Backup_Appendix D_Climate and Hydrology Analysis — original pdf
Backup
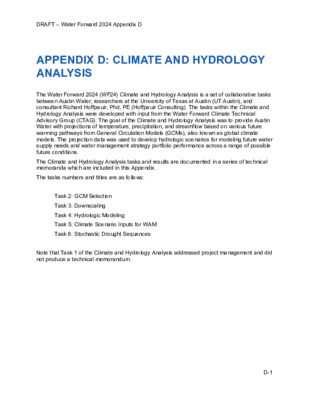
DRAFT – Water Forward 2024 Appendix D APPENDIX D: CLIMATE AND HYDROLOGY ANALYSIS The Water Forward 2024 (WF24) Climate and Hydrology Analysis is a set of collaborative tasks between Austin Water, researchers at the University of Texas at Austin (UT Austin), and consultant Richard Hoffpauir, Phd, PE (Hoffpauir Consulting). The tasks within the Climate and Hydrology Analysis were developed with input from the Water Forward Climate Technical Advisory Group (CTAG). The goal of the Climate and Hydrology Analysis was to provide Austin Water with projections of temperature, precipitation, and streamflow based on various future warming pathways from General Circulation Models (GCMs), also known as global climate models. The projection data was used to develop hydrologic scenarios for modeling future water supply needs and water management strategy portfolio performance across a range of possible future conditions. The Climate and Hydrology Analysis tasks and results are documented in a series of technical memoranda which are included in this Appendix. The tasks numbers and titles are as follows: Task 2: GCM Selection Task 3: Downscaling Task 4: Hydrologic Modeling Task 5: Climate Scenario Inputs for WAM Task 6: Stochastic Drought Sequences Note that Task 1 of the Climate and Hydrology Analysis addressed project management and did not produce a technical memorandum. D-1 DRAFT – Water Forward 2024 Appendix D Summary Task 2: Selection of General Circulation Models April 2022 Prepared by: The University of Texas at Austin This report summarizes the efforts as part of the UT-CoA project for evaluation of 35 General Circulations Models (GCMs) from the Coupled Model Intercomparison Project Phase 6 (CMIP6) in the Colorado River Basin. The goal is to find the most appropriate models by comparing the model results with observational datasets. Statistical analysis of three variables, including precipitation, temperature, and the number of dry days, provides quantitative assessment against the observational datasets. A methodology for scoring model performance is presented. Taylor diagrams are also used for visual comparison across multiple models. Finally, best- performing GCMs are suggested for use in the future tasks. D-2 DRAFT – Water Forward 2024 Appendix D List of Acronyms CMIP Coupled Model Intercomparison Project CPC Climate Predictions Center GCM General Circulation Model (or Global Climate Model) NDD Number of Dry Days P Precipitation R Correlation coefficient T Temperature UDEL University of Delaware WAM Water Availability Model WRCP World Climate Research Program D-3 DRAFT – Water Forward 2024 Appendix D Introduction GCMs are important tools for understanding Earth’s complex climate. They are used to project future climate conditions and help estimate future changes. For a robust climate assessment, multiple scenarios of future climate generated with varying assumed greenhouse gas emissions with multiple models need to be considered. The Coupled Model Intercomparison Project (CMIP) provides us with such an opportunity. CMIP, a standard experimental protocol for exploring the GCM output, provides a range of potential future climate scenarios (Taylor et al., 2012). This project was started in 1995 under the World Climate Research Program (WCRP). CMIP helps researchers and policymakers assess the impact of climate change on hydro- climatological variables such as precipitation, temperature, and streamflow using different climate projections (Meehl et al., 2014). CMIP Phase 6 (CMIP6) is the most recent phase and includes climate simulations for past, present, and future climate. Simulations ranging from 1850 to 2014 are called “historical simulations”. The historical simulations can be used to evaluate model performance against observed values of variables of interest. Future climate simulations are represented by different Shared Socioeconomic Pathways (SSPs) for different greenhouse gas (GHG) emission scenarios according to different climate policies. These future simulations begin in 2015 and consist of high and very high GHG emissions (SSP3-7.0 and SSP5-8.5), intermediate GHG emissions (SSP2-4.5) and very low and low GHG emissions (SSP1-1.9 and SSP1-2.6). Depending on the level of climate change mitigation and socio-economic assumptions, the trajectories of the emissions vary. For a detailed description of these SSPs, please refer to Gidden et al. (2019). One of the major challenges in using GCM outputs is the presence of large uncertainty among GCMs regarding future change. Uncertainty