States, where the most exceptional heat occurred back in the 1930s.15 The cause of this downward trend, which is in contrast to mean and extreme temperatures in all other seasons, may be related to the land cover trends such as were mentioned earlier.16 Whatever the cause of the long- term slight downward trend, it appears to have reversed, with a slight increase in extreme monthly heat in recent decades. Extreme low temperatures during the winter months exhibit a stronger and more robust trend.17 Despite year- to-year fluctuations that are much larger for extreme cold than extreme heat, there is a long-term warming trend in monthly extreme cold temperatures across Texas, and the trend is larger in recent decades. Extreme cold at urban index stations has warmed at a greater rate than at rural index stations. At all time periods, extreme cold is warming fastest, while extreme heat is warming slowest. This is broadly consistent with expectations: extreme cold air comes from the Arctic, which in general is warming faster than other parts of the globe. There have been some studies in recent years debating whether loss of Arctic sea ice and overall Arctic warming leads to changes in weather patterns that favor more intense incursions of Arctic 6 7 air18, but this tendency, if present, has not been strong enough in Texas to stop the accelerating rise of extreme cold temperatures. This warming of extreme cold temperatures in Texas, much more rapid than warming of average temperatures, is consistent with what has been observed elsewhere in the Northern Hemisphere and is inconsistent with the argument that loss of Arctic sea ice is enhancing extreme cold over the continents.19 Note that there has not been a winter with extreme cold temperatures above 24 °F in over two decades, despite the upward trends, which could be a statistical aberration or a consequence of the aforementioned changes in weather patterns. Overall, extreme heat is becoming more frequent and more severe, while extreme cold is becoming less frequent and less severe. The temperature extreme projections here are based on the 1975-2018 trends, since temperature trends themselves are well simulated over that period by climate models and are projected by models to continue at a similar pace. The number of 100-degree days at typical stations is expected to nearly double by 2036 compared to 2000- 2018, with a typical year having 25 triple-digit days at urban and rural stations and 20 triple-digit days at semi-urban stations. Triple-digit counts will tend to be larger toward the south and away from the coast, and smaller elsewhere. Extreme monthly summertime temperatures averaged 97.8 °F during 1950-1999 and 98.3 °F during 2000-2018. Based on the 1975-2018 trend, the expected value of extreme monthly summertime temperatures would be 98.9 °F by 2036, an increase of 0.6 °F compared to the 2000-2018 average and 1.1 °F compared to the 1950- 1999 average. Meanwhile, extreme monthly wintertime temperatures averaged 18.5 °F during 1950-1999 and 20.8 °F during 2000-2018. Based on the 1975-2018 trend, extreme wintertime temperatures would increase 3.3 °F by 2036 compared to the 2000-2018 average and 5.6 °F compared to the 1950-1999 average. Trends in extreme cold are much larger than trends in extreme heat, which is leading to an overall decrease in the range of annual temperature extremes over time. That decrease is likely to continue. The extreme heat projections remain largely within the range of historical variability, but the increase in the number of hot days is such that the typical number of triple- digit days by 2036 is projected to be larger than all but four years since 1950.20 Meanwhile, the expected warming of extreme wintertime temperatures would make typical wintertime extremes by 2036 milder than all but five of the winters in the historic record.21 Also, trends in extreme temperatures in urban areas are generally as large or larger than those in rural areas, so that extreme heat is an increasing large risk in urban areas and extreme cold is an increasingly remote one. 8 Extreme JJAS Temps 1950-2018 1975-2018 1895-2018 1890 1900 1910 1920 1930 1940 1950 1960 1970 1980 1990 2000 2010 Hottest day of the month, June-September, at composite county stations across Texas, with linear trends beginning in 1895, 1950, and 1975. Extreme DJF Temps 1950-2018 1975-2018 1895-2018 1890 1900 1910 1920 1930 1940 1950 1960 1970 1980 1990 2000 2010 trends beginning in 1895, 1950, and 1975. Average coldest day of the month, December-February, at composite county stations across Texas, with linear Extreme Cold Average Extreme Heat 7 110 106 102 98 94 90 28 24 20 16 12 8 1.2 0.9 0.6 0.3 0 e d a c e D r e p t i e h n e r h a F s e e r g e D -0.3 1895-2018 8 1950-2018 1975-2018 county data Comparison of the sizes of the trends in average temperatures and extreme heat and cold in the 9 PRECIPITATION Precipitation in Texas is quite variable, both in space and time. Much of the state has two rainy seasons, with the rainiest months on average being May, June, September, and October. In far West Texas, the wettest months are July and August, while far East Texas averages similar amounts in every month. Rainfall amounts increase from west to east, with the southeast corner of the state near Beaumont averaging over eight times the annual rainfall of some areas near El Paso. The long-term trend of precipitation in Texas has been positive. Over the past century, parts of central and eastern Texas have experienced precipitation increases of 15% or more, while in much of the western part of the state the long-term trend is flat or even downward.22 The tendency for increasing precipitation in Texas is not consistent with the majority of global climate models, with the average simulated trend being -2.6% per century.23 Models and observations both tend to feature more positive (or less negative) trends toward northeastern Texas than toward southwestern Texas.24 Superimposed on the generally upward precipitation trend is considerable variability. El Niño has a prominent influence on cool-season rainfall in Texas: during El Niño years, Texas tends to be wet, while during La Niña years, Texas tends to be dry. Individual El Niño or La Niña events tend to last only 1-3 years, so this relationship is not useful for multidecadal predictions. However, both the Atlantic and Pacific Oceans feature multidecadal variability: the Atlantic Multidecadal Oscillation (AMO) and the Pacific Decadal Oscillation (PDO). Both have been shown to substantially influence precipitation in the United States, including Texas.25 Precipitation Trends, 1895-2018 ± Precipitation (% change/century) 15.0 - 21.0 10.0 - 15.0 5.0 - 10.0 0.0 - 5.0 -6.3 - 0.0 Precipitation trends since 1895 according to NCEI nClimDiv data. 10 Texas Decadal Precipitation and Natural Drivers 25 20 15 10 5 0 1900 1920 1940 1960 1980 2000 2020 MAMJ MAMJ JASO JASO NDJF NDJF -AMO M-O -AMO M-O PDO N-J PDO N-J Solid: Average Texas precipitation, inches, nine-year running averages, for March-June (green), July-October (orange), and November-February (gray), with dots showing values for last nine individual years. Dashed light green: Pacific Decadal Oscillation, November-June, nine-year running average, with squares showing values for last nine individual years. Dashed dark red: Atlantic Multidecadal Oscillation, March- October, sign reversed, nine-year running average, with squares showing values for last nine individual years. Both AMO and PDO have been rescaled and offset for ease of comparison with Texas precipitation.26 The graph of decadal-scale variations of average Texas precipitation illustrates the distinct seasons during which the AMO and PDO influence Texas precipitation. The AMO was relatively high in the 1930s, 1950s, and 2000s and low in the 1900s, 1970s, 1980s, and early 1990s. The negative of the AMO is plotted here so that upticks in the graph correspond to upticks in Texas precipitation. The AMO pattern can be detected in the March-June precipitation (correlation = -0.27) and July-October precipitation (correlation = -0.47). Meanwhile, the PDO was relatively low near 1920, 1950, and 2010, and relatively high near 1940, 1980, and 1990. This pattern can be detected in the November-February precipitation (correlation = 0.53) and March-June precipitation (correlation = 0.54). So the AMO influences Texas precipitation on a decadal scale from spring to fall, while the PDO influences Texas precipitation from late fall through spring. The decadal-scale variability is so large that the eye has difficulty detecting the aforementioned long-term upward trend in Texas precipitation. Over the next couple of decades, this implies that variability is likely to have a far larger effect on precipitation changes than any long-term climate trend. Unfortunately, neither the AMO nor PDO can reliably be predicted two decades into the future. The last nine years illustrates that the PDO is much more erratic than the AMO. Also, given the extreme annual precipitation in some recent years and the dry year of 2011, the nine-year precipitation averages will almost certainly exhibit a short-term upward blip. Both the AMO and PDO favored unusually dry conditions in Texas during the first part of the 21st century. Since that pattern is unlikely to recur given the slow variability of the PDO and AMO, the expectation is that rainfall in Texas over the next two decades will tend to be greater than what was experienced from 1998 to 2012. The positive long-term precipitation trend further supports that expectation. The climate model projections provide weak evidence for a precipitation decline. Also projected is a change in the seasonality of rainfall in North Texas, with less rain in the summer.27 11 11 EXTREME RAINFALL Many studies have documented an increase in extreme rainfall in Texas and surrounding areas for a variety of durations and thresholds.28 On average across the region, extreme one-day precipitation has increased by 0.2” to 0.5” since the middle of the 20th century.29 Within Texas, the local experience of extreme rainfall varies widely from place to place, with some locations having experienced a decrease in intensity of extreme rainfall over the period of data availability while the majority of locations have experienced an increase.30 The median change since 1960 is an increase in intensity of 7%. All other things being equal, an increase in overall precipitation amounts would be expected to lead to an increase in extreme precipitation amounts, which inevitably implies an increase in the frequency of extreme precipitation above a given threshold. So the overall trend in Texas precipitation, discussed previously, contributes to the observed trend in extreme precipitation probabilities. In addition to the overall precipitation effect, extreme rainfall is strongly affected by increased temperatures. A column of air that is producing rainfall will produce about 4% more rainfall for every °F of warming.31 The extra precipitation intensity can also affect storm