Item 2C - Street Impact Fee - WGI study — original pdf
Backup
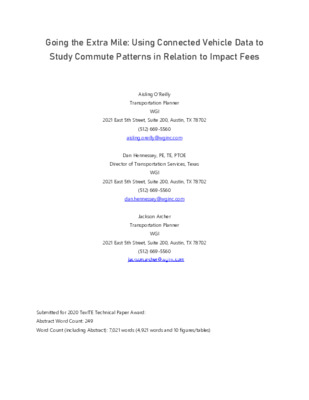
Going the Extra Mile: Using Connected Vehicle Data to Study Commute Patterns in Relation to Impact Fees Aisling O’Reilly Transportation Planner WGI (512) 669-5560 aisling.oreilly@wginc.com 2021 East 5th Street, Suite 200, Austin, TX 78702 Dan Hennessey, PE, TE, PTOE Director of Transportation Services, Texas WGI 2021 East 5th Street, Suite 200, Austin, TX 78702 (512) 669-5560 dan.hennessey@wginc.com Jackson Archer Transportation Planner WGI 2021 East 5th Street, Suite 200, Austin, TX 78702 (512) 669-5560 dan.hennessey@wginc.com Submitted for 2020 TexITE Technical Paper Award: Abstract Word Count: 249 Word Count (including Abstract): 7,021 words (4,921 words and 10 figures/tables) ABSTRACT The City of Austin is considering the adoption of a street impact fee program, which would change the manner in which developers take responsibility for paying for their portion of growth on the City’s transportation network. In developing this program, the City split Austin into seventeen zones by which to determine the maximum impact fee that can be charged per state law. Using a day’s worth of vehicle trip data from connected vehicle data company Wejo, each zone’s vehicle miles traveled (VMT) data were assessed to determine the average length of trips during the morning commute period, evening commute period, and overall daily trip lengths. The purpose for these analyses was to determine if certain areas of the city showed drastically different VMT patterns than others and what that impact might be on street infrastructure. We found that specific zones characterized by low amounts of employment and housing, typically on the periphery of the city, consistently generated the highest average VMT, whereas central zones had the lowest average VMT. This ability to evaluate real-world data on travel patterns allows the City of Austin and other jurisdictions to consider VMT as a criterion for evaluating development, including the imposition of street impact fees. When developers choose to build in high impact zones (high average VMT), it may be appropriate for them to pay a higher proportion towards growth mitigation than low impact zones (low average VMT), depending on the jurisdiction’s priorities and the type of growth they hope to incentivize. 2 BACKGROUND Growth within a community can place a significant burden on its infrastructure, depending on the planning processes that have preceded the growth. One way that a jurisdiction can make that growth pay for itself is via impact fees. The foundation of impact fees is the idea that developers should share a portion of the cost of local improvements rather than relying on existing citizens and taxpayers to pay entirely for mitigating growth. As the population of Texas grew rapidly in the 1980s, many local governments began to impart impact fees on new development. This authority was officially adopted in 1987, later becoming the basis of Chapter 395 of the Texas Local Government Code. The sorts of infrastructure improvements that can be accommodated via impact fees are water, wastewater, drainage, or roadway improvements; this paper focuses on roadway network impacts and the corresponding street impact fee. An impact fee may only be imposed to cover the cost of constructing capital improvements or facility expansions that have been included in a given area’s Capital Improvement Plan (CIP). It is important to note that these fees should only include the proportion of the cost that is attributable to growth; impact fees may not be used to maintain existing facilities or alleviate existing issues. Many cities in Texas have opted to establish street impact fee standards in their communities, including Fort Worth, College Station, and Arlington. The City of Austin has used impact fees to fund other improvements since the 1990s, but street impact fees have not yet been imparted on the development community. A technical analysis and determination of street impact fee zones was begun on behalf of the City in 2016 and a proposed policy is anticipated to be brought to Council sometime in 2020. While the methodology for the determination of zone sizes and calculations of maximum impact fees are specified in the Texas Local Government Code, the determination of the proportion of the maximum impact fee to be charged to developers is a policy decision determined by City leadership. 