in climate projections arises from three distinct sources: internal variability, model uncertainty, and scenario uncertainty (Pfahl et al., 2017; O’Gorman, 2015; Pendergrass & Hartmann, 2014; Vano et al., 2014). Large uncertainty amongst climate models makes it difficult for stakeholders to determine which model projections are most suitable for their applications. To reduce this uncertainty, work performed for this Task screened GCMs based on their ability to simulate the observed climatology of Texas, with emphasis on the Colorado River Basin. Based on a literature review (Srivastava et al., 2020; Zhang et al., 2011) and correlations reported in the 2018 Water Forward Plan (Austin Water, 2018), we primarily focused on the monthly fields (indices) of temperature (T), precipitation (P), and the number of dry days (NDD), because they are significantly correlated with streamflow and have applications for drought analysis. NDD is defined as the daily precipitation that is lower than 0.01 inch (0.254 mm). Based on the models’ performance over the Colorado River Basin, we ranked the GCMs for identifying the most appropriate models. Methods Since each observational dataset and model simulation have different resolutions, we first regrid them to a common 1-degree horizontal grid, which is the same resolution as that of the Texas Water Development Board (TWDB) quadrangle evaporation and precipitation dataset (TWDB, 2020) which is used as input for the Texas Commission on Environmental Quality (TCEQ) Water Availability Model (WAM). Nearest-neighbor interpolation is used for precipitation, and bilinear interpolation is used for temperature. There are 27 quadrangles (grids) that cover or border the Colorado River Basin and are used in this report (Fig. 1 in Appendix) All months in the period from 1979 to 2014 are considered in the analysis. D-4 DRAFT – Water Forward 2024 Appendix D Sixth Coupled Model Intercomparison Project (CMIP6) CMIP6 (Eyring et al., 2016), the latest phase of CMIP, is used. Here we use the “historical simulations” that cover the period of instrumental measurements from 1850 to 2014. At the time of downloading data, 35 of the CMIP models have the required variables with ensemble sizes that range from 1 to 50 (Table 1). The available ensemble sizes are sometimes different from one variable to another, as noted in Table 1. We calculate monthly NDD from the daily precipitation of individual ensemble members before computing the ensemble average. It preserves the probability distribution of the daily precipitation. Models use different calendars (e.g., 360 days, no leap, standard). Thus, “percentage” instead of “days” is used in the following analysis. D-5 DRAFT – Water Forward 2024 Appendix D Table 1. List of the CMIP6 models used in this analysis Model Modeling Agency ACCESS-CM2 Commonwealth Scientific and Industrial Research Organisation (CSRIO), Australian Research Council Centre of Excellence for Climate System Science (ARCSS) ACCESS-ESM1-5 CSIRO BCC-CSM2-MR Beijing Climate Center, Meteorological Administration 250km 3, 3, 2 CAMS-CSM1-0 Chinese Academy of Meteorological Sciences 100km 3, 3, 1 CanESM5 Canadian Centre for Climate Modelling and Analysis 500km CESM2 National Center for Atmospheric Research (NCAR) 100km CMCC-CM2-SR5 Fondazione Centro Euro-Mediterraneo sui Cambiamenti Climatici (CMCC) CESM2-WACCM NCAR CMCC-ESM2 CMCC CNRM-CM6-1 Centre National de Recherches Meteorologiques (CNRM) 250km CNRM-CM6-1-HR CNRM CNRM-ESM2-1 CNRM EC-Earth3 EC-Earth-Consortium EC-Earth3-Veg EC-Earth-Consortium EC-Earth3-Veg-LR EC-Earth-Consortium FGOALS-g3 Chinese Academy of Sciences HadGEM3-GC31-LL Met Office Hadley Centre Met Office Hadley Centre HadGEM3-GC31- MM IITM-ESM GFDL-ESM4 NOAA/Geophysical Fluid Dynamics Laboratory (GFDL) 100km 3, 3, 1 Centre for Climate Change Research, Indian Institute of Tropical Meteorology Pune 250km 1, 1. 1 INM-CM4-8 Institute for Numerical Mathematics 100km 1, 1, 1 D-6 Nominal resolutio n Number of members* 100km 3, 3, 3 250km 10, 10, 40 50, 50, 50 11, 11, 10 100km 3, 3, 3 100km 1, 1, 1 100km 1, 1, 1 10, 10, 30 50km 1, 1, 1 250km 9, 9, 10 100km 5, 1, 5 100km 3, 3, 4 100km 3, 3, 3 250km 6, 6, 4 250km 5, 5, 5 100km 4, 4, 4 DRAFT – Water Forward 2024 Appendix D INM-CM5-0 Institute for Numerical Mathematics IPSL-CM6A-LR Institut Pierre Simon Laplace (IPSL) KACE-1-0-G National Institute of Meteorological Sciences/Korea Meteorological Administration KIOST-ESM Korea Institute of Ocean Science & Technology 250km 1, 1, 1 MPI-ESM1-2-HR Max Planck Institute for Meteorology 100km 10, 10, 7 NESM3 Nanjing University of Information Science and Technology 250km 5, 5, 