structure, and climate change can alter weather patterns and the frequency of dangerous storms. The direct temperature effect appears to be most important over the long haul in most midlatitude locations such as Texas. Changes in storm motion and frequency are expected to be comparatively subtle.32 As noted earlier, computer model projections of overall rainfall amounts in Texas are somewhat inconsistent, but in general they show an overall leveling off or slight decrease of precipitation amounts.33 This suggests that trends of extreme precipitation in the future will be dominated by the increasing temperature effect and changes in storm structure. The strongest influence should be temperatures near the moisture source for Texas extreme precipitation, that is, the tropical oceans and the Gulf of Mexico. Tropical ocean temperatures are not expected to rise as quickly as temperatures over land in Texas, but would still be sufficient to produce an additional 2%-3% increase in extreme rainfall intensity from the temperature effect alone.34 So extreme rainfall intensity and frequency are projected to continue increasing, though probably not as rapidly as they have increased in the past. Rainfall risk is often characterized in terms of the 100- year rainfall event, which is an amount of rain over a given duration that has a 1% chance of occurring in any given year. If extreme rainfall amounts increase by just 1220%, the 100-year rainfall event threshold is exceeded twice as often.35 So Texas’s 7% median increase in the 100-year rainfall amount between 1960 and 2017 corresponds to a roughly 30% increase in the frequency of heavy rainfall exceeding the older 100-year threshold. Based on projected temperatures and the dominance of the direct temperature effect on extreme rainfall, we anticipate an additional increase of 2%-3% in expected extreme rainfall intensity in 2036 compared to 2000-2018 and an overall increase of 6%-10% compared to 1950- 1999. These changes in amount correspond to increases in the odds of extreme precipitation of 10%-15% and 30%-50%, respectively. Note that the variations of extreme rainfall trends across Texas imply that one should not assume that recent extreme rainfall history in a given location is a suitable baseline for projecting future trends. While estimates of extreme rainfall risk based on historical data show a large uptick in Houston but little change in Dallas-Fort Worth36, climate change should be acting to increase the risk more uniformly across the entire state. The projected increase of extreme rainfall risk given above is relative to the expected past risk of extreme rainfall in a given location, not the actual occurrences of extreme rainfall in that location. Likewise, the actual extreme rainfall over the next 20 years at any given location may defy the odds, either favorably or unfavorably. 100 Year Return Period, One-Day Rainfall ± Percent Change, 1960-2017 -10 - 0 -20 - -10 < -20 Insufficient Data > 40 30 - 40 20 - 30 10 - 20 0 - 10 Percentage change in the intensity of one-day rainfall with a 1% probability of occurring in any given year, based on a time-dependent statistical fit of annual daily precipitation maxima at composite stations. 12 13 DROUGHT At first glance, the long-term increase in average rainfall should imply a decrease in drought as well. The linear trend in total statewide precipitation since 1895 is +0.85% per decade, since 1950 is +2.34% per decade, and since 1975 is +0.87% per decade.37 The large trend since 1950 is due to the extended dry conditions beginning around 1950, especially elevating the trend since 1950 because of such a low starting point. Texas Statewide Precipitation The corresponding drought severity based on precipitation alone, as measured by the cumulative 9-month Standardized Precipitation Index, does indicate declines in severity. The change in cumulative dryness is -6.0% per decade since 1896, -14.4% per decade since 1950, and +15.7% per decade since 1975. As noted with respect to precipitation, the change since 1950 is large in part because of the extended drought that occurred early in the 1950s. The rise of cumulative dryness since 1975, however, has happened despite a slight increase of precipitation over the same period. The explanation for this difference can be seen in the statewide precipitation graph. Note that the period of time from 1965 to 1985 featured very little precipitation variability, with statewide average precipitation ranging between 22” and 35”. Since then, precipitation has become more variable, with 2011 precipitation below 14” and 2015 precipitation above 41”. Greater precipitation variability leads to more intense droughts even if the overall precipitation amount doesn’t change. 1895 1905 1915 1925 1935 1945 1955 1965 1975 1985 1995 2005 2015 Statewide average precipitation, from NCEI’s nClimDiv dataset, with linear trends since 1895, 1950, and 1975. ) s e h c n i ( n o i t a t i p i c e r P 45 40 35 30 25 20 15 10 5 0 14 9-Month SPI: Overall Yearly Drought Severity 1890s to 2018 1950 to 2018 1975 to 2018 40 30 20 10 0 -10 -20 -30 -40 2.5 1.5 2 1 0.5 0 1896 1906 1916 1926 1936 1946 1956 1966 1976 1986 1996 2006 2016 Statewide annual cumulative drought severity, with linear trend lines beginning in 1896, 1950, and 1975. 38 The historic record of precipitation variability is, itself, quite variable, with no clear trend over the period of record despite the recent uptick in variability. Climate models generally simulate an increase in interannual precipitation variability in Texas since 1950 but little or no change in interannual precipitation variability going forward.39 The lack of future variability is itself anomalous compared to the rest of the Northern Hemisphere land areas, which tend to show an increase in variability in excess of the increase in average precipitation itself.40 So the recent trend of increased interannual variability is probably not going to continue apace through 2036, unless average precipitation itself continues to increase, and the precipitation variability experienced over 2000- 2018 is probably representative of what should be expected through 2036. 14 Temperature also affects drought directly, by increasing the rate of evaporation from the soil and from water bodies. Many drought indices, such as the Palmer Drought Severity Index, attempt to include the effect of temperature on dryness.41 Compared to rainfall-only SPI indices at various time scales, particularly the 9-month scale that the PDSI is most responsive to, the PDSI shows a less negative trend over the entire period and a larger positive trend recently.42 3-Month SPI 9-Month SPI 24-Month SPI PDSI Comparison of short-duration (three months), medium-duration (nine months), and long-duration (24 months) cumulative annual drought severity based on precipitation, as quantified using the Standardized Precipitation Index (SPI). Also shown are trends in the cumulative drought severity based on the Palmer Drought Severity Index (PDSI), whose variations are most strongly correlated with the 9-month SPI. 15 However, while the SPI neglects the temperature effect on drought entirely, the PDSI may overestimate it.43 This is because, in addition to precipitation and temperature, carbon dioxide also affects drought. Elevated carbon dioxide levels improve the water use efficiency by plants, so would lead to increased soil moisture and decreased drought. Elevated carbon dioxide levels also increase biomass if plants are not otherwise water- or nutrient- limited, which might increase water use and decrease soil moisture. While these two effects work in opposite directions, the water use efficiency effect seems to be dominant, thereby allowing increased CO2 to lead to improved plant growth despite meteorological factors leading to increased drought, at least on a global scale.44 Nonetheless, improved plant health overall does not eliminate possible detrimental effects from drought accompanied by increased temperatures, particularly since decreased plant water use itself implies increased temperatures.45 Also, different plants respond to carbon dioxide (and heat stress) differently.46 Lastly, global climate model simulations that attempt to incorporate changes in plant physiology still indicate a substantial decrease in future soil moisture across the Great Plains.47 Increased carbon dioxide does not reduce the temperature effect of evaporation from lakes and reservoirs or from bare soil. Historically, there has been an increase in the evaporative capacity of the atmosphere across most of Texas, especially in West Texas and the Panhandle, and that trend is expected to continue due to robust projections of rising temperatures.48 A continuation of the observed trend would lead 15 to a roughly 4% increase in expected summertime evaporative losses from reservoirs in 2036 compared to 2000-2018.49 For hydrological drought, runoff is important. Runoff changes depend on changes in soil moisture and changes in precipitation amount and intensity. Across the midlatitudes, there is on average a decrease of topsoil moisture but little change in deep soil moisture.50 This would favor a slight decrease in runoff for a given amount of precipitation. Countering that is the tendency for greater rainfall intensity,51 which would lead to a greater runoff fraction and in turn would partially cause the decrease in soil moisture, while a model-simulated change in precipitation seasonality in some areas could lead to greater drying in summer when drought is most severe.52 Despite all the nuances discussed above, the primary driver for changes in future drought remains changes in overall precipitation amount. In Texas, the projected slight decrease mentioned in the previous section would imply a small increase of drought intensity by itself. For agricultural drought, this small increase of meteorological drought could easily be neutralized by improved water use efficiency by plants, leading to no agricultural drought trend or even a reduction in drought susceptibility, though several other factors will come into play, not least the increasing temperatures. For hydrologic drought, the increase of surface water evaporation would worsen the impact of the increased meteorological drought. Because of all the factors at play, it is impossible to make quantitative statewide projections of drought trends. The majority of factors point toward increased drought severity. Nonetheless, it seems highly likely that any such underlying trend will be dwarfed by the impact of multidecadal variability, which historical records show is large for Texas. Also, as indicated by paleoclimate records, worse droughts have occurred in Texas than the climate data record alone would indicate.53 16 17 RIVER FLOODINGTexas has been impacted greatly by river flooding in the past, causing both fatalities and economic damage. In fact, Texas ranked highest amongst the United States in flood related fatalities during 1959-2005.54 Throughout most of the state, intense daily and weekly precipitation