Item 4. ATC Food Plan Research Coding - Natalie Poulos - May 2025 — original pdf
Backup
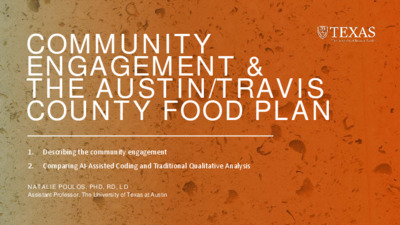
COMMUNITY ENGAGEMENT & THE AUSTIN/TRAVIS COUNTY FOOD PLAN 1. Describing the community engagement 2. Comparing AI-Assisted Coding and Traditional Qualitative Analysis NATALIE POULOS, PHD, RD, LD Assistant Professor, The University of Texas at Austin Why this study? • Qualitative researcher • Engaged in community food systems • Food Access Issue Area Working Group Research Aims: 1. Identify key food system concerns as described by community members 2. Compare AI-assisted qualitative coding and traditional content analysis Community Engagement Data • Public events included: – Community events – City-organized events – Online surveys • Two questions 1. What are your hopes & dreams for the Austin/Travis County food system? 2. What are your challenges & concerns with the Austin/Travis County food system? • 2,820 unique responses were documented – – 43 community events 27 zip codes Method & Analysis Comparison AI-Based Analysis Traditional Qualitative Analysis • • Public health practitioner based in local government GPT-4 + GPT for Sheets – Map comments to preidentified codes (issue areas) • • Define terms Set confidence – Asked AI to identify ‘themes’ (generative) based on community comments • • • “Analyze the following comments and provide me with the top themes related to food” Refined themes to 12 themes Expert review and discussion (3 themes added) – Map comments to ‘themes’ • GPT_MAP(Search_keys, Data, Confidence level, Top Results) • • • • Trained research team of at least two on reflexive content analysis Creation of codebook using preidentified content areas Testing reliability – Add in additional codes to highlight comments that did not fit original codes Manual coding – Issue areas + reflexive content codes Issue Area Code Comparison Food System Code Definition of Code1 Example of Key Terms2 • Direct comparison Markets & Retail of methods • Issue area codes Processing & Distribution How food is sold and purchased. What happens to food from where it is grown to when it reaches your plate, including how food is moved and processed. • Definitions and key terms based on City of Austin State of Food System Report (2022) Access & Consumption How we eat our food who struggles to get enough food, and what impact our consumption has on our health Food Production Where our food comes from, including everything from farming to ranching to backyard gardening Grocery Stores, Bodegas, Farmers Markets, Food Jobs, restaurants, Food Apps, Workers Rights, Selling Food Food Processing Facilities, Food Permits, Food Regulations, Food Aggregation, Food Distribution, Supply Chain, Food Delivery, Food Hubs Hunger Relief Programs, Hunger related policy, accessibility, disability access, access to transportation, nutrition, health, wellness, racial and ethnic disparities, homelessness, discrimination Farming, Agriculture, farms and environmental impact, green jobs, environmental sustainability, urban farms, land ownership, ranching and cattle, incentives for farming Post Consumption & Food Waste What happens to the parts of food we don’t eat and the impact of food waste on the environment. Food Recovery Apps, Composting, Trash pickup, Resource Management, Waste Prevention, Smart purchasing, Better purchasing practices, food rescue Key Notes from Comparison • AI-assisted use a “confidence” of 0.796 – Dropped 912 responses (+ 7 N/A = 919) • • “I hope butterflies will come” “Too many preservatives” • Content analysis was more discrete, less interpretive – 243 considered n/a or irrelevant – 1241 did not fit Food System Codes Needed new codes to highlight concerns or ideas outside of the 5 issue areas AI-Assisted: Themes Top themes identified: 1. Access to food and affordability 2. Agriculture and local food production 3. Nutrition, Health, and Wellness 4. Community Engagement Inductive Codes Access to Food & Affordability Advocacy & Civic Engagement Agriculture & Local Food Production Policy & Government Action Sustainability & Environment Resource Management & Waste Prevention Nutrition, Health, & Wellness Community Engagement Justice & Social Disparities Education, Innovation, & Resource Building Transportation & Infrastructure Diversity, Faith, & Cultural Inclusion Economic Issues & Labor Access to Land & Home Ownership Resilience & Emergency Response Challenges and Concerns Hopes and Dreams n 715 33 546 10 134 136 217 106 73 47 92 16 20 121 14 % 31% 1% 24% 0% 6% 6% 9% 5% 3% 2% 4% 1% 1% 5% 1% n 932 118 908 13 229 153 371 375 64 139 78 34 13 227 22 % 25% 3% 25% 0% 6% 4% 10% 10% 2% 4% 2% 1% 0% 6% 1% Content Analysis: Content Areas Top Content Areas: 1. Nutrition Education 2. Food Quality 3. Cost and Income Challenges and Concerns Hopes and Dreams Inductive Codes Food Justice Schools Nutrition Education Inclusive + Culturally Relevant Foods Food Policy Environmental Impact Transportation Food Quality Health Cost/Income Housing Community n 18 35 90 40 60 57 62 218 41 232 40 50 % 1.61% 3.13% 8.04% 3.57% 5.36% 5.09% 5.54% 19.48% 3.66% 20.73% 3.57% 4.47% n 41 57 111 70 50 45 40 202 17 79 37 86 % 4.55% 6.32% 12.31% 7.76% 5.54% 4.99% 4.43% 22.39% 1.88% 8.76% 4.10% 9.53% Key Take Aways • With data familiarity and human oversight, AI tools can be a useful for large amounts of data processing – Efficient, adaptable, affordable – Identified a similar issue area priorities (deductive) – Themes v. Content Areas were less comparable (inductive) • AI-based were interpretive & overlapping • Need to create definitions for themes before coding • Careful review of ‘confidence’ • Traditional content analysis is more discrete and can highlight important, but less common ideas – Time and resource intensive method AI Considerations for Next Time 1. Review codes matched to generative themes – Illogical mappings • 23rd ranked comment for Sustainability and Environment = “World Peace” 2. Review accuracy of Post-It Application – May explain so many irrelevant comments 3. Prompts are important. – Ensure all codes developed have definitions 4. Practitioners' equity lens is important – AI has been shown to misclassify or under-classify topics of racial justice and equity Thank you! For listening, and importantly to my collaborators Hannah Price, MS, Senior Research Coordinator, University of Texas at Austin, Lauren Bell, MPH, Doctoral Student, University of Texas at Austin Courtney Byrd-Williams, PhD, Assistant Professor, UT Health Houston, School of Public Health Sergio Torres-Peralta, Community Engagement Specialist, City of Austin Edwin Marty, Food Policy Manager, City of Austin Questions? Natalie.Poulos@austin.utexas.edu Academic Updates: This work has been submitted to the Journal of Prevention Science (under review). This work (Aim 2) and qualitative analysis (Aim 1) are being presented at the Society for Prevention Science Conference 2025.