4 MIROC6 JAMSTEC, AORI, NIES, R-CCS MIROC-ES2L JAMSTEC, AORI, NIES, R-CCS MPI-ESM1-2-LR Max Planck Institute for Meteorology MRI-ESM2-0 Meteorological Research Institute NorESM2-LM NorESM2-MM NCC NCC TaiESM1 AS-RCEC UKESM1-0-LL Met Office Hadley Centre 100km 250km 10, 10, 10 32, 32, 32 250km 3, 3, 3 250km 10, 10, 50 500km 10, 1, 1 250km 10, 10, 10 100km 5, 5, 7 250km 3, 3, 3 100km 3, 3, 3 100km 1, 1, 1 250km 19,19, 17 *Number of members that include temperature, precipitation, and NDD (daily precipitation), respectively Observational-based Datasets Two global gridded observational datasets are used: CPC Global Unified Gauge-Based Analysis of Daily Precipitation (Xie et al., 2007; Chen et al., 2008) and University of Delaware Global Gridded Air Temperature (Willmott and Matsuura, 2001). The gauge-based precipitation product is provided by the NOAA Climate Predictions Center (CPC) with quality control using 30,000 gauge stations, radar, and satellites. Monthly air temperature from UDEL is provided as local grid-point estimates and is widely used in model benchmarking. We regrid both datasets from 0.5 to the common 1-degree coordinates. Statistical Metrics We use several common statistical metrics to assess the model performance skill regarding (1) spatial distribution (long-term averaged maps: 27 grids), and (2) temporal distribution (annual or seasonal cycle: 12 months) of Colorado River Basin-averaged fields. All statistical metrics in this document are based on the ensemble-averaged fields of each model, instead of the average of skill scores from each member. The correlation coefficient (R) is used to compare the similarity between model fields (m) and observational fields (o). R is defined as: D-7 (1) DRAFT – Water Forward 2024 Appendix D 𝑁𝑁 𝑛𝑛=1 ∑ 1 𝑁𝑁 𝑅𝑅 = (𝑚𝑚𝑛𝑛 − 𝑚𝑚� ) and 𝜎𝜎𝑚𝑚𝜎𝜎𝑜𝑜 (𝑜𝑜𝑛𝑛 − 𝑜𝑜̅) are the standard deviations of m and o, and are the averaged values, and where respectively. Weights of grid cells are considered when calculating spatial standard deviation of the models can be compared to the standard deviation of the observations to show relative skill of the variance ( . The and 𝜎𝜎𝑚𝑚 ). 𝜎𝜎𝑜𝑜 𝑚𝑚� 𝑅𝑅 𝜎𝜎 𝑜𝑜̅ Evaluating Model Skill 𝜎𝜎� = 𝜎𝜎𝑚𝑚/𝜎𝜎𝑜𝑜 There are many methods and statistical metrics for evaluating models. Here we used a relatively straightforward and simple skill score, which is presented in Taylor, 2001 as follows: 4 4(1 + 𝑅𝑅) 2 1 where is the skill score that ranges from 0 (the least skillful) to 1 (the most skillful). ) 𝜎𝜎� maximum correlation, which can be set to a value according to the model fundamental limit; here we set to the maximum R obtained from each metrics and variable. 4 (1 + 𝑅𝑅0) (𝜎𝜎� + 𝑆𝑆 = 𝑅𝑅0 𝑆𝑆 is the (2) 𝑅𝑅0 We use an overall score ( ) to summarize all skill scores as follows: 𝑆𝑆𝑜𝑜𝑜𝑜𝑜𝑜𝑜𝑜𝑜𝑜𝑜𝑜𝑜𝑜 𝑆𝑆𝑜𝑜𝑜𝑜𝑜𝑜𝑜𝑜𝑜𝑜𝑜𝑜𝑜𝑜 = 2𝑆𝑆𝑠𝑠𝑠𝑠𝑜𝑜𝑠𝑠𝑠𝑠𝑜𝑜𝑜𝑜,𝑇𝑇 + 2𝑆𝑆𝑠𝑠𝑠𝑠𝑜𝑜𝑠𝑠𝑠𝑠𝑜𝑜𝑜𝑜,𝑃𝑃 + 2𝑆𝑆𝑠𝑠𝑠𝑠𝑜𝑜𝑠𝑠𝑠𝑠𝑜𝑜𝑜𝑜,𝑁𝑁𝑁𝑁𝑁𝑁 + 𝑆𝑆𝑠𝑠𝑜𝑜𝑚𝑚𝑠𝑠𝑜𝑜𝑜𝑜𝑜𝑜𝑜𝑜,𝑇𝑇 + 𝑆𝑆𝑠𝑠𝑜𝑜𝑚𝑚𝑠𝑠𝑜𝑜𝑜𝑜𝑜𝑜𝑜𝑜,𝑃𝑃 + 𝑆𝑆𝑠𝑠𝑜𝑜𝑚𝑚𝑠𝑠𝑜𝑜𝑜𝑜𝑜𝑜𝑜𝑜,𝑁𝑁𝑁𝑁𝑁𝑁 2 + 2 + 2 + 1 + 1 + 1 The weighted score is adapted from Collier et al. (2018). Theoretically, the skill scores cannot be expected to be 1 because of internal climate variations and model limitations. We decided to give the spatial skills more weight than temporal skills to emphasize their importance. (3) Taylor diagram Another way to summarize model performance is the Taylor Diagram. A Taylor diagram provides a visualized summary of the relative skills of multiple models. In Fig. 2-4 (Appendix), 35 models are represented by different marks. The distance between the observation point (a red dot on the x-axis) and each model is a measure of how realistically models reproduce the observations. Three statistics are shown: distance from origin shown in black dashed contours), and the root-mean-square difference (RMSD shown in green contours). The statistics shown in the Taylor Diagram are related, which can be presented in the form of the law of cosines, (azimuthal angles shown in blue contours), 𝑅𝑅 𝜎𝜎 (radial = 𝜎𝜎𝑚𝑚 where lengths of the three sides of the triangle and ′2 − 2𝜎𝜎𝑚𝑚𝜎𝜎𝑜𝑜𝑅𝑅 + 𝜎𝜎𝑜𝑜 is the centered RMSD. In a geometric relationship, is the angle opposite of 𝑅𝑅𝑅𝑅𝑆𝑆𝑅𝑅 𝑅𝑅𝑅𝑅𝑆𝑆𝑅𝑅 𝑅𝑅𝑅𝑅𝑆𝑆𝑅𝑅 2 ′ ′ , 2 𝑅𝑅 are the , and . 