events are the primary meteorological drivers correlated with the most extensive flooding.55 This would imply that a single or a short series of extreme precipitation events plays a dominant role in causing river flooding, and that antecedent soil conditions have limited impact. However, east and northeast Texas are the exception to this pattern, as extended wet periods are the primary meteorological drivers best correlated with extensive river flooding. This would imply that antecedent soil conditions- as controlled by seasonal precipitation and evaporation- play a dominant role in flood events in those areas. Research has found a historical decrease in the magnitude of flood events at many river gauges, resulting from abrupt drops in flood magnitude.56 This can be attributed to the construction of reservoirs and dams for flood management throughout the 20th century. As substantial future reservoir construction is not anticipated, this trend driver will not continue. Projected increases in temperatures and precipitation intensity will have competing effects on river flooding. Increased precipitation intensity will lead to more precipitation-runoff events that suggest more river flooding in the future. In contrast, increased temperatures would mitigate flooding by decreasing soil moisture and increasing the capacity for soil to hold new rainfall. This would limit the amount of precipitation-runoff that would go into rivers and increases in river streamflow would be lower. At the seasonal scale, there are mixed historical trends, with increased peak streamflow in the winter and decreased peak streamflow in the fall and spring that vary by climate zone.57 For the Trinity and Neches River Basins, both in east Texas where annual precipitation is greatest within the state, most gauges show statistically significant increases in winter extreme streamflow post-1965. While the other three seasons do not have statistically significant trends, there is still a general increasing trend in extreme streamflow for the Neches and Trinity river gauges. For the Colorado and Brazos River Basins (where annual precipitation decreases towards the west), there is an increasing trend in extreme streamflow in the winter since 1965, however, the spring and fall show a general decreasing trend. In summary, river flooding in Texas is projected to have no substantial change through 2036. This is in large part due to the construction of dams and reservoirs for flood management in the 20th century. There is a mixture of historical trends categorized by season, and this does not bring forth a clear and coherent trend to project. Also, meteorological drivers of river flooding (increased rainfall intensity, decreased soil moisture) are projected to have competing influences. On balance, if an increasing trend is present in river flooding, it will be at the most extreme flood events or in the wettest parts of the state where there is so much rainfall that a decrease in soil moisture would have little mitigating impact. Location and the decade of occurrence of stations that experienced an abrupt change in the mean of annual maximum floods. Of these, 22 out of the 24 experienced a decrease in the mean. (Adapted from Figure 4 of Villarini and Smith, 2013) Panels a) and b) shows the locations of selected river gauges relative to its river basin and climate zone, and c) shows the statistical trends of 1-day 95th percentile seasonal streamflow trends of each gauge after 1965. A Mann Kendall statistic greater than 1.96 indicates a significant increasing trend while a Mann Kendall statistic less than -1.96 indicates a significant decreasing trend. (Adapted from Figures 1 and 14 of Mishra et al., 2011) 16 18 a)b)c)URBAN FLOODING Urban flooding differs from river flooding in that catchments tend to be much smaller and the effects of urbanization are relevant for the projection of flooding in metropolitan areas. In general, urbanization greatly decreases or even eliminates the infiltration rates of the soil through the construction of impervious surfaces. For a given precipitation event, this greatly increases the precipitation runoff within a given basin and results in higher streamflow and flooding for urban rivers. Mitigation measures, such as detention ponds, can wholly or partly counter this effect. Historic trends of urban flooding are variable and determined by local flood control factors. The distribution of impervious surfaces within a river basin, pre-existing land surfaces, alterations of land surfaces, pre-existing flood control impoundments that alter runoff flow, the terrain within a basin, etc. all vary from city to city in Texas. Nearly all metropolitan areas in the state contain gauges with increasing, decreasing, and neutral trends in flooding, the majority of those being neutral.58 The degree of increasing and decreasing trend gauges varies from city, with the Houston metropolitan region standing out as a hotspot for increasing urban flooding trends (with over 60% of Texas’s metropolitan increasing trend gauges in Harris County alone), whereas decreasing trends are spread among many metropolitan regions. A recent study has argued that the mere existence of Houston has intensified rainfall and increased streamflow twentyfold, but those findings may not be robust.59 Local trends in the future may not necessarily reflect what has happened in the past. Many of the decreasing trend gauges are generally the gauges with a comparatively longer record of data (over 50 years) which are usually on major rivers which have been affected by dam/reservoir construction over the record period.60 As mentioned in the river flood section, little substantial reservoir construction is anticipated in the future. In addition, many of the increasing trend gauges tend to have a comparatively shorter record of data (25-50 years) where natural variability will have a larger influence on the calculated trend. Regardless of the variable historical trends, Texas’s urban population has increased by over 2% per year during 2000-2010, resulting in the largest urban area and the second largest urban population of the United States.61 As Texas’s population continues to grow, its urban area is likely to continue to expand and become denser, and, in combination with the projected increase in intense precipitation, the effects of urbanization would result in an increase in precipitation runoff and urban flooding. Such a trend is supported by results from hydrological model simulations.62 Assuming that the flooding trends in small, rapidly- responding urban basins are driven climatologically by rainfall intensity, the change in frequency of extreme rainfall would translate directly to a change in the expected frequency of urban flooding: 30%-50% more in 2036 relative to climatological expectations for 1950-1999 and 10%-15% more relative to 2000-2018. 19 Number of examined stream gauges exhibiting no statistically significant trends, downward trends, and upwards trends in peak river flow, grouped by metropolitan area. (Figure 4 from Berg, 2018) 20 18 Closer examination of statistically significant gauge trends in a) Dallas-Fort Worth-Arlington and b) Houston-The Woodlands-Sugar Land areas (Figure 5 from Berg, 2018) air. Freezing rain is most common in the northeastern part of the state. The climate data record for freezing rain observations is quite sparse compared to snow and rain. Freezing rain trends in Texas during the last half of the 20th century were mixed.67 Because freezing rain is strongly dependent on temperature conditions, one might expect a decrease in the threat of freezing rain over time. However, the weather patterns that produce freezing rain are rather specific, and changes in the frequency of those weather patterns could easily amplify or neutralize any temperature-driven trend. So the expectation of a decline in freezing rain frequency is tentative, and the magnitude of such a decline is as yet unknown. WINTER PRECIPITATION Winter precipitation is rare enough in Texas to be automatically disruptive in most areas. Snow and sleet are most common across northern Texas, the Panhandle, west-central Texas, and far west Texas.63 However, snow has been reported on occasion in almost every county in Texas. In the southern part of the state and in coastal regions, snow is rare, but nonetheless, large accumulations of snow are possible. Notable events include 1895 from Galveston to Beaumont (nearly two feet of snowfall accumulation)64, 1985 in San Antonio (over a foot), and 2004 in Victoria (nearly a foot).65 Given these rare events, it is perhaps not totally unbelievable that the one-day snowfall record for Texas is held not by a Panhandle city but by Hillsboro, north of Waco, with 26” in 1929. These sorts of extremely unusual but highly disruptive snow events have not been studied in a climate change context and they are too rare for any trends to be robustly detected in the historical record. Farther to the northwest, where snow is more common, there is no noticeable trend in the frequency of 2” or greater snowfalls from 1930 to 2007.66 Because the determining factor for snowfall in northwest Texas is typically air temperature, a reasonable expectation is that snowfall frequency and intensity will decrease in the future, somewhat reducing the snow hazard. Freezing rain is highly disruptive to vehicular travel and to electric power transmission. Freezing rain can occur when a warm layer of air aloft overlays subfreezing air near the surface. In Texas, this happens when a strong cold front stalls across Texas as an upper-air disturbance picks up warmer, humid air from over the Gulf of Mexico and carries it northward above the shallow cold Average annual snowfall at county index stations. 