3 RESEARCH OBJECTIVE This document presents the results of research conducted to evaluate the street impact fee zones established by the City of Austin’s technical analysis (see Figure 1). This research had the following objectives: • Determine average VMT per trip originating in a given zone for 24 hours of trip data • Determine average VMT per trip originating in a given zone for the AM peak period (6:00 AM – 9:00AM) and PM peak period (4:00 PM – 7:00PM) • Determine average VMT per trip ending in a given zone for the AM peak period (6:00 AM – 9:00AM) and PM peak period (4:00 PM – 7:00PM) With this information, we can determine which street impact zones contribute the most vehicle miles per trip to the City’s transportation network; planning and policy decisions can be based on these additional calculations, which will be laid out throughout this paper. METHODS Data used for research purposes were provided by Wejo, a connected vehicle data collection and analysis company. Wejo provided WGI with one day’s worth of connected vehicle movement data from GMC vehicles 2014 and newer occurring in the Austin area. The data included the following attributes of which were useful in calculating daily VMT: Journey ID: a unique ID to represent each individual vehicle trip. Assigned to all data points collected between the origin and destination. Captured Time Stamp: string representing the timestamp collected for each data point in a journey. Latitude and Longitude: measured in degrees for each point, to six decimal places. Ignition Status: string representing vehicle ignition status. Three options: key on, midtrip, and key off. The data also included speed, the heading of the vehicle, and a unique identifier for each individual data point. As our research was broad and did not consist of analyzing individual trips, these attributes were not used for VMT calculations. A data point was collected on a given journey by a change in one of the attributes listed above; as such, each journey resulted in hundreds of individual data points, often caused by changes in latitude, longitude, and speed. 4 A total of 123,013 unique journeys were captured, consisting of over 28 million data points for connected vehicle journeys in Austin from Wednesday, May 8, 2019. Because each journey contained hundreds of data points, we filtered through the data based on ignition status, only keeping those journeys that contained “key on” and “key off” attributes. By doing so, we were left with the beginning and end points of each journey, but not each point on the route taken by the vehicle. We then discovered that 74,654 journeys in the dataset contained both “key on” and “key off” attributes, meaning that approximately 50,000 journeys did not have a definite beginning and end. This could mean that the origin or destination were not within the zone of collection defined by Wejo, that the driver drove through the study area without stopping, or that the beginning/end was not captured due to being at the end of the data collection time threshold. We were then able to join the origin and destination based on the journey ID, resulting in one line per journey, with origin and destination latitude, longitude, and timestamps. FIGURE 1: CITY OF AUSTIN STREET IMPACT FEE ZONES Source: City of Austin Street Impact Fee Study ArcGIS Online Map, 2019. 5 With locations and times of origin and destination data, we were tasked with determining which trips were entirely encapsulated within our study area. As our focus was on the street impact fee study zones as determined by the City, we wanted to replicate those zones in ArcGIS so we could filter the data by location. Because the street impact fee study zones are largely based on City of Austin boundaries, we were able to utilize the City’s open data portal and download an existing shapefile of city boundaries and use that as a starting point to digitize our study zones. The boundaries of study zones were most often defined at major roads or bodies of water, so manually clipping the existing boundary shapefile was feasible. The result was one shapefile composed of seventeen polygons, each corresponding to a street impact fee study zone. We were then able to add our origin and destination point data to the map, assigning each point that fell within a polygon to the corresponding zone it represented. Journeys with origins or destinations that were outside of the street impact fee polygons were removed from the dataset, as our focus was only trips that began and ended within the City of Austin. As many journeys started or ended outside our area of interest, this process left 49,905 journeys within our study area, indicating two-thirds of trips occurred entirely within City of Austin borders. The resulting dataset included a CSV file of: one line for each journey, represented by journey ID; origin and destination latitude and longitude; and origin and destination zone (represented by a letter from A to P, with DT representing downtown). The Open Street Maps package for Python was utilized to plot origin and destination data and model the most likely route a vehicle would take by finding the shortest path between the two points on the street network, much like Google Maps. The package, OSMnx, allows for users to access and reference entire street networks through Python. This process allowed us to understand the likely distances of each trip without having to review all of the intermediate points. Some journeys appeared to have been falsely triggered, made evident by the shortest distance between origin and destination equaling zero and confirmed by the timestamp differences being a matter of seconds. Alternatively, the journey could have been so short that the same origin and destination nodes were triggered (driving less than one block). To avoid muddling the data with low values that did not