𝜎𝜎𝑜𝑜 𝜎𝜎𝑚𝑚 𝑅𝑅𝑅𝑅𝑆𝑆𝑅𝑅 ′ (4) D-8 DRAFT – Water Forward 2024 Appendix D Results Table 2 shows the skill score of the 35 CMIP6 models and their rankings. The rankings of the models are based on their ability to simulate the observed spatial patterns and annual cycles of monthly temperature, monthly precipitation, and monthly number of dry days over the Colorado River Basin. The ranking of a model varies based on the region of interest and evaluation criteria. Also, the rankings are based on model performance in the historical simulation/observed period, and model performance might vary in future scenarios. For these reasons, we are not declaring any model as the “Best Model,” as further detailed investigation on the model physics would be required for making such a conclusion. The ranking of all 35 models provides flexibility to select the desired number of models to provide the most useful data for the Water Forward update. After meetings with AW and the Water Forward 2024 climate technical advisory group, we plan to select the top 5 highest- ranked models and use one ensemble member from each model for historical simulation and a wide range of future scenarios, including SSP1-2.6 (low GHG emissions), SSP2-4.5 (medium GHG emissions), and SSP5-8.5 (high GHG emissions). The experiment designs of the SSP scenarios are presented in Meinshausen et al. (2020). SSP1-2.6 represents a possible more “sustainable” pathway, and its 2100 radiative forcing level is 2.6 W/m2. SSP2-4.5 is a “middle of the road” with 4.5 W/m2 radiative forcing by 2100. SSP5-8.5 is the one with the highest emission. We plan to use these three future projections to capture a wide range of potential emissions, and therefore, a wide range of possible warming levels. Table 3 shows the selected and unselected models. Here we select the 5 models based on three criteria: (1) rankings, (2) data availability for all scenarios and variables that will be used, and (3) no duplicated models from the same modeling center. As shown in Table 1, that CMIP6 models have ensemble sizes that range from 1 to 50 but because of time limitations, only the first available realization (single ensemble member) from each model will be used. Table 4 provides more details for the five selected models and the ensemble member being considered for each model. D-9 DRAFT – Water Forward 2024 Appendix D Table 2. The skills scores of 35 model performances over the Colorado River Basin Model Ranking CNRM-CM6-1-HR 𝑺𝑺𝒔𝒔𝒔𝒔𝒔𝒔𝒔𝒔𝒔𝒔𝒔𝒔𝒔𝒔,𝑻𝑻 0.90 𝑺𝑺𝒔𝒔𝒔𝒔𝒔𝒔𝒔𝒔𝒔𝒔𝒔𝒔𝒔𝒔,𝑷𝑷 0.94 𝑺𝑺𝒔𝒔𝒔𝒔𝒔𝒔𝒔𝒔𝒔𝒔𝒔𝒔𝒔𝒔,𝑵𝑵𝑵𝑵𝑵𝑵 0.97 𝑺𝑺𝒔𝒔𝒕𝒕𝒕𝒕𝒔𝒔𝒕𝒕𝒕𝒕𝒔𝒔𝒔𝒔,𝑻𝑻 0.98 𝑺𝑺𝒔𝒔𝒕𝒕𝒕𝒕𝒔𝒔𝒕𝒕𝒕𝒕𝒔𝒔𝒔𝒔,𝑷𝑷 0.63 𝑺𝑺𝒔𝒔𝒕𝒕𝒕𝒕𝒔𝒔𝒕𝒕𝒕𝒕𝒔𝒔𝒔𝒔,𝑵𝑵𝑵𝑵 0.55 𝑺𝑺𝒕𝒕𝒐𝒐𝒕𝒕𝒕𝒕𝒔𝒔𝒔𝒔𝒔𝒔 0.864 HadGEM3-GC31- MM 0.93 1.00 0.96 0.94 0.21 0.68 0.845 UKESM1-0-LL 0.92 0.88 0.70 0.94 0.75 0.68 0.818 HadGEM3-GC31-LL 0.91 0.91 0.70 0.95 0.54 0.75 0.809 CNRM-CM6-1 0.90 0.79 0.72 0.96 0.72 0.70 0.801 CNRM-ESM2-1 0.91 0.82 0.75 0.95 0.65 0.63 0.798 KACE-1-0-G 0.91 0.94 0.79 0.94 0.08 0.72 0.779 GFDL-ESM4 0.91 0.78 0.81 0.94 0.54 0.49 0.775 ACCESS-CM2 0.89 0.95 0.77 0.95 0.31 0.33 0.758 EC-Earth3 0.91 0.95 0.95 0.92 0.13 0.04 0.747 EC-Earth3-Veg 0.91 0.95 0.95 0.91 0.14 0.04 0.745 EC-Earth3-Veg-LR 0.91 0.87 0.96 0.91 0.24 0.05 0.745 INM-CM4-8 0.93 0.80 0.81 0.97 0.23 0.37 0.740 NESM3 0.96 0.82 0.42 0.93 0.54 0.78 0.738 INM-CM5-0 0.93 0.73 0.75 0.98 0.22 0.49 0.724 CanESM5 0.91 0.69 0.76 0.93 0.12 0.56 0.706 KIOST-ESM 0.90 0.55 0.46 0.98 0.76 0.36 0.657 BCC-CSM2-MR 0.88 0.56 0.57 0.91 0.02 0.94 0.656 CMCC-CM2-SR5 0.95 0.44 0.41 0.96 0.64 0.26 0.608 CESM2-WACCM 0.94 0.74 0.49 0.96 0.07 0.06 0.605 NorESM2-MM 0.94 0.79 0.48 0.95 0.03 0.02 0.601 TaiESM1 0.91 0.33 0.50 0.97 0.48 0.45 0.596 ACCESS-ESM1-5 0.87 0.76 0.37 0.98 0.33 0.00 0.592 CESM2 0.94 0.70 0.41 0.96 0.08 0.06 0.579 IPSL-CM6A-LR 0.90 0.22 0.11 0.96 0.96 0.65 0.557 1 2 3 4 5 6 7 8 9 10 11 12 13 14 15 16 17 18 19 20 21 22 23 24 25 D-10 DRAFT – Water Forward 2024 Appendix D 1 2 3 4 5 6 7 8 CMCC-ESM2 0.95 0.34 0.24 0.96 0.57 0.25 0.540 MRI-ESM2-0 0.93 0.40 0.10 0.96 0.58 0.44 0.537 MPI-ESM1-2-HR 0.92 0.58 0.34 0.91 0.01 0.15 0.528 FGOALS-g3 0.96 0.16 0.27 0.95 0.31 0.31 0.484 MIROC6 1.00 0.53 0.01 0.92 0.08 0.22 0.478 NorESM2-LM 0.95 0.61 0.02 0.95 0.04 0.03 0.467 MIROC-ES2L 0.99 0.23 0.01 0.91 0.10 0.34 0.423 MPI-ESM1-2-LR 0.94 0.19 0.07 0.93 0.05 0.07 0.385 IITM-ESM 0.90 0.31 0.01 0.98 0.01 0.00 0.383 CAMS-CSM1-0 0.90 0.23 0.03 0.91 0.02 0.00 0.360 26 27 28 29 30 31 32 33 34 35 Table 3. Selection processes Ranking Model Selected? Model Center Nominal Resolution CNRM-CM6-1-HR CNRM (Centre National de Recherches Meteorologiques) 50km no; no SSP245 daily data no; no SSP245 data no; duplicated modeling center (2) HadGEM3-GC31-MM Met Office Hadley Centre 100km UKESM1-0-LL yes Met Office Hadley Centre 250km HadGEM3-GC31-LL Met Office Hadley Centre 250km CNRM-CM6-1 yes CNRM-ESM2-1 CNRM CNRM no; duplicated modeling center (5) KACE-1-0-G yes National Institute of Meteorological Sciences/Korea Meteorological Administration GFDL-ESM4 NOAA-GFDL 100km no; no SSP126 data 250km 250km 250km D-11 DRAFT – Water Forward 2024 Appendix D 10 9 ACCESS-CM2 yes 100km CSIRO (Commonwealth Scientific and Industrial