21 SEVERE THUNDERSTORMS There is no reliable, long-term record of severe thunderstorms or the severe weather they produce: tornadoes, hail, and strong winds. Reporting methods and magnitude scales have changed over time for tornadoes and hail events. Maps of the historic distribution of tornadoes, hail, and strong winds make it clear that no corner of the state is immune to severe thunderstorms.68 The dense clusters of observed events around major metropolitan areas such as Dallas- Fort Worth reflect the enhanced likelihood that a severe thunderstorm there will be witnessed or will cause damage. It is also possible to detect lines of severe weather reports along some major highways, such as Interstate 20 near Midland. The strong dependence of report location on population density gives an indication of how changes in severe thunderstorm reports over time are more strongly affected by changes in population than by changes in severe thunderstorms themselves.69 Not only is the climate data record for severe thunderstorms poor, severe thunderstorms are too small to be simulated directly by present-day climate models. Therefore, when assessing trends in severe thunderstorms, it is necessary to consider indirect indicators of severe thunderstorm frequency and intensity such as wind shear and convective instability, both of which favor severe storms. As temperatures increase, the amount of energy available to fuel these storms will increase as temperature and low-level moisture increase.70 Even though shear will likely decrease as the temperature gradient from the poles to the equator weakens, the increase in CAPE outweighs any decrease in low level shear.71 This results in an overall increase in the number of days capable of producing severe thunderstorms. Regarding the specific hazards of thunderstorms, lightning occurs most often during the months of May and June. Severe wind is most prevalent during the summer months from disorganized storm systems in the High Plains of Texas.72 The most robust trend in tornado activity is a tendency of more tornadoes in large outbreaks, but the factors apparently driving that trend are not projected to continue.73 Warmer temperatures are likely to lead to less hail overall, particular during the summer, but increases in available thunderstorm energy may lead to an increase of the risk of very large hail in springtime.74 22 21 23 Top: Locations and magnitudes of tornadoes in Texas, 1950-2017. Data from https://www.spc.noaa.gov/gis/svrgis. Bottom left: Locations and magnitudes of severe hail in Texas, 1950-2017. Data from https://www.spc.noaa.gov/gis/svrgis. Bottom right: Locations and magnitudes of potentially damaging thunderstorm winds in Texas, 1950-2017. Data from https://www.spc.noaa.gov/gis/svrgis. 22 23 HURRICANES AND COASTAL EROSION Sea level rise and storm intensity both affect coastal flooding and erosion. The change in ocean height relative to coastal lands, called relative sea level rise, is one of many factors affecting coastal erosion. Relative sea level rise is a combination of three factors: eustatic sea level rise, local variations in sea level rise, and relative land motion. Eustatic sea level rise is the change in global mean ocean height and is primarily the result of increasing temperatures that cause thermal expansion and melting glaciers and ice sheets. Local variations are produced by changes in wind patterns and ocean currents, and are minor for the Gulf of Mexico. Relative land motion in coastal Texas is dominated by coastal subsidence. Sabine Pass Galveston Pier 21 Freeport Rockport Port Mansfield Port Isabel Station ID 8770822 8771450 8772440 8774770 8778490 8779770 1958-2017 1904-2017 1954-2008 1937-2017 1963-2017 1944-2017 RSLR 5.80 mm/yr 6.49 mm/yr 4.43 mm/yr 5.58 mm/yr 3.05 mm/yr 3.98 mm/yr 95% C +/- 0.77 +/- 0.23 +/- 1.05 +/- 0.49 +/- 0.74 +/- 0.34 Relative sea-level rise (RSLR) and 95% confidence interval (95% C) at selected Texas tide gauges through 2017.75 Subsidence is a gradual lowering of land-surface elevation and is the result of the extraction of groundwater, oil, or gas or increasing sediment loading or infrastructure construction. As the coast of Texas slowly sinks, water potentially encroaches landward so quickly that it can exceed natural sediment accretion rates. In the state of Texas, the rate of subsidence ranges from less than 2 mm/yr to as much as 7 mm/yr.76 The variations are due to historical differences in oil, gas, or groundwater extraction and sediment loading, resulting in generally larger rates of subsidence in southeast Texas than in south Texas.77 The combination of local subsidence, eustatic sea level rise, and changes in sediment deposition and transport have produced a retreat of the Texas coastline along nearly the entire length of its barrier islands.78 In Galveston Bay and probably other bays and estuaries behind 24 Net rates of long-term change for the Texas Gulf shoreline between Sabine Pass and the Rio Grande, calculated from shoreline positions between the 1930’s and 2012. From Paine et al. (2014). the barrier islands, sediment deposition is not keeping up with relative sea level rise, leading to loss of coastal wetlands.79 In many areas there has been a decrease in extraction of groundwater and other resources as the problems associated with coastal subsidence have become clearer, resulting in a reduction in coastal subsidence. However, eustatic sea level has shown indications of acceleration,80 so on balance the near-term future rate of coastline retreat may be expected to be similar to historic rates. 25 Rising sea levels lead directly to increased risk of storm surge from hurricanes, as the storm surge is on top of an elevated baseline. Given typical return periods for storm surges along the Gulf Coast,81 a 1 meter relative sea level rise produces a doubling of storm surge risk, as a surge that would in the past have been expected have a 1% chance of occurring in any given year would in the future have a 2% chance of occurring in any given year. The places along the coast with the largest rates of relative sea level rise may have a doubled storm surge risk by 2050 relative to the risk at the beginning of the 20th century, purely due to the relative sea level rise itself. An additional element of enhanced risk is provided by an expected increase in the intensity of very strong hurricanes, despite an expected lack of increase, or even a decrease, in hurricane frequency overall.82 Different research studies have produced some conflicting results, and local trends over the western Gulf of Mexico will also be affected by changes in wind patterns for which global climate models have little predictive skill. At this point, the enhanced risk is difficult to quantify, but substantial scientific progress on this topic is likely as climate models become better able to simulate the observed spatial distribution, frequency, and intensity of hurricanes.83 25 WILDFIRES Fire depends on strong winds, low humidity, surface dryness, and factors affecting the amount of vegetation available to burn (known as fuel load). There is weak statistical evidence for a slight decline in the frequency of unusually strong winds speeds across the southern Great Plains.84 One future climate simulation predicts an increase in average wind speed across most of Texas in all seasons.85 On the whole, expectations for wind speed trends lack robustness. Low humidity and surface dryness are closely related. Low humidity refers to the extremely dry weather accompanying wildfire outbreaks across Texas, while surface dryness refers to the lack of moisture in dry, dormant, or otherwise combustible vegetation. Surface dryness is strongly influenced by weather conditions, but it is a consequence of desiccation of vegetation over an extended period of time rather than simply lack of humidity on a particular day. Vapor pressure deficit, an indicator of the ability of moisture to evaporate, is projected to increase as temperatures rise and carbon dioxide fertilization reduces transpiration, leading to both lower humidity and increased surface dryness.86 Overall, increased dryness should extend the wildfire season in places where the fire season is presently constrained by low levels of aridity, such as eastern Texas.87 Changes in fuel load involve two competing effects: increased aridity leading to reduced plant growth, and increased carbon dioxide leading to increased plant growth. Multiple papers predict wildfires in the Southwest (including Texas) will change differently than elsewhere in the country as fuel load gradually becomes the determining limit on fires.88 This transition in wildfire regime suggests that over the next twenty years, wildfire risk may increase more slowly in the Panhandle and Far West Texas than elsewhere as increased aridity reduces biomass. Meanwhile, the area of the state commonly affected by wildfires may expand eastward as fuels become drier faster in a warmer climate. 26 REFERENCES Al Mukaimi, M. E., T. M. Dellapenna, and J. R. Williams, 2018: Enhanced land subsidence in Galveston Bay, Texas: Interaction between sediment accumulation rates and relative sea level rise. Estuarine, Coastal and Shelf Science, 207, 183-193. Anderson, C. J., C. K. Wikle, Q. Zhou, and J. A. Royle, 2007: Population Influences on Tornado Reports in the United States. Weather and Forecasting, 22, 571-579. Ashley, S. T., and W. S. Ashley, 2008: Flood Fatalities in the United States. Journal of Applied Meteorology and Climatology, 47, 805-818. Berg, A., J. Sheffield, and P. C. D. Milly, 2017: Divergent surface and total soil moisture projections under global warming. Geophysical Research Letters, 44, 236-244. Camargo, S. J., and A. A. Wing, 2016: Tropical cyclones in climate models. Wiley Interdisciplinary Reviews: Climate Change, 7, 211-237. Capotondi, A., and M. A. Alexander, 2010: Relationship between Precipitation in the Great Plains of the United States and Global SSTs: Insights from the IPCC AR4 Models. Journal of Climate, 23, 2941-2958. Capotondi, A., and Coauthors, 2015: Understanding ENSO Diversity. Bulletin of the American Meteorological Society, 96, 921-938. Changnon, S. A., and T. R. Karl, 2003: Temporal and Spatial Variations of Freezing Rain in the Contiguous United States: 1948–2000. Journal of Applied Meteorology, 42, 1302-1315. Berg, A., and Coauthors, 2016: Land-atmosphere feedbacks amplify aridity increase over land under global warming. Nature Climate Change, 6, 869-+. Cleaveland, M. K., T. H. Votteler, D. K. Stahle, R. C. Casteel, and J. L. Banner, 2011: Extended Chronology of Drought in South Central, Southeastern and West Texas. Texas Water Journal, 2, 54-96. Berg, M. D., 2018: Peak flow trends highlight emerging urban flooding hotspots in Texas. Texas Water Journal, 9, 18-29. Cohen, J., K. Pfeiffer, and J. A. Francis, 2018: Warm Arctic episodes linked with increased frequency of extreme winter weather in the United States. Nature Communications, 9, 869. Berghuijs, W. R., R. A. Woods, C. J. Hutton, and M. Sivapalan, 2016: Dominant flood generating mechanisms across the United States. Geophysical Research Letters, 43, 4382-4390. Cohen, J., and Coauthors, 2014: Recent Arctic amplification and extreme mid-latitude weather. Nature Geoscience, 7, 627. Blackport, R., J. A. Screen, K. van der Wiel, and R. Bintanja, 2019: Minimal influence of reduced Arctic sea ice on coincident cold winters in mid-latitudes. Nature Climate Change, 9, 697-704. Bomar, G. W., 2017: Weather in Texas: The Essential Handbook. Third Edition ed. University of Texas Press, 290 pp. Bright, R. M., E. Davin, T. O’Halloran, J. Pongratz, K. G. Zhao, and A. Cescatti, 2017: Local temperature response to land cover and management change driven by non-radiative processes. Nature Climate Change, 7, 296-+. Brimelow, J. C., W. R. Burrows, and J. M. Hanesiak, 2017: The changing hail threat over North America in response to anthropogenic climate change. Nature Climate Change, 7, 516. Brooks, H. E., 2013: Severe thunderstorms and climate change. Atmospheric Research, 123, 129-138. Bukovsky, M. S., R. R. McCrary, A. Seth, and L. O. Mearns, 2017: A Mechanistically Credible, Poleward Shift in Warm- Season Precipitation Projected for the U.S. Southern Great Plains? Journal of Climate, 30, 8275-8298. Collins, M., and Coauthors, 2013: Long-term Climate