reflect true trip data, all journeys in which the shortest distance was equal to zero were removed from the dataset. There were 10,063 trips with a shortest distance of zero that were removed from the dataset. Additionally, if for whatever reason OSMnx was unable to find a path between the origin and destination on the existing street network, our code returned the value “No Path”. There were 301 trips with no found path that were removed from the dataset. After filtering the data, we had 39,541 usable trips to analyze within our study area. Each step is a conditional that must be met in order to be considered a usable journey. Figure 2 conveys the process of how journeys were evaluated for inclusion within the dataset. We were then able to use pivot tables in Excel to determine the total number of trips beginning and ending in each zone, the total VMT for each 6 zone, and the average VMT for trips beginning and ending in each zone. We compiled this data in ArcGIS, adding the attributes to our shapefile of street impact fee zones. FIGURE 2: JOURNEY DATA PROCESSING METHODOLOGY Provided Data: 123,013 journeys Key On/Key Off, In Study Area: 49,905 journeys (-73,108) Distance > 0: Distance ≠ No Path: 39,842 journeys 39,541 usable journeys (-10,063) (-301) 7 RESULTS Patterns for the entire day of data generally show that centralized zones have shorter trips on average than zones on the edge of town. The zone with the lowest average VMT was Zone I, just north of downtown, which encapsulates the University of Texas campus, Clarksville, Enfield, Tarrytown, North Campus, and Hyde Park. Trips originating in Zone I averaged 2.9 miles. The zone with the highest average VMT was Zone A, the farthest north zone, encompassing the Lakeline and Avery Ranch areas. Trips originating in Zone A averaged just over 8 miles. The zone with the highest total VMT was Zone L, the Bouldin Creek, Zilker, and Travis Heights neighborhoods, though it also had the highest number of total trips, resulting in having the second lowest average VMT of all seventeen zones. This is also one of the densest neighborhoods within the City of Austin, indicating that density decreases VMT in urban settings. A closer look at housing and employment data suggests that high average VMT is closely tied to the amount of available housing and employment facilities in a given area. The City of Austin Street Impact Fee Study in which the zones were determined included a comprehensive estimate of employment square footage and dwelling units by zone for the year 2017, which provides helpful context when analyzing commute patterns. A brief comparison of highest and lowest average VMT zones and approximate housing and employment estimates are included below in Table 1. TABLE 1: HIGHEST AND LOWEST VMT ZONES, DWELLING UNITS, EMPLOYMENT SQUARE FOOTAGE Highest Average VMT Zones Highest Average VMT (miles) Total Dwelling Units Per Zone Total Employment Square Footage Per Zone Lowest Average VMT Zones Lowest Average VMT (miles) Total Dwelling Units Per Zone Total Employment Square Footage Per Zone 10,256 4,630,000 41,490 20,416,000 8.01 7.17 6.50 3,910 1,356,000 9,282 7,131,000 I F J 2.90 3.18 3.22 28,421 21,225,000 37,764 20,413,000 Source: City of Austin Street Impact Fee Study, 2019. 8 A P K AM PEAK PERIOD Figure 4 shows the Street Impact Fee Zones by VMT specifically for the AM peak period (trips from 6:00 – 9:00 AM). There were 4,736 journeys that began during the AM peak period. Because AM peak period trips are typically for commuting purposes, trends in trip length can be telling in determining how far people are commuting to work or school each day. Originating trip length on the left in Figure 4 shows where people are coming from, whereas ending trip length on the left shows where people are commuting to. FIGURE 4: STREET IMPACT FEE ZONES BY VMT, AM PEAK PERIOD (6:00 AM TO 9:00 AM) Average VMT Beginning in Each Zone, AM Peak Average VMT Ending in Each Zone, AM Peak 9 LOWEST ORIGINATING ZONES – CONNECTED URBAN CORE The lowest originating zones for the AM peak period were Zone DT, Zone I, and Zone F. Both Zones I and F were also identified as lowest overall VMT generating zones for the full day of trip data. These zones are similar in that they are all centrally located, have high employment opportunities, and those who live in these zones likely stay within central Austin for work or school, leading to low originating trip lengths. Because of the high amount of employment opportunities, these zones likely have higher ending trip lengths due to employing commuters from outer zones. AM trips beginning and ending in the same zone (internal capture) rates are quite high ranging from 50-61.34 percent, suggesting that residents are employed within their own zone, resulting in low VMT. These zones also contain the lowest single-occupant mode share splits within the City, making the VMT per person-trip even lower compared to other portions of the City. HIGHEST ORIGINATING AND ENDING ZONES – DISCONNECTED SUBURBS In contrast are Zones A, H, and P, which both begin and end the AM peak period as the highest average VMT zones. Both Zones A and P were in the top