Research Organisation), ARCCSS (Australian Research Council Centre of Excellence for Climate System Science) EC-Earth3 yes EC-Earth-Consortium 100km D-12 DRAFT – Water Forward 2024 Appendix D Table 4. List of 5 models that will be used for following tasks Model Realization Atmosphere Model Ocean Model Land Surface Model UKESM1-0-LL r1i1p1f2 NEMO-HadGEM3-GO6.0 JULES-ES-1.0 MetUM-HadGEM3- GA7.1 CNRM-CM6-1 r1i1p1f2 Arpege 6.3 KACE-1-0-G r1i1p1f1 MetUM-HadGEM3- GA7.1 NEMO3.6 MOM4p1 Surfex 8.0c JULES-HadGEM3- GL7.1 ACCESS- CM2 r1i1p1f1 MetUM-HadGEM3- GA7.1 ACCESS-OM2 CABLE2.5 EC-Earth3 r1i1p1f1 IFS NEMO3.6 HTESSEL Conclusions Based on the model evaluation results described in this memo, we will use three future emission scenarios (SSPs) in the Water Forward modeling. Data of five climate models and ensemble members selected through the process in the memo under these three future emission scenarios will be analyzed, and communicated using end-of -century warming levels (see Table 5). These future emission scenarios will be combined with various other possible Water Forward future scenarios, which include multiple possibilities for future water demand and future regional supply, to generate a suite of overall Water Forwacollird modeling scenarios that will be used in conjunction with the WAM to evaluate water supply and demand management strategies. By employing this scenario-based modeling technique, we are modeling a wide range of possible futures, including possible climate outcomes, to evaluate which strategies and portfolios perform well against many potential futures, rather than attempting to predict which future scenario is most likely. Table 5. List of emission scenarios and models to be used in following tasks Climate Scenario # SSP Models Average End of Century Warming for CRB (°F) Ensemble members of models 1-2.6 2-4.5 5-8.5 4.4 6.6 11.4 ACCESS-CM2 r1i1p1f1 CNRM-CM6-1 r1i1p1f2 EC-Earth3 r1i1p1f1 KACE-1-0-G r1i1p1f1 UKESM1-0-LL r1i1p1f2 D-13 1 2 3 DRAFT – Water Forward 2024 Appendix D D-14 DRAFT – Water Forward 2024 Appendix D References Austin Water (2018). A Water Plan for the Next 100 year. https://austintexas.gov/sites/default/files/files/Water/WaterForward/Water_Forward_Plan_Re port_-_A_Water_Plan_for_the_Next_100_Years.pdf Chen, M., Shi, W., Xie, P., Silva, V. B. S., Kousky, V. E., Wayne Higgins, R., & Janowiak, J. E. (2008). Assessing objective techniques for gauge-based analyses of global daily precipitation. Journal of Geophysical Research: Atmospheres, 113(D4). https://doi.org/10.1029/2007JD009132 Eyring, V., Bony, S., Meehl, G. A., Senior, C. A., Stevens, B., Stouffer, R. J., & Taylor, K. E. (2016). Overview of the Coupled Model Intercomparison Project Phase 6 (CMIP6) experimental design and organization. Geoscientific Model Development, 9(5), 1937–1958. https://doi.org/10.5194/gmd-9-1937-2016 Gelca, R., Hayhoe, K., Scott-Fleming, I., Crow, C., Dawson, D., & Patiño, R. (2016). Climate– water quality relationships in Texas reservoirs. Hydrological Processes, 30(1), 12–29. https://doi.org/10.1002/hyp.10545 Gidden, M. J., Riahi, K., Smith, S. J., Fujimori, S., Luderer, G., Kriegler, E., van Vuuren, D. P., van den Berg, M., Feng, L., Klein, D., Calvin, K., Doelman, J. C., Frank, S., Fricko, O., Harmsen, M., Hasegawa, T., Havlik, P., Hilaire, J., Hoesly, R., Horing, J., Popp, A., Stehfest, E., and Takahashi, K.(2019): Global emissions pathways under different socioeconomic scenarios for use in CMIP6: a dataset of harmonized emissions trajectories through the end of the century, Geosci. Model Dev., 12, 1443– 1475, https://doi.org/10.5194/gmd-12-1443-2019. Meinshausen, M., Nicholls, Z. R. J., Lewis, J., Gidden, M. J., Vogel, E., Freund, M., Beyerle, U., Gessner, C., Nauels, A., Bauer, N., Canadell, J. G., Daniel, J. S., John, A., Krummel, P. B., Luderer, G., Meinshausen, N., Montzka, S. A., Rayner, P. J., Reimann, S., Smith, S. J., van den Berg, M., Velders, G. J. M., Vollmer, M. K., and Wang, R. H. J. (2020). The shared socio- economic pathway (SSP) greenhouse gas concentrations and their extensions to 2500, Geosci. Model Dev., 13, 3571–3605, https://doi.org/10.5194/gmd-13-3571-2020 Meehl, G. A., Moss, R., Taylor, K. E., Eyring, V., Stouffer, R. J., Bony, S., & Stevens, B. (2014). Climate Model Intercomparisons: Preparing for the Next Phase. Eos, Transactions American Geophysical Union, 95(9), 77–78. https://doi.org/10.1002/2014EO090001 O’Gorman, P. A. (2015). Precipitation Extremes Under Climate Change. Current Climate Change Reports, 1(2), 49–59. https://doi.org/10.1007/s40641-015-0009-3 Pendergrass, A. G., & Hartmann, D. L. (2014). Changes in the Distribution of Rain