Change: Projections, Commitments and Irreversibility. Climate Change 2013: The Physical Science Basis. Contribution of Working Group I to the Fifth Assessment Report of the Intergovernmental Panel on Climate Change, T. F. Stocker, and Coauthors, Eds., Cambridge University Press. Cook, B. I., T. R. Ault, and J. E. Smerdon, 2015: Unprecedented 21st century drought risk in the American Southwest and Central Plains. Science Advances. Dai, A., T. Zhao, and J. Chen, 2018: Climate Change and Drought: a Precipitation and Evaporation Perspective. Current Climate Change Reports, 4, 301-312. Dangendorf, S., C. Hay, F. M. Calafat, M. Marcos, C. G. Piecuch, K. Berk, and J. Jensen, 2019: Persistent acceleration in global sea-level rise since the 1960s. Nature Climate Change, 9, 705-710. Davis, R. A. J., 2011: Sea-Level Change in the Gulf of Mexico. Texas A&M University Press, 171 pp. Diffenbaugh, N. S., M. Scherer, and R. J. Trapp, 2013: Robust increases in severe thunderstorm environments in 27 response to greenhouse forcing. Proceedings of the National Academy of Sciences of the United States of America, 110, 16361-16366. Kunkel, K. E., and Coauthors, 2013: Monitoring and Understanding Trends in Extreme Storms: State of Knowledge. Bulletin of the American Meteorological Society, 94, 499-514. Easterling, D. R., and Coauthors, 2017: Precipitation change in the United States. Climate Science Special Report: Fourth National Climate Assessment, Volume I, D. J. Wuebbles, D. W. Fahey, K. A. Hibbard, D. J. Dokken, B. C. Stewart, and T. K. Maycock, Eds., U.S. Global Change Research Program, 207-230. Kurtzman, D., and B. R. Scanlon, 2007: El Nino-Southern Oscillation and Pacific Decadal Oscillation impacts on precipitation in the southern and central United States: Evaluation of spatial distribution and predictions. Water Resources Research, 43. Elsner, J. B., L. E. Michaels, K. N. Scheitlin, and I. J. Elsner, 2013: The Decreasing Population Bias in Tornado Reports across the Central Plains. Weather, Climate, and Society, 5, 221-232. Kushnir, Y., R. Seager, M. Ting, N. Naik, and J. Nakamura, 2010: Mechanisms of Tropical Atlantic SST Influence on North American Precipitation Variability*. Journal of Climate, 23, 5610-5628. Frederikse, T., S. Jevrejeva, R. E. M. Riva, and S. Dangendorf, 2018: A Consistent Sea-Level Reconstruction and Its Budget on Basin and Global Scales over 1958-2014. Journal of Climate, 31, 1267-1280. Guyette, R. P., F. R. Thompson, J. Whittier, M. C. Stambaugh, and D. C. Dey, 2014: Future Fire Probability Modeling with Climate Change Data and Physical Chemistry. Forest Science, 60, 862-870. Huang, Y., S. Wu, and J. O. Kaplan, 2015: Sensitivity of global wildfire occurrences to various factors in the context of global change. Atmospheric Environment, 121, 86-92. Lanza, M., 2017: Space City Rewind: Houston’s Great Snow of 1895. Space City Weather. Letetrel, C., M. Karpytchev, M. N. Bouin, M. Marcos, A. SantamarÍa-Gómez, and G. Wöppelmann, 2015: Estimation of vertical land movement rates along the coasts of the Gulf of Mexico over the past decades. Continental Shelf Research, 111, 42-51. Liu, Y., S. L. Goodrick, and J. A. Stanturf, 2013: Future U.S. wildfire potential trends projected using a dynamically downscaled climate change scenario. Forest Ecology and Management, 294, 120-135. Janssen, E., D. J. Wuebbles, K. E. Kunkel, S. C. Olsen, and A. Goodman, 2014: Observational- and model-based trends and projections of extreme precipitation over the contiguous United States. Earths Future, 2, 99-113. Mahmood, R., and Coauthors, 2014: Land cover changes and their biogeophysical effects on climate. International Journal of Climatology, 34, 929-953. Kirtman, B., and Coauthors, 2013: Near-term Climate Change: Projections and Predictability. Climate Change 2013: The Physical Science Basis. Contribution of Working Group I to the Fifth Assessment Report of the Intergovernmental Panel on Climate Change, T. F. Stocker, and Coauthors, Eds., Cambridge University Press. Kloesel, K., and Coauthors, 2018: Southern Great Plains. Impacts, Risks, and Adaptation in the United States: Fourth National Climate Assessment, Volume II, D. R. Reidmiller, C. W. Avery, D. R. Easterling, K. E. Kunkel, K. L. M. Lewis, T. K. Maycock, and B. C. Stewart, Eds., U.S. Global Change Research Program, 987-1035. Kluver, D., and D. Leathers, 2015: Winter snowfall prediction in the United States using multiple discriminant analysis. International Journal of Climatology, 35, 2003-2018. Kossin, J. P., T. Hall, T. R. Knutson, K. E. Kunkel, R. J. Trapp, D. E. Waliser, and M. E. Wehner, 2017: Extreme Storms. Climate Science Special Report: Fourth National Climate Assessment, Volume I, D. J. Wuebbles, D. W. Fahey, K. A. Hibbard, D. J. Dokken, B. C. Stewart, and T. K. Maycock, Eds., U.S. Global Change Research Program, 257-276. Maloney, E. D., and Coauthors, 2014: North American Climate in CMIP5 Experiments: Part III: Assessment of Twenty-First-Century Projections. Journal of Climate, 27, 2230-2270. McCabe, G. J., M. A. Palecki, and J. L. Betancourt, 2004: Pacific and Atlantic Ocean influences on multidecadal drought frequency in the United States. Proceedings of the National Academy of Sciences of the United States of America, 101, 4136-4141. Meehl, G. A., J. M. Arblaster, and C. T. Y. Chung, 2015: Disappearance of the southeast US “warming hole” with the late 1990s transition of the Interdecadal Pacific Oscillation. Geophysical Research Letters, 42, 5564-5570. Milly, P. C. D., and K. A. Dunne, 2016: Potential evapotranspiration and continental drying. Nature Climate Change, 6, 946-+. Mishra, A. K., and V. P. Singh, 2010: Changes in extreme precipitation in Texas. J Geophys Res-Atmos, 115. Mishra, A. K., V. P. Singh, and M. Özger, 2011: Seasonal streamflow extremes in Texas river basins: Uncertainty, trends, and teleconnections. Journal of Geophysical Research, 116. 28 Morice, C. P., J. J. Kennedy, N. A. Rayner, and P. D. Jones, 2012: Quantifying uncertainties in global and regional temperature change using an ensemble of observational estimates: The HadCRUT4 data set. Journal of Geophysical Research: Atmospheres, 117. Mukherjee, S., A. Mishra, and K. E. Trenberth, 2018: Climate Change and Drought: a Perspective on Drought Indices. Current Climate Change Reports, 4, 145-163. Needham, H. F., B. D. Keim, D. Sathiaraj, and M. Shafer, 2012: Storm Surge Return Periods for the United States Gulf Coast. Advances in Hurricane Engineering, 715-740. Nerem, R. S., B. D. Beckley, J. T. Fasullo, B. D. Hamlington, D. Masters, and G. T. Mitchum, 2018: Climate-change- driven accelerated sea-level rise detected in the altimeter era. Proceedings of the National Academy of Sciences of the United States of America, 115, 2022-2025. Nigam, S., B. Guan, and A. Ruiz-Barradas, 2011: Key role of the Atlantic Multidecadal Oscillation in 20th century drought and wet periods over the Great Plains. Geophysical Research Letters, 38, n/a-n/a. Nikiel, C. A., and E. A. B. Eltahir, 2019: Summer Climate Change in the Midwest and Great Plains due to Agricultural Development during the Twentieth Century. Journal of Climate, 32, 5583-5599. Paciorek, C. J., D. A. Stone, and M. F. Wehner, 2018: Quantifying statistical uncertainty in the attribution of human influence on severe weather. Weather and Climate Extremes, 20, 69-80. Paine, J. G., T. L. Caudle, and J. R. Andrews, 2014: Shoreline Movement Along the Texas Gulf Coast, 1930’s to 2012, 62 pp. Paine, J. G., T. L. Caudle, and J. R. Andrews, 2017: Shoreline and Sand Storage Dynamics from Annual Airborne LIDAR Surveys, Texas Gulf Coast. Journal of Coastal Research, 33, 487-506. Pendergrass, A. G., R. Knutti, F. Lehner, C. Deser, and B. M. Sanderson, 2017: Precipitation variability increases in a warmer climate. Sci Rep, 7, 17966. Peng, S.-S., and Coauthors, 2014: Afforestation in China cools local land surface temperature. Proceedings of the National Academy of Sciences, 111, 2915-2919. Perica, S., S. Pavlovic, M. St. Laurent, C. Trypaluk, D. Unruh, and O. Wilhite, 2018: NOAA Atlas 14: Precipitation- Frequency Atlas of the United States, Volume 11 Version 2.0: Texas, 40 pp. Pfahl, S., P. A. O’Gorman, and E. M. Fischer, 2017: Understanding the regional pattern of projected future changes in extreme precipitation. Nature Climate Change, 7, 423. Pryor, S. C., and J. Ledolter, 2010: Addendum to “Wind speed trends over the contiguous United States”. Journal of Geophysical Research: Atmospheres, 115. Scheff, J., 2018: Drought Indices, Drought Impacts, CO2, and Warming: a Historical and Geologic Perspective. Current Climate Change Reports, 4, 202-209. Schmandt, J., G. R. North, and J. Clarkson, 2011: The Impact of Global Warming on Texas. Second ed. University of Texas Press, 318 pp. Schubert, S., and Coauthors, 2009: A U.S. CLIVAR Project to Assess and Compare the Responses of Global Climate Models to Drought-Related SST Forcing Patterns: Overview and Results. Journal of Climate, 22, 5251-5272. Seager, R., A. Hooks, A. P. Williams, B. Cook, J. Nakamura, and N. Henderson, 2015: Climatology, Variability, and Trends in the U.S. Vapor Pressure Deficit, an Important Fire-Related Meteorological Quantity. Journal of Applied Meteorology and Climatology, 54, 1121-1141. Smith, B. T., T. E. Castellanos, A. C. Winters, C. M. Mead, A. R. Dean, and R. L. Thompson, 2013: Measured Severe Convective Wind Climatology and Associated Convective Modes of Thunderstorms in the Contiguous United States, 2003–09. Weather and Forecasting, 28, 229-236. Stambaugh, M. C., R. P. Guyette, E. D. Stroh, M. A. Struckhoff, and J. B. Whittier, 2018: Future southcentral US wildfire probability due to climate change. Climatic Change, 147, 617-631. Swann, A. L. S., 2018: Plants and Drought in a Changing Climate. Current Climate Change Reports, 4, 192-201. Swann, A. L. S., F. M. Hoffman, C. D. Koven, and J. T. Randerson, 2016: Plant responses to increasing CO2 reduce estimates of climate impacts on drought severity. Proceedings of the National Academy of Sciences of the United States of America, 113, 10019-10024. Thomson, A. M., and Coauthors, 2011: RCP4.5: a pathway for stabilization of radiative forcing by 2100. Climatic Change, 109, 77-94. Tippett, M. K., C. Lepore, and J. E. Cohen, 2016: More tornadoes in the most extreme U.S. tornado outbreaks. Science, 354, 1419-1423. Trenberth, K. E., A. Dai, R. M. Rasmussen, and D. B. Parsons, 2003: The Changing Character of Precipitation. Bulletin of the American Meteorological Society, 84, 1205- 1218. TSHA, 2018: Texas Almanac 2018-2019. Texas State Historical Association, 752 pp. 29 USGCRP, 2017: Climate Science Special Report: Fourth National Climate Assessment, Volume I. U.S. Global Change Research Program, 470 pp. Van Klooster, S. L., and P. J. Roebber, 2009: Surface-Based Convective Potential in the Contiguous United States in a Business-as-Usual Future Climate. Journal of Climate, 22, 3317-3330. van Oldenborgh, G. J., E. Mitchell-Larson, G. A. Vecchi, H. de Vries, R. Vautard, and F. Otto, 2019: Cold waves are getting milder in the northern