three highest VMT generating zones for the full day of trip data, and all three zones remain highly ranked for VMT in all analyzed scenarios (see Table 2). These zones are characterized by the average trip length both beginning and ending strikingly high. Internal capture rates are low for these zones (8.33-38.71 percent), suggesting residents seek employment opportunities elsewhere. Additionally, these zones often have less connected roadway networks such that even traveling to a neighboring zone can produce high VMT; the average AM trip length from Zone A to adjacent Zone B was just under 7 miles. Unlike the connected suburbs, these zones do not have high VMT just from commuting to Zone DT; commuting to any other zone substantially raises average VMT due to the lack of connectivity of the roadway network. Given that these zones also have substantially less housing and employment than any other zones, their permanent presence as the highest generating VMT zones is expected. 10 ORIGINATING HIGH, ENDING LOW – CONNECTED SUBURBAN ZONES Many zones have minimal (within one mile) differences between their originating and ending AM trips, while other zones reveal drastic differences based on commute patterns. The most visually striking differences include Zone E, Zone M, and Zone N. Zones E, M, and N show similar commuting patterns to one another; they all have relatively high originating AM trip lengths (5.07 to 6.01 miles) and low ending AM trip lengths (3.32 to 3.65 miles). A closer inspection of Zones E, M, and N reveals that while a large proportion of trips begin and end internally (43.35 percent, 50 percent, and 41.16 percent, respectively), trips to Zone DT skew the mean trip length substantially higher. For example, trips beginning and ending within Zone E average 2 miles, but over 10 percent of AM trips beginning in Zone E end in Zone DT. The average VMT from Zone E to Zone DT is just under 13 miles. The trend of low length ending AM trips is because people do not generally commute to these regions; over 75 percent of trips that ended in Zone E began internally or the neighboring Zone B. Similarly, 81 percent of trips that ended in Zone M began internally or in neighboring Zone N. This pattern shows that while these zones produce relatively high average VMT, they have the employment opportunities to support residents, resulting in a low ending VMT. Despite being on the urban fringe, these zones have residential and employment infrastructure to operate as self-sufficient clusters. Without those commuting to Zone DT, these zones would likely operate with the same low originating VMT as those in the urban core, though their distance to and reliance on jobs downtown give them the characteristics of a standard suburb. PM PEAK PERIOD Figure 5 shows the Street Impact Fee Zones by VMT specifically for the PM peak period (trips from 4:00 – 7:00 PM). There were 7,652 journeys that began during the PM peak period. PM peak period trip data should, to an extent, appear to be the inverse of AM peak period, as commuters who work the standard hours will be captured under both peak periods and making the reverse trip of their morning commute. The connected suburban zones (Zones E, M, and N) show similar patterns in the PM peak period, though the VMT difference between origin and destination are much less pronounced. This could be caused by those employed in the zones working in industries that do not follow traditional workday hours, thus their trips home are not being captured. A more likely explanation is that PM peak period trips are muddled by other local evening trips; the PM peak period has more trips (7,652 journeys) than the AM peak period (4,736). The lowest VMT generating zones differed in the PM peak period, though generally speaking, the lower VMT generating zones had consistently moderate to high employment opportunities and housing. 11 As seen in Table 2, when analyzing the entire day’s dataset, the highest and lowest three VMT generating zones remain the same for originating or ending in a given zone. In other words, for the entire day’s data, there is no discernable difference between the length of trips that originate in a given zone or end in a given zone. A clear distinction appears when analyzing peak hour conditions. There is much greater variety between the lowest generating VMT zones during peak hours; the top three average VMT generators for all scenarios were different orders or the same four zones, whereas the lowest average VMT zones alternate between eight zones. FIGURE 5: STREET IMPACT FEE ZONES BY VMT, PM PEAK PERIOD (4:00 PM TO 7:00 PM) Average VMT Beginning in Each Zone, PM Peak Average VMT Ending in Each Zone, PM Peak 12 TABLE 2: HIGHEST AND LOWEST AVERAGE VMT GENERATING ZONES PER ANALYSIS SCENARIO Scenario Highest Average VMT Zones Highest Average VMT (miles) Lowest Lowest Average VMT Average VMT Zones (miles) Originating in Zone, All Day Data Ending in Zone, All Day Data Originating in Zone, AM Peak Period (6:00 – 9:00 AM) Ending in Zone, AM Peak Period (6:00 – 9:00 AM) Originating in Zone, PM Peak Period (4:00 – 7:00 PM) Ending in Zone, PM Peak Period (4:00 – 7:00 PM) A P