Frequency and Intensity in Response to Global Warming. Journal of Climate, 27(22), 8372–8383. https://doi.org/10.1175/JCLI-D-14-00183.1 Pfahl, S., O’Gorman, P. A., & Fischer, E. M. (2017). Understanding the regional pattern of projected future changes in extreme precipitation. Nature Climate Change, 7(6), 423–427. https://doi.org/10.1038/nclimate3287 Srivastava, A., Grotjahn, R., & Ullrich, P. A. (2020). Evaluation of historical CMIP6 model simulations of extreme precipitation over contiguous US regions. Weather and Climate Extremes, 29, 100268. https://doi.org/10.1016/j.wace.2020.100268 D-15 DRAFT – Water Forward 2024 Appendix D Taylor, K. E. (2001). Summarizing multiple aspects of model performance in a single diagram. Journal of Geophysical Research: Atmospheres, 106(D7), 7183–7192. https://doi.org/10.1029/2000JD900719 Taylor, K. E., Stouffer, R. J., & Meehl, G. A. (2012). An Overview of CMIP5 and the Experiment Design. Bulletin of the American Meteorological Society, 93(4), 485–498. https://doi.org/10.1175/BAMS-D-11-00094.1 TWDB (2020). The Texas Water Development Board Lake Evaporation and Precipitation dataset. https://waterdatafortexas.org/lake-evaporation-rainfall Vano, J. A., Udall, B., Cayan, D. R., Overpeck, J. T., Brekke, L. D., Das, T., Hartmann, H. C., Hidalgo, H. G., Hoerling, M., McCabe, G. J., Morino, K., Webb, R. S., Werner, K., & Lettenmaier, D. P. (2014). Understanding Uncertainties in Future Colorado River Streamflow. Bulletin of the American Meteorological Society, 95(1), 59–78. https://doi.org/10.1175/BAMS- D-12-00228.1 Willmott, C. J. and K. Matsuura. (2001). Terrestrial Air Temperature and Precipitation: Monthly and Annual Time Series. http://climate.geog.udel.edu/~climate/html_pages/README.ghcn_ts2.html. Xie, P., Chen, M., Yang, S., Yatagai, A., Hayasaka, T., Fukushima, Y., & Liu, C. (2007). A Gauge-Based Analysis of Daily Precipitation over East Asia. Journal of Hydrometeorology, 8(3), 607–626. https://doi.org/10.1175/JHM583.1 Zhang, X., Alexander, L., Hegerl, G. C., Jones, P., Tank, A. K., Peterson, T. C., Trewin, B., & Zwiers, F. W. (2011). Indices for monitoring changes in extremes based on daily temperature and precipitation data. Wiley Interdisciplinary Reviews: Climate Change, 2(6), 851–870. D-16 DRAFT – Water Forward 2024 Appendix D Task 2 Appendix Figure 1. 27 quadrangles (grids) that cover the Colorado River Basin D-17 DRAFT – Water Forward 2024 Appendix D (a) Spatial Pattern of Temperature (b) Temporal Pattern of Temperature Figure 2. The Taylor diagram for displaying statistics of temperature over the Colorado River Basin simulated by 35 CMIP6 models compared with the UDEL. (a) the spatial pattern across 27 grids, (b) the temporal pattern (annual cycle) across 12 months. (See “Methods” for details on Taylor Diagram) D-18 DRAFT – Water Forward 2024 Appendix D Figure 3. The Taylor diagram for displaying statistics of precipitation over the Colorado River Basin simulated by 35 CMIP6 models compared with the CPC. (a) the spatial pattern across 27 D-19 DRAFT – Water Forward 2024 Appendix D grids, (b) the temporal pattern (annual cycle) across 12 months. (See “Methods” for details on Taylor Diagram) D-20 DRAFT – Water Forward 2024 Appendix D Figure 4. The Taylor diagram for displaying statistics of number of dray days (NDD) over The Colorado River Basin simulated by 35 CMIP6 models compared with the CPC. (a) the spatial D-21 DRAFT – Water Forward 2024 Appendix D pattern across 27 grids, (b) the temporal pattern (annual cycle) across 12 months. (See “Methods” for details on Taylor Diagram) D-22 DRAFT – Water Forward 2024 Appendix D Figure 5. Modeled and observed temperature (1979—2014) D-23 DRAFT – Water Forward 2024 Appendix D Figure 6. Modeled and observed precipitation (1979—2014) D-24 DRAFT – Water Forward 2024 Appendix D Figure 7. Modeled and observed percentage of dry days with daily precipitation less than 0.01 inch (1979—2014) D-25 DRAFT – Water Forward 2024 Appendix D Figure 8. Modeled and observed annual temperature, precipitation and dryday cycle over the Colorado River Basin (1979—2014) D-26 DRAFT – Water Forward 2024 Appendix D Task 3: Downscale and Analyze GCM Data Prepared by: The University of Texas at Austin July 2022 Summary In Task 3, we analyze simulations of five general circulation models (GCMs) contributing to the sixth phase of the Coupled Model Intercomparison Project Phase 6 (CMIP6) under different future emission scenarios (SSP1-2.6, SSP2-4.5 and SSP5-8.5) and summarized the range of projected climate variables for three future time periods (2021-2040, 2041-2060 and 2081-2100) over the Colorado River Basin (CRB) and the Austin