midlatitudes. Environmental Research Letters, 14, 114004. van Vuuren, D. P., and Coauthors, 2011: The representative concentration pathways: an overview. Climatic Change, 109, 5-31. Villarini, G., and J. A. Smith, 2013: Flooding in Texas: Examination of temporal changes and impacts of tropical cyclones. Journal of the American Water Resources Association, 49, 825-837. Climate Assessment, Volume I, D. J. Wuebbles, D. W. Fahey, K. A. Hibbard, D. J. Dokken, B. C. Stewart, and T. K. Maycock, Eds., U.S. Global Change Research Program, 185-206. Vose, R. S., and Coauthors, 2014: Improved historical temperature and precipitation time series for U.S. climate divisions. Journal of Applied Meteorology and Climatology, 53, 1232-1251. Wang, H., S. Schubert, M. Suarez, and R. Koster, 2010: The Physical Mechanisms by Which the Leading Patterns of SST Variability Impact U.S. Precipitation. Journal of Climate, 23, 1815-1836. Wood, A. W., L. R. Leung, V. Sridhar, and D. P. Lettenmaier, 2004: Hydrologic implications of dynamical and statistical approaches to downscaling climate model outputs. Climatic Change, 62, 189-216. Zhang, W., G. Villarini, G. A. Vecchi, and J. A. Smith, 2018: Urbanization exacerbated the rainfall and flooding caused by hurricane Harvey in Houston. Nature, 563, 384-388. Vogel, M. M., R. Orth, F. Cheruy, S. Hagemann, R. Lorenz, B. J. J. M. van den Hurk, and S. I. Seneviratne, 2017: Regional amplification of projected changes in extreme temperatures strongly controlled by soil moisture-temperature feedbacks. Geophysical Research Letters, 44, 1511-1519. Zhao, G., H. L. Gao, and L. Cuo, 2016: Effects of Urbanization and Climate Change on Peak Flows over the San Antonio River Basin, Texas. Journal of Hydrometeorology, 17, 2371-2389. Vose, R. S., D. R. Easterling, K. E. Kunkel, A. N. LeGrande, and M. F. Wehner, 2017: Temperature changes in the United States. Climate Science Special Report: Fourth National 30 ENDNOTES Endnotes i More general discussions of climate change and its impact on Texas may be found in: Schmandt, J., G. R. North, and J. Clarkson, 2011: The Impact of Global Warming on Texas. Second ed. University of Texas Press, 318 pp. USGCRP, 2017: Climate Science Special Report: Fourth National Climate Assessment, Volume I. U.S. Global Change Research Program, 470 pp. Kloesel, K., and Coauthors, 2018: Southern Great Plains. Impacts, Risks, and Adaptation in the United States: Fourth National Climate Assessment, Volume II, D. R. Reidmiller, C. W. Avery, D. R. Easterling, K. E. Kunkel, K. L. M. Lewis, T. K. Maycock, and B. C. Stewart, Eds., U.S. Global Change Research Program, 987-1035. ii Online data archive: ftp://ftp.ncdc.noaa.gov/pub/data/cirs/climdiv/ Vose, R. S., and Coauthors, 2014: Improved historical temperature and precipitation time series for U.S. climate divisions. Journal of Applied Meteorology and Climatology, 53, 1232-1251. iii Global temperatures are from the HadCRUT4 data set. All linear trends in this report are calculated using ordinary least squares. Morice, C. P., J. J. Kennedy, N. A. Rayner, and P. D. Jones, 2012: Quantifying uncertainties in global and regional temperature change using an ensemble of observational estimates: The HadCRUT4 data set. Journal of Geophysical Research: Atmospheres, 117. iv Meehl, G. A., J. M. Arblaster, and C. T. Y. Chung, 2015: Disappearance of the southeast US "warming hole" with the late 1990s transition of the Interdecadal Pacific Oscillation. Geophysical Research Letters, 42, 5564-5570, Vose, R. S., D. R. Easterling, K. E. Kunkel, A. N. LeGrande, and M. F. Wehner, 2017: Temperature changes in the United States. Climate Science Special Report: Fourth National Climate Assessment, Volume I, D. J. Wuebbles, D. W. Fahey, K. A. Hibbard, D. J. Dokken, B. C. Stewart, and T. K. Maycock, Eds., U.S. Global Change Research Program, 185-206. v The historical simulations and projections are based on CMIP5 multi-model ensemble output from the Downscaled CMIP3 and CMIP5 Climate and Hydrology Projections archive at https://gdo- dcp.ucllnl.org/downscaled_cmip_projections The first available ensemble member from the combined Historic and RCP 4.5 was used from each of the following models for temperature and from the combined Historic and RCP 8.5 for precipitation. A ^ means only RCP 4.5 simulations were available from a particular model, so it was not included in the precipitation ensemble. Modeling Center (or Group) Institute ID Model Name Commonwealth Scientific and Industrial Research Organization (CSIRO) and Bureau of Meteorology (BOM), Australia CSIRO-BOM Beijing Climate Center, China Meteorological Administration BCC Canadian Centre for Climate Modelling and Analysis National Center for Atmospheric Research CCCMA NCAR Community Earth System Model Contributors NSF-DOE-NCAR Centro Euro-Mediterraneo per I Cambiamenti Climatici CMCC CMCC-CM Centre National de Recherches Météorologiques / Centre Européen de Recherche et Formation Avancée en Calcul Scientifique Commonwealth Scientific and Industrial Research Organization in collaboration with Queensland Climate Change Centre of Excellence CNRM-CERFACS CNRM-CM5 CSIRO-QCCCE CSIRO-Mk3.6.0 ACCESS1.0 ACCESS1.3^ BCC-CSM1.1 BCC- CSM1.1(m) CanESM2 CCSM4 CESM1(BGC) CESM1(CAM5) 43 31 EC-EARTH consortium EC-EARTH EC-EARTH^ LASG, Institute of Atmospheric Physics, Chinese Academy of Sciences and CESS,Tsinghua University LASG-CESS FGOALS-g2 LASG, Institute of Atmospheric Physics, Chinese Academy of Sciences LASG-IAP FGOALS-s2^ The First Institute of Oceanography, SOA, China FIO FIO-ESM NOAA Geophysical Fluid Dynamics Laboratory NOAA GFDL NASA Goddard Institute for Space Studies NASA GISS National Institute of Meteorological Research/Korea Meteorological Administration NIMR/KMA HadGEM2-AO Met Office Hadley Centre (additional HadGEM2-ES realizations contributed by Instituto Nacional de Pesquisas Espaciais) MOHC (additional realizations by Institute for Numerical Mathematics Institut Pierre-Simon Laplace Japan Agency for Marine-Earth Science and Technology, Atmosphere and Ocean Research Institute (The University of Tokyo), and National Institute for Environmental Studies Atmosphere and Ocean Research Institute (The University of Tokyo), National Institute for Environmental Studies, and Japan Agency for Marine-Earth Science and Technology Max-Planck-Institut für Meteorologie (Max Planck Institute for Meteorology) Meteorological Research Institute Norwegian Climate Centre INPE) INM IPSL MIROC MPI-M MRI NCC MIROC MIROC5 GFDL-CM3 GFDL-ESM2G GFDL-ESM2M GISS-E2-H-CC^ GISS-E2-R GISS-E2-R-CC^ HadGEM2-CC HadGEM2-ES INM-CM4 IPSL-CM5A-LR^ IPSL-CM5A-MR IPSL-CM5B-LR MIROC-ESM MIROC-ESM- CHEM MPI-ESM-MR MPI-ESM-LR MRI-CGCM3 NorESM1-M NorESM1-ME^ The 1/8-degree BCSD (bias-corrected and statistically-downscaled) model output was downloaded as a geographically averaged ensemble mean over a latitude-longitude box centered on Texas: 27.5°-35.5°N, 94.5°- 104.0°W, inclusive. Wood, A. W., L. R. Leung, V. Sridhar, and D. P. Lettenmaier, 2004: Hydrologic implications of dynamical and statistical approaches to downscaling climate model outputs. Climatic Change, 62, 189-216. vi van Vuuren, D. P., and Coauthors, 2011: The representative concentration pathways: an overviewibid., 109, 5-31. Thomson, A. M., and Coauthorsibid.: RCP4.5: a pathway for stabilization of radiative forcing by 2100, 77-94. vii Kirtman, B., and Coauthors, 2013: Near-term Climate Change: Projections and Predictability. Climate Change 2013: The Physical Science Basis. Contribution of Working Group I to the Fifth Assessment Report of the Intergovernmental Panel on Climate Change, T. F. Stocker, and Coauthors, Eds., Cambridge University Press. viii 1900-1999 average: 64.6 °F. 2000-2018 average: 66.0 °F. 2036 projection: 67.6 °F. Warmest year on record: 2012 (67.8 °F). 32 44 ix To our knowledge, no studies have specifically investigated this issue for Texas, but the effect has been noted elsewhere. Mahmood, R., and Coauthors, 2014: Land cover changes and their biogeophysical effects on climate. International Journal of Climatology, 34, 929-953. Peng, S.-S., and Coauthors, 2014: Afforestation in China cools local land surface temperature. Proceedings of the National Academy of Sciences, 111, 2915-2919. Bright, R. M., E. Davin, T. O'Halloran, J. Pongratz, K. G. Zhao, and A. Cescatti, 2017: Local temperature response to land cover and management change driven by non-radiative processes. Nature Climate Change, 7, 296-+. x The stations are chosen for their geographical representativeness, data completeness, and lack of substantial changes in station location, and they are characterized as Urban (within metropolitan areas of at least 500,000 population), Semi-Urban (within cities of 10,000-100,000 or near cities of 100,000-500,000), and Rural (within cities of less than 10,000 or well outside of or upwind of larger cities). These stations, and which of four regions of the state in which they are found (Northwest, Northeast, South, and Coastal), are listed here: Urban: El Paso Intl Airport (NW); San Antonio Intl Airport (S); Dallas Love Field (NE); Houston Hobby Airport (C); McAllen (S) Semi-Urban: Hereford (NW); Huntsville (NE); College Station Easterwood Field (S); Harlingen (C); Corpus Christi Intl Airport (C); Brownfield (NW) Rural: Dalhart FAA Airport (NW); Luling (S); Midland Intl Airport (NW); Lufkin Angelina Co Airport (NE); Amarillo Intl Airport (NW); Victoria Regional Airport (C); Beeville 5 NE (R); Crosbyton (NW); Centerville (NE) In order to retain a sufficient number of homogeneous stations, the index data record only covers the period 1950- present. xi The county temperature stations are created as follows: using SC-ACIS web services, every daily maximum/minimum temperature observation is retrieved for a given county. Each year, starting in 1890, a station with no more than ten missing days is identified. If no such station exists, data for that year is marked as missing. If at least one such station exists, data from the station with the longest period-of-record is chosen. This data becomes the year’s data for that county. The process is repeated for each year through 2018. Compared to the index stations, the county stations are much less homogeneous. In particular, different stations would have taken observations at different times, which can skew average temperatures. Because of this, we only use the county stations to identify trends in monthly temperature extremes. Such block maxima are relatively insensitive to time-of-observation artifacts. xii Although El Paso is part of the NW region, its summertime temperatures are markedly higher than other stations within the region. So that El Paso's large number of 100 °F days would not have an outside influence on regional trends, triple-digit days at all other index stations in the NW region were averaged for the period 1961-1990, and a temperature threshold at El Paso was selected that is reached about as often as 100 °F was reached at other NW stations. That threshold turned out to be 103 °F, so urban triple-digit days in NW Texas are estimated by the number of days reaching 103 °F at El Paso. xiii Vogel, M. M., R. Orth, F. Cheruy, S. Hagemann, R. Lorenz, B. J. J. M. van den Hurk, and S. I. Seneviratne, 2017: Regional amplification of projected changes in extreme temperatures strongly controlled by soil moisture- temperature feedbacks. Geophysical Research Letters, 44, 1511-1519. xivEach year’s value consists of the average of four temperatures: the hottest temperature in June, the hottest temperature in July, the hottest temperature in August, and the hottest temperature in September. For example, if at the station being used for Travis County the highest temperature during June 2008 was 95, in July 2008 98, in August 2008 100, and in September 2008 97, the average hottest day in 2008 would be 97.5. The value for index stations is calculated in a similar manner, except that only June-August are used. xv USGCRP, 2017: Climate Science Special Report: Fourth National Climate Assessment, Volume I. U.S. Global Change Research Program, 470 pp. xvi Nikiel, C. A., and E. A. B. Eltahir, 2019: Summer Climate Change in the Midwest and Great Plains due to Agricultural Development during the Twentieth Century. Journal of Climate, 32, 5583-5599. xvii Calculated from county stations as the average of the lowest temperatures recorded during each of January, February, and December. xviii Cohen, J., and Coauthors, 2014: Recent Arctic amplification and extreme mid-latitude weather. Nature Geoscience, 7, 627. Cohen, J., K. Pfeiffer, and J. A. Francis, 2018: Warm Arctic episodes linked with increased frequency of extreme winter weather in the United States. Nature Communications, 9, 869. 