K A P K A H P A H P P A H P A H 8.01 7.17 6.50 7.77 6.80 6.10 9.86 7.52 7.46 11.36 6.82 6.43 7.91 6.73 6.45 7.83 7.75 6.26 DT I F J I F J I F N J E N L F I DT L 2.90 3.18 3.22 2.84 3.13 3.20 2.31 2.55 3.20 3.32 3.62 3.62 3.02 3.09 3.16 2.88 2.91 3.07 13 APPLICATIONS To understand the implications of developing land uses in different zones, the anticipated average VMT generated by new developments can be studied. For the purposes of this analysis, the highest and lowest daily VMT generating zones were used to model hypothetical development locations; Zone A for the highest generating zone, and Zone I for the lowest generating zone. Specific locations for the hypothetical development have been included for determining transportation mode splits, which are available through census data at the block group scale. A 300-dwelling unit mid-rise apartment was chosen to model in multiple locations, selected based on areas of zones where similarly sized developments have been constructed or are under construction. The development in Zone A was modeled to be near Lakeline Boulevard and West Parmer Lane; the development in Zone I was modeled to be near North Lamar Boulevard and West 46th Street. Figure 6 includes a map of an approximation of these locations and a percentage of total person trips that are taken by motor vehicle, retrieved from the corresponding ACS Census Block Groups. The Institute of Transportation Engineers (ITE) Trip Generation Manual, 10th Edition, was used in tandem with mode split data to estimate the number of person and vehicle trips originating from the two developments. As they are the same size and land use, the same number of person trips are to be expected. Person trips were estimated using ITE’s average number of person trips per vehicle trip rates for multifamily mid-rise housing. ITE’s vehicle trip generation for Multifamily Housing Mid-Rise with 300 dwelling units were used to calculate approximate person vehicle trips before each block group’s mode split was applied. Mode split data were divided into “vehicle trips” and “other modes”. Carpool percentages were divided in half, with 50% added to vehicle trips and the remaining 50% added to other modes. Mode split data has been included as Appendix A. The resulting “vehicle trip” percentage for the two block groups were multiplied by the number of person trips for the developments to provide an estimate for how many people would be expected to be driving vs utilizing other modes of transportation. These values for AM and PM peak hour are reflected in row 2 of Table 4. These values were then multiplied by the average VMT for both zones, originating for the AM peak hour and ending for the PM peak hour. A detailed breakdown of the methodology has been included as Appendix B. Once the total estimated VMT per zone was calculated, it was divided by the estimated number of person trips for each development and each peak hour. The result is the average VMT per person trip, shown in row 3 of Table 4. Finally, total estimated VMT was calculated for each zone and peak hour. To make this estimate, the entering vehicle trips were assumed to have the average “end in zone” trip length, while the exiting vehicle trips were assumed to have the average “begin in zone” trip length for the given peak hour (see Appendix D). 14 FIGURE 6: EXAMPLE DEVELOPMENT LOCATIONS WITH MODE SPLIT PERCENTAGES Data retrieved from 2017 ACS Census Block Groups. 15 TABLE 4: VEHICLE TRIPS AND VMT FOR ZONE A AND ZONE I DEVELOPMENTS Estimated Person Trips Vehicle Trips VMT Per Person Trip AM 168 145 8.54 Zone A Development Lakeline Blvd / W Parmer Ln Zone I Development N Lamar Blvd / W 46th St PM 224 194 6.76 AM 168 111 1.69 PM 224 148 1.89 Total Estimated VMT 1,486.70 1,425.98 316.69 447.12 The results shown in Table 4 show a striking difference between a development in Zone A vs Zone I. While the person trips are the same, the mode split data provides a perspective on what proportion of trips are expected to reflect the average VMT rate calculated in the results section. The high number of vehicle trips and high average VMT rates for Zone A are made apparent when comparing total VMT to Zone I; Zone A is anticipated to generate over 4.5 times more vehicle miles than Zone I during the AM Peak hour, and just over 3 times more vehicle miles during the PM Peak Hour. This analysis provides a look into how a given development can impact the street infrastructure via the quantitative impact of vehicles miles traveled on the roadway network. Utilizing mode split data in concurrence with average VMT allows for tangible and measurable impacts as opposed to hypothesizing typical urban/suburban commute trends. This methodology could be utilized in the future; as developers choose to build in high impact zones (high average VMT), it may be appropriate for them to pay a higher proportion towards growth mitigation than low impact zones (low average VMT), depending on the jurisdiction’s priorities and the type of growth they hope to incentivize. 