area. We also perform trend analysis of the variables for the period 2015 to 2100, using ordinary least squares linear regression. Both average and extreme weather variables are projected to change. Average annual temperature and annual number of days with maximum temperature above 100°F are expected to increase rapidly, with a decrease in annual number of days with minimum temperature below 32°F also projected. Under the higher emission scenario (SSP5-8.5), the annual maximum 5-day rainfall and the annual number of days with rainfall above 2” increases. Number of dry days with rainfall less than 0.01” increases and the average annual precipitation shows non-monotonic trend with a small change. This disparity in the changes in mean and extreme rainfall events implies a shift in frequency distribution of rainfall toward more heavy rainfall, as well as a decrease in rainfall frequency. Hence both heavy rainfall days and dry days are projected to increase. As we move towards the end of the 21st century, the differences among projections from different models and scenarios increases. The findings documented in this report are consistent with previous investigations over this region. The data described in this report will be used to generate climate-adjusted hydrology for the three emission scenarios to be used in the Water Forward 2024 water availability model. D-27 DRAFT – Water Forward 2024 Appendix D List of Acronyms CMIP Coupled Model Intercomparison Project CPC Climate Predictions Center CRB Colorado River Basin GCM General Circulation Model (or Global Climate Model) GHG Greenhouse Gas GWL Global Warming Level IPCC Intergovernmental Panel on Climate Change SSP Shared Socioeconomic Pathway UDEL University of Delaware WAM Water Availability Model D-28 DRAFT – Water Forward 2024 Appendix D Introduction Changes in the frequency and severity of extreme weather events will be one of the most impactful consequences of global warming. Studies suggest that continued greenhouse gas (GHG) emissions will lead to further warming, along with long-term changes in all components of the climate system. Texas, the largest state in the continental U.S. by area, is consistently prone to natural disasters. Historic observations suggest that there has been a long-term upward trend in precipitation in Texas in all seasons, averaging about 8.5% per century. The largest trends have been in central and eastern Texas, while parts of west Texas have seen a decrease. Additionally, there has been an upward trend in extreme precipitation at a variety of time scales. Annual air temperature anomalies in Texas show similar trend as global mean air temperature, although the variability is higher than the globally averaged temperatures (USGRP, 2017; Nielsen-Gammon et al., 2020). In the future, more frequent high-temperature extremes, decreased cold temperature extremes, longer dry spells, and more intense heavy rainfall events are expected (WF18; Nielsen-Gammon et al., 2021). For a robust climate assessment, multiple scenarios of future climate generated with varying assumed GHG emissions with multiple models are considered in the Coupled Model Intercomparison Project (CMIP) (Taylor et al. 2012; Meehl et al., 2014). CMIP helps researchers and policymakers gain a better understanding of the response of the climate system to a range of potential emission or concentration scenarios. In the most recent phase of CMIP, designated as CMIP6 (Eyring et al., 2016), future climate simulations are represented in different Shared Socioeconomic Pathways (SSPs) for different GHG emission scenarios according to different climate policies. These future simulations begin in 2015 and consist of high and very high GHG emissions (SSP3-7.0 and SSP5-8.5), intermediate GHG emissions (SSP2-4.5) and very low and low GHG emissions (SSP1-1.9 and SSP1-2.6). Depending on the level of climate change mitigation and socio-economic assumptions, the trajectories of the emissions vary (Figure 1). The response of climate systems to these emission scenarios is expected to be different. D-29 DRAFT – Water Forward 2024 Appendix D Figure 2: Future emission of Carbon Dioxide (CO2), the main contributor of greenhouse gas concentration according to different scenarios (image source: IPCC, 2021) One goal of the Water Forward 2024 update is to incorporate uncertainty into our future planning. To be able to test how water strategies perform under multiple possible climate futures, climate data for multiple emission scenarios (SSPs) were analyzed. In this study, we analyzed future projections of five GCMs under three emission scenarios (SSP1-2.6, SSP2-4.5 and SSP5-8.5). Downscaled data for each of the scenarios will be used in future tasks to generate climate-adjusted stream flows which will be used as inputs to the Texas Commission on Environmental Quality (TCEQ) Water Availability Model (WAM). Water strategies included in the ultimate Water Forward 2024 plan will be tested in the WAM against all climate scenarios examined here to measure their performance against multiple possible futures. Data and Methods Observational-based Datasets Daily precipitation, maximum temperature, and minimum temperature from the Oregon State University Parameter-Elevation Regressions on Independent Slopes Model (PRISM) dataset was used in this task. PRISM uses station data together with a digital elevation model (DEM) to provide gridded daily precipitation data on 4 km resolution for the United States (Daly et al., 2008). Sixth Coupled Model Intercomparison Project (CMIP6) In this task, daily precipitation, maximum temperature, and minimum temperature from five CMIP6 models (Table 1) under three GHG emission scenarios were analyzed. The five models in Table 1 were selected as part of Task 2. The first available realization (ensemble member) from each model was used. Historical simulations of these models from 1850 to 2014 and future simulations from 2015 to 2100 were used. Table 1. List of the CMIP6 models used in this analysis Model Modeling Agency Realization Calendar Original Resolution (lon × lat) CMIP6 SSP emission scenarios ACCESS- CM2 Commonwealth Scientific and Industrial Research Organisation (CSRIO) Australian Research Council Centre of Excellence for Climate System Science (ARCSS) CNRM- CM6-1 Centre National de Recherches Meteorologiques (CNRM) r1i1p1f1 Gregorian 1.88°× 1.25° EC-Earth3 EC-Earth-Consortium r1i1p1f1 Gregorian 0.70°×0.70° r1i1p1f2 Gregorian 1.41°×1.41° SSP1-2.6 SSP2-4.5 SSP5-8.5 National Institute of Meteorological Sciences/ Korea Meteorological Administration r1i1p1f1 360_day 1.88°× 1.25° Met Office Hadley Centre r1i1p1f2 360_day 1.88°×1.25° KACE-1-0-G UKESM1-0- LL D-30 DRAFT – Water Forward 2024 Appendix D Direct output evaporation data from the GCMs were not used in this analysis. We compared GCM evaporation data with TWDB lake evaporation data and found that the GCM values were very low as compared to the TWDB lake evaporation. This is because the evaporation data that we get from GCM is for the whole grid cell, not only over lakes and representation of land cover (vegetation, water bodies (lakes in this case)) are different in GCMs than in real world. Instead, we computed evaporation data (ET) over the TWDB quadrangles derived from the Hargreaves evapotranspiration equation (Hargreaves, 1982; Hargreaves et al., 1985) using GCM daily minimum and maximum temperature (Tmin and Tmax ): ET = k1(Tmax-Tmin)0.5(Tavg+k3)Ra + k4 (1) here k1, k2, k3, k4 are parameters for fitting model evaporation to TWDB lake evaporation, which effectively bias-corrects model evaporation to match observation. Ra is the extraterrestrial solar radiation at the latitude of observation site. The Hargreaves-derived evaporation data will be used as input to the WAM. Interpolation All datasets were re-gridded to a common 1-degree horizontal grid (latitude x longitude), which is the same grid spacing used for the Texas Water Development Board (TWDB) quadrangle evaporation and precipitation dataset (TWDB, 2020), which is used as input for the TCEQ WAM. Nearest neighbor interpolation was used for precipitation, and bilinear interpolation was used for temperature. Bilinear interpolation is commonly used in climate research fields when both sourced and targeted grids are rectilinear. For precipitation, which is discontinuous compared to other variables, bilinear interpolation is not appropriate because it smooths the maximum and increases the zero-precipitation events (Jones, 1999; Accadia et al., 2003). Therefore, nearest neighbor interpolation was applied to precipitation. There are 27 TWDB quadrangles (grids) that cover or border the Colorado River Basin (CRB; Fig. S1 in Appendix). Inverse distance weighted spatial interpolation is performed to estimate the variables for Austin from the nearest four quadrangles (709, 710, 809, 810). Bias correction: We bias correct the re-gridded GCM data using the PRISM daily observation from the period 1981-2014 at the center of each quadrangle. Daily minimum and maximum temperature are bias corrected using a linear transform: are the daily bias corrected temperatures and where temperatures. Values for observed mean ( GCM standard deviation and mean ( correct data for each GCM at each quadrangle per month. and 𝑜𝑜𝑜𝑜𝑜𝑜����� 𝑇𝑇𝑏𝑏𝑏𝑏 𝑇𝑇𝑏𝑏𝑏𝑏 