45 33 34 Blackport, R., J. A. Screen, K. van der Wiel, and R. Bintanja, 2019: Minimal influence of reduced Arctic sea ice on coincident cold winters in mid-latitudes. Nature Climate Change, 9, 697-704. xix van Oldenborgh, G. J., E. Mitchell-Larson, G. A. Vecchi, H. de Vries, R. Vautard, and F. Otto, 2019: Cold waves are getting milder in the northern midlatitudes. Environmental Research Letters, 14, 114004. xx Those summers are 1990, 1998, 2009, and 2011. xxi Those winters are 1931 (27.1 °F), 1941 (25.1 °F), 1992 (24.7 °F), 1991 (24.3 °F), and 1907 (24.2 °F). xxii The county precipitation stations were created following the same method as the county temperature stations. xxiii Precipitation from single ensemble members from the Historical+RCP4.5 CMIP5 runs were averaged over the box 25°N-37.5°N, 95°W-105°W and downloaded from the KNMI Climate Explorer. The models were: ACCESS1- 0 ACCESS1-3 bcc-csm1-1 bcc-csm1-1-m BNU-ESM CanESM2 CCSM4 CESM1-BGC CESM1-CAM5 CMCC- CM CMCC-CMS CNRM-CM5 CSIRO-Mk3-6-0 EC-EARTH FGOALS-g2 FIO-ESM GFDL-CM3 GFDL-ESM2G GFDL-ESM2M GISS-E2-H GISS-E2-H GISS-E2-H GISS-E2-H-CC GISS-E2-R GISS-E2-R GISS-E2-R GISS-E2- R-CC HadGEM2-AO HadGEM2-CC HadGEM2-ES inmcm4 IPSL-CM5A-LR IPSL-CM5A-MR IPSL-CM5B-LR MIROC5 MIROC-ESM MIROC-ESM-CHEM MPI-ESM-LR MPI-ESM-MR MRI-CGCM3 NorESM1-M NorESM1-ME. xxiv Maloney, E. D., and Coauthors, 2014: North American Climate in CMIP5 Experiments: Part III: Assessment of Twenty-First-Century Projections. Journal of Climate, 27, 2230-2270. xxv McCabe, G. J., M. A. Palecki, and J. L. Betancourt, 2004: Pacific and Atlantic Ocean influences on multidecadal drought frequency in the United States. Proceedings of the National Academy of Sciences of the United States of America, 101, 4136-4141. Kurtzman, D., and B. R. Scanlon, 2007: El Nino-Southern Oscillation and Pacific Decadal Oscillation impacts on precipitation in the southern and central United States: Evaluation of spatial distribution and predictions. Water Resources Research, 43. Schubert, S., and Coauthors, 2009: A U.S. CLIVAR Project to Assess and Compare the Responses of Global Climate Models to Drought-Related SST Forcing Patterns: Overview and Results. Journal of Climate, 22, 5251- 5272. Kushnir, Y., R. Seager, M. Ting, N. Naik, and J. Nakamura, 2010: Mechanisms of Tropical Atlantic SST Influence on North American Precipitation Variability*ibid., 23, 5610-5628. Wang, H., S. Schubert, M. Suarez, and R. Kosteribid.: The Physical Mechanisms by Which the Leading Patterns of SST Variability Impact U.S. Precipitation, 1815-1836. Capotondi, A., and M. A. Alexanderibid.: Relationship between Precipitation in the Great Plains of the United States and Global SSTs: Insights from the IPCC AR4 Models, 2941-2958. Nigam, S., B. Guan, and A. Ruiz-Barradas, 2011: Key role of the Atlantic Multidecadal Oscillation in 20th century drought and wet periods over the Great Plains. Geophysical Research Letters, 38, n/a-n/a. xxvi Texas precipitation data is from the nClimDiv dataset of the National Centers for Environmental Information. The AMO and PDO were obtained from the KNMI Climate Explorer using ERSSTv5 sea surface temperatures and, in the case of the AMO, the AMO residual from a regression against global mean surface temperature. xxvii Bukovsky, M. S., R. R. McCrary, A. Seth, and L. O. Mearns, 2017: A Mechanistically Credible, Poleward Shift in Warm-Season Precipitation Projected for the U.S. Southern Great Plains? Journal of Climate, 30, 8275-8298. xxviii Easterling, D. R., and Coauthors, 2017: Precipitation change in the United States. Climate Science Special Report: Fourth National Climate Assessment, Volume I, D. J. Wuebbles, D. W. Fahey, K. A. Hibbard, D. J. Dokken, B. C. Stewart, and T. K. Maycock, Eds., U.S. Global Change Research Program, 207-230. Janssen, E., D. J. Wuebbles, K. E. Kunkel, S. C. Olsen, and A. Goodman, 2014: Observational- and model-based trends and projections of extreme precipitation over the contiguous United States. Earths Future, 2, 99-113. Kunkel, K. E., and Coauthors, 2013: Monitoring and Understanding Trends in Extreme Storms: State of Knowledge. Bulletin of the American Meteorological Society, 94, 499-514. Mishra, A. K., and V. P. Singh, 2010: Changes in extreme precipitation in Texas. J Geophys Res-Atmos, 115. xxix Easterling, D. R., and Coauthors, 2017: Precipitation change in the United States. Climate Science Special Report: Fourth National Climate Assessment, Volume I, D. J. Wuebbles, D. W. Fahey, K. A. Hibbard, D. J. Dokken, B. C. Stewart, and T. K. Maycock, Eds., U.S. Global Change Research Program, 207-230. xxx One year block maxima of 1-day precipitation totals were fit to a GEV distribution with linearly time-dependent location and scale parameters using the climetRemes Python library. The trend in frequency of extreme rainfall was calculated using the time-dependent PDF. Citation for software package: Paciorek, C. J., D. A. Stone, and M. F. Wehner, 2018: Quantifying statistical uncertainty in the attribution of human influence on severe weather. Weather and Climate Extremes, 20, 69-80. 46 35 xxxi Trenberth, K. E., A. Dai, R. M. Rasmussen, and D. B. Parsons, 2003: The Changing Character of Precipitation. Bulletin of the American Meteorological Society, 84, 1205-1218. xxxii Pfahl, S., P. A. O’Gorman, and E. M. Fischer, 2017: Understanding the regional pattern of projected future changes in extreme precipitation. Nature Climate Change, 7, 423. xxxiii Easterling, D. R., and Coauthors, 2017: Precipitation change in the United States. Climate Science Special Report: Fourth National Climate Assessment, Volume I, D. J. Wuebbles, D. W. Fahey, K. A. Hibbard, D. J. Dokken, B. C. Stewart, and T. K. Maycock, Eds., U.S. Global Change Research Program, 207-230. xxxiv Based on the 2035 global temperature projection by Kirtman et al. (0.4 °C increase by 2036 compared to 2010- 2018) and the ratio of Gulf of Mexico to global temperature increases by Collins et al. (75%-100%). Kirtman, B., and Coauthors, 2013: Near-term Climate Change: Projections and Predictability. Climate Change 2013: The Physical Science Basis. Contribution of Working Group I to the Fifth Assessment Report of the Intergovernmental Panel on Climate Change, T. F. Stocker, and Coauthors, Eds., Cambridge University Press. Capotondi, A., and Coauthors, 2015: Understanding ENSO Diversity. Bulletin of the American Meteorological Society, 96, 921-938, Collins, M., and Coauthors, 2013: Long-term Climate Change: Projections, Commitments and Irreversibility. Climate Change 2013: The Physical Science Basis. Contribution of Working Group I to the Fifth Assessment Report of the Intergovernmental Panel on Climate Change, T. F. Stocker, and Coauthors, Eds., Cambridge University Press. xxxv Based on the county precipitation station analysis, the odds of extreme (once per decade or so) one-day precipitation decrease by a factor of two for every 20% increase in precipitation amount. xxxvi Based on the updated NOAA Atlas 14 values for Texas, which are used for flood risk calculations. Perica, S., S. Pavlovic, M. St. Laurent, C. Trypaluk, D. Unruh, and O. Wilhite, 2018: NOAA Atlas 14: Precipitation- Frequency Atlas of the United States, Volume 11 Version 2.0: Texas, 40 pp. xxxvii Online data archive: ftp://ftp.ncdc.noaa.gov/pub/data/cirs/climdiv/ Vose, R. S., and Coauthors, 2014: Improved historical temperature and precipitation time series for U.S. climate divisions. Journal of Applied Meteorology and Climatology, 53, 1232-1251. xxxviii Data and analysis archive label: climdiv-sp09st-v1.0.0-20190204 and SP09 Online data archive: ftp://ftp.ncdc.noaa.gov/pub/data/cirs/climdiv/ Overall yearly drought severity is computed by summing the negative statewide 9-month SPI values for a given year and dividing by 12 to obtain an average annual drought intensity. xxxix The historic simulations and projections are based on the CMIP5 multi-model ensemble output, as with Footnote iv for temperature, with the following differences: Model simulations using the RCP8.5 scenario are extracted, to improve signal-to-noise ratio. Precipitation is summed for each water year, October-September, rather than each calendar year, in order to better reflect the potential for dryness during the growing season and so as to not divide cool-season precipitation variability associated with El Niño across two separate years. The water year precipitation is converted to anomalies by subtracting the centered 29-year mean for each simulation, with the 1950- 1978 mean used for calculating anomalies in 1950-1964. Interannual precipitation variance is then calculated using the same 29-year intervals, and variance time series are created from the overlapping 29-year periods. xl Pendergrass, A. G., R. Knutti, F. Lehner, C. Deser, and B. M. Sanderson, 2017: Precipitation variability increases in a warmer climate. Sci Rep, 7, 17966. xli Mukherjee, S., A. Mishra, and K. E. Trenberth, 2018: Climate Change and Drought: a Perspective on Drought Indices. Current Climate Change Reports, 4, 145-163. xlii Data and analysis archive label: climdiv-pdsist.v1.0-.0-20190204 Online data archive: ftp://ftp.ncdc.noaa.gov/pub/data/cirs/climdiv/ Vose, R. S., and Coauthors, 2014: Improved historical temperature and precipitation time series for U.S. climate divisions. Journal of Applied Meteorology and Climatology, 53, 1232-1251. xliii Milly, P. C. D., and K. A. Dunne, 2016: Potential evapotranspiration and continental drying. Nature Climate Change, 6, 946-+. xliv Swann, A. L. S., F. M. Hoffman, C. D. Koven, and J. T. Randerson, 2016: Plant responses to increasing CO2 reduce estimates of climate impacts on drought severity. Proceedings of the National Academy of Sciences of the United States of America, 113, 10019-10024. Swann, A. L. S., 2018: Plants and Drought in a Changing Climate. Current Climate Change Reports, 4, 192-201. Scheff, J.Ibid.: Drought Indices, Drought Impacts, CO2, and Warming: a Historical and Geologic Perspective, 202- 209. xlv Dai, A., T. Zhao, and J. Chenibid.: Climate Change and Drought: a Precipitation and Evaporation Perspective, 301-312. 