16 FOR FURTHER RESEARCH Although the various scenarios yielded different results for highest and lowest VMT generating zones, the general trends are clear; zones farther from the city center with less employment opportunities may fuel urban sprawl by requiring residents to commute. Though trip patterns were clear-cut, this study could be refined to yield more definitive results as technology allows. Firstly, this is not a comprehensive analysis of all vehicle travel, as only connected vehicle data from newer GMC vehicles were provided. This is only a small subset of all single-occupancy vehicle travel in Austin and trends may differ slightly when considering all travel; though because results generally showed long trips on the periphery and shorter trips centrally, zone rankings for highest and lowest VMT generators would likely remain similar. However, this assumption can only be verified with additional research and attempting to collect vehicle trip data at a broader scale. Similarly, this study only analyzed one day’s worth of data for the City of Austin. While we tried to ensure that the selected date of collection was more likely to reflect day-to-day travel patterns (collection midweek in May, with schools in session, outside of atypical Central Texas events), external factors we are unaware of could have impacted commute patterns. Additionally, because our criteria for a usable trip was so specific, some zones had significantly fewer journeys than others. While this is expected in areas with less employment and housing, it is difficult to know if our records reflect the true representative length of vehicle trips when there is a small sample; five of the seventeen zones had less than 500 daily trips beginning in the zone. Again, it is difficult with this initial study to determine if the discrepancy in the number of trips is proportional to population and what biases are potentially in play. This could be an area for additional study and future research. Another area for future consideration could be refining trip length methodology. We chose to find the shortest path between origin and destination for simplicity purposes, though this may not be the actual path taken by the driver. In some ways, this methodology is useful, as we can be confident that we are not overestimating VMT; if anything, the calculated averages are lower than the “true” values. However, for a more comprehensive understanding of the true trip lengths taken, methodology should be refined in future studies. 17 CONCLUSION transportation network. The City of Austin is considering the potential adoption of a street impact fee program. Such a program would change the manner in which developers take responsibility for paying for their portion of growth on the City’s Developments in certain areas do in fact put more strain on the street infrastructure than others. We found that specific zones characterized by low amounts of employment and housing, typically on the periphery of the city, consistently generated the highest average VMT, whereas central zones with more housing and employment had the lowest average VMT. The difference between high average VMT zones and low average VMT zones was more than five miles, and certain zones showed average travel distances of more than 150 percent as compared to the distances in zones closer to the urban core, revealing striking discrepancies in commute patterns (not to mention drastic mode split differences in these areas of the City). This ability to evaluate real-world data on travel patterns allows the City of Austin and other jurisdictions to consider VMT as a criterion for evaluating development, including the imposition of street impact fees. When developers choose to build in high impact zones (high average VMT), it may be appropriate for them to pay a higher proportion towards growth mitigation than low impact zones (low average VMT), depending on the jurisdiction’s priorities and the type of growth they hope to incentivize. The rapid growth and mounting sprawl in the City of Austin and many other areas has been a challenge to planners and local leaders. Fighting gentrification, attempting to build support for multimodal infrastructure, and maintaining support of current residents is a difficult balancing act. Land use policy affects all transportation decisions and ensuring high VMT generating zones pay their fair share should encourage development closer to the urban core (and as a result, provide more support for transportation solutions in those areas). A change in financial incentives for new development could allow local leaders to honestly tell their constituents that their taxes are doing more with less. 18 APPENDIX A: CENSUS MODE SPLIT DATA USED Zone A, Census Tract 205.08 Single Occupancy Carpool Transit Motorcycle Telecommute 14.1 1.1 1.6 1.3 3.2 Walk Mode Split Zone I, Census Tract 2.03 5.5 15.8 6.8 0.08 Single Occupancy Carpool Transit Telecommute Other 3.2 Walk Mode Split As noted, 50% of carpool percentages were added to Single Occupancy Vehicle and Motorcycle rates to calculate total vehicle trips. Data retrieved from http://ruvenbrooks-web01.s3-website-us-east-1.amazonaws.com/. 78.7 Vehicle 62.6 Vehicle 90 80 70 60 50 40 30 20 10 0 70 60 50 40 30 20 10 0 19 APPENDIX B - DETAILED METHODOLOGY 20