47 36 Berg, A., and Coauthors, 2016: Land-atmosphere feedbacks amplify aridity increase over land under global warming. Nature Climate Change, 6, 869-+. xlvi Swann, A. L. S., 2018: Plants and Drought in a Changing Climate. Current Climate Change Reports, 4, 192-201. xlvii Cook, B. I., T. R. Ault, and J. E. Smerdon, 2015: Unprecedented 21st century drought risk in the American Southwest and Central Plains. Science Advances. xlviii Seager, R., A. Hooks, A. P. Williams, B. Cook, J. Nakamura, and N. Henderson, 2015: Climatology, Variability, and Trends in the U.S. Vapor Pressure Deficit, an Important Fire-Related Meteorological Quantity. Journal of Applied Meteorology and Climatology, 54, 1121-1141. xlix Basis for estimate: Given a statewide average 1 hPa increase in vapor pressure deficit from 1960-2013 and a climatological mean warm season vapor pressure deficit of 14 hPa (both from Seager et al. 2015), the fractional trend is about -1/14 in 50 years, or -1/7 in 100 years. Over the 26 or so years from 2000-2018 to 2036, a continuation of the trend would yield a change of about -1/28, or -4%. l Berg, A., J. Sheffield, and P. C. D. Milly, 2017: Divergent surface and total soil moisture projections under global warming. Geophysical Research Letters, 44, 236-244. li Pendergrass, A. G., R. Knutti, F. Lehner, C. Deser, and B. M. Sanderson, 2017: Precipitation variability increases in a warmer climate. Sci Rep, 7, 17966. lii Bukovsky, M. S., R. R. McCrary, A. Seth, and L. O. Mearns, 2017: A Mechanistically Credible, Poleward Shift in Warm-Season Precipitation Projected for the U.S. Southern Great Plains? Journal of Climate, 30, 8275-8298. liii Cleaveland, M. K., T. H. Votteler, D. K. Stahle, R. C. Casteel, and J. L. Banner, 2011: Extended Chronology of Drought in South Central, Southeastern and West Texas. Texas Water Journal, 2, 54-96. liv Ashley, S. T., and W. S. Ashley, 2008: Flood Fatalities in the United States. Journal of Applied Meteorology and Climatology, 47, 805-818. lv Berghuijs, W. R., R. A. Woods, C. J. Hutton, and M. Sivapalan, 2016: Dominant flood generating mechanisms across the United States. Geophysical Research Letters, 43, 4382-4390. lvi Villarini, G., and J. A. Smith, 2013: Flooding in Texas: Examination of temporal changes and impacts of tropical cyclones. Journal of the American Water Resources Association, 49, 825-837. lvii Mishra, A. K., V. P. Singh, and M. Özger, 2011: Seasonal streamflow extremes in Texas river basins: Uncertainty, trends, and teleconnections. Journal of Geophysical Research, 116. lviii Berg, M. D., 2018: Peak flow trends highlight emerging urban flooding hotspots in Texas. Texas Water Journal, 9, 18-29. lix Zhang, W., G. Villarini, G. A. Vecchi, and J. A. Smith, 2018: Urbanization exacerbated the rainfall and flooding caused by hurricane Harvey in Houston. Nature, 563, 384-388. Among our concerns with this study are the use of generic building heights rather than actual lidar-measured heights to represent Houston urbanization and a method of aggregating streamflow trends that gives undue influence to outliers. lx Berg, M. D., 2018: Peak flow trends highlight emerging urban flooding hotspots in Texas. Texas Water Journal, 9, 18-29, Villarini, G., and J. A. Smith, 2013: Flooding in Texas: Examination of temporal changes and impacts of tropical cyclones. Journal of the American Water Resources Association, 49, 825-837. lxi Data accessed from U.S. Census Bureau, https://www.census.gov/geo/reference/ua/urban-rural-2010.html, 18 February 2019. lxii Zhao, G., H. L. Gao, and L. Cuo, 2016: Effects of Urbanization and Climate Change on Peak Flows over the San Antonio River Basin, Texas. Journal of Hydrometeorology, 17, 2371-2389. lxiii The county-scale historic snowfall analysis is generated from county-specific composite station data, as with the county-scale temperature and precipitation analyses discussed earlier in the report, except that the number of allowed missing data points per year is increased to 27 to allow for the lower reliability of reporting of null snowfall amounts. Also, for snowfall a year is defined as beginning on July 1 and ending on June 30. lxiv Lanza, M., 2017: Space City Rewind: Houston’s Great Snow of 1895. Space City Weather. [Accessed March 16, 2019] lxv Bomar, G. W., 2017: Weather in Texas: The Essential Handbook. Third Edition ed. University of Texas Press, 290 pp, TSHA, 2018: Texas Almanac 2018-2019. Texas State Historical Association, 752 pp. lxvi Kluver, D., and D. Leathers, 2015: Winter snowfall prediction in the United States using multiple discriminant analysis. International Journal of Climatology, 35, 2003-2018. lxvii Changnon, S. A., and T. R. Karl, 2003: Temporal and Spatial Variations of Freezing Rain in the Contiguous United States: 1948–2000. Journal of Applied Meteorology, 42, 1302-1315. 48 37 lxviii Data source: Storm Prediction Center, National Oceanic and Atmospheric Administration, https://www.spc.noaa.gov/gis/svrgis/ lxix For example, see: Anderson, C. J., C. K. Wikle, Q. Zhou, and J. A. Royle, 2007: Population Influences on Tornado Reports in the United States. Weather and Forecasting, 22, 571-579. Elsner, J. B., L. E. Michaels, K. N. Scheitlin, and I. J. Elsner, 2013: The Decreasing Population Bias in Tornado Reports across the Central Plains. Weather, Climate, and Society, 5, 221-232. lxx Van Klooster, S. L., and P. J. Roebber, 2009: Surface-Based Convective Potential in the Contiguous United States in a Business-as-Usual Future Climate. Journal of Climate, 22, 3317-3330. lxxi Brooks, H. E., 2013: Severe thunderstorms and climate change. Atmospheric Research, 123, 129-138, Diffenbaugh, N. S., M. Scherer, and R. J. Trapp, 2013: Robust increases in severe thunderstorm environments in response to greenhouse forcing. Proceedings of the National Academy of Sciences of the United States of America, 110, 16361-16366. lxxii Smith, B. T., T. E. Castellanos, A. C. Winters, C. M. Mead, A. R. Dean, and R. L. Thompson, 2013: Measured Severe Convective Wind Climatology and Associated Convective Modes of Thunderstorms in the Contiguous United States, 2003–09. Weather and Forecasting, 28, 229-236. lxxiii Tippett, M. K., C. Lepore, and J. E. Cohen, 2016: More tornadoes in the most extreme U.S. tornado outbreaks. Science, 354, 1419-1423. lxxiv Brimelow, J. C., W. R. Burrows, and J. M. Hanesiak, 2017: The changing hail threat over North America in response to anthropogenic climate change. Nature Climate Change, 7, 516. lxxv Tide gauge data is from the National Oceanic and Atmospheric Administration, https://tidesandcurrents.noaa.gov/sltrends/ lxxvi Letetrel, C., M. Karpytchev, M. N. Bouin, M. Marcos, A. SantamarÍa-Gómez, and G. Wöppelmann, 2015: Estimation of vertical land movement rates along the coasts of the Gulf of Mexico over the past decades. Continental Shelf Research, 111, 42-51. lxxvii Davis, R. A. J., 2011: Sea-Level Change in the Gulf of Mexico. Texas A&M University Press, 171 pp. lxxviii Paine, J. G., T. L. Caudle, and J. R. Andrews, 2017: Shoreline and Sand Storage Dynamics from Annual Airborne LIDAR Surveys, Texas Gulf Coast. Journal of Coastal Research, 33, 487-506. Paine, J. G., T. L. Caudle, and J. R. Andrews, 2014: Shoreline Movement Along the Texas Gulf Coast, 1930’s to 2012, 62 pp. lxxix Al Mukaimi, M. E., T. M. Dellapenna, and J. R. Williams, 2018: Enhanced land subsidence in Galveston Bay, Texas: Interaction between sediment accumulation rates and relative sea level rise. Estuarine, Coastal and Shelf Science, 207, 183-193. lxxx Frederikse, T., S. Jevrejeva, R. E. M. Riva, and S. Dangendorf, 2018: A Consistent Sea-Level Reconstruction and Its Budget on Basin and Global Scales over 1958-2014. Journal of Climate, 31, 1267-1280. Nerem, R. S., B. D. Beckley, J. T. Fasullo, B. D. Hamlington, D. Masters, and G. T. Mitchum, 2018: Climate- change-driven accelerated sea-level rise detected in the altimeter era. Proceedings of the National Academy of Sciences of the United States of America, 115, 2022-2025. Dangendorf, S., C. Hay, F. M. Calafat, M. Marcos, C. G. Piecuch, K. Berk, and J. Jensen, 2019: Persistent acceleration in global sea-level rise since the 1960s. Nature Climate Change, 9, 705-710. lxxxi Kossin, J. P., T. Hall, T. R. Knutson, K. E. Kunkel, R. J. Trapp, D. E. Waliser, and M. E. Wehner, 2017: Extreme Storms. Climate Science Special Report: Fourth National Climate Assessment, Volume I, D. J. Wuebbles, D. W. Fahey, K. A. Hibbard, D. J. Dokken, B. C. Stewart, and T. K. Maycock, Eds., U.S. Global Change Research Program, 257-276, Needham, H. F., B. D. Keim, D. Sathiaraj, and M. Shafer, 2012: Storm Surge Return Periods for the United States Gulf Coast. Advances in Hurricane Engineering, 715-740. lxxxii Kossin, J. P., T. Hall, T. R. Knutson, K. E. Kunkel, R. J. Trapp, D. E. Waliser, and M. E. Wehner, 2017: Extreme Storms. Climate Science Special Report: Fourth National Climate Assessment, Volume I, D. J. Wuebbles, D. W. Fahey, K. A. Hibbard, D. J. Dokken, B. C. Stewart, and T. K. Maycock, Eds., U.S. Global Change Research Program, 257-276. lxxxiii Camargo, S. J., and A. A. Wing, 2016: Tropical cyclones in climate models. Wiley Interdisciplinary Reviews: Climate Change, 7, 211-237. lxxxiv Pryor, S. C., and J. Ledolter, 2010: Addendum to “Wind speed trends over the contiguous United States”. Journal of Geophysical Research: Atmospheres, 115. lxxxv Liu, Y., S. L. Goodrick, and J. A. Stanturf, 2013: Future U.S. wildfire potential trends projected using a dynamically downscaled climate change scenario. Forest Ecology and Management, 294, 120-135. 49 38 lxxxvi Huang, Y., S. Wu, and J. O. Kaplan, 2015: Sensitivity of global wildfire occurrences to various factors in the context of global change. Atmospheric Environment, 121, 86-92. lxxxvii Liu, Y., S. L. Goodrick, and J. A. Stanturf, 2013: Future U.S. wildfire potential trends projected using a dynamically downscaled climate change scenario. Forest Ecology and Management, 294, 120-135. lxxxviii Guyette, R. P., F. R. Thompson, J. Whittier, M. C. Stambaugh, and D. C. Dey, 2014: Future Fire Probability Modeling with Climate Change Data and Physical Chemistry. Forest Science, 60, 862-870, Stambaugh, M. C., R. P. Guyette, E. D. Stroh, M. A. Struckhoff, and J. B. Whittier, 2018: Future southcentral US wildfire probability due to climate change. Climatic Change, 147, 617-631. 50 39 TEXAS A&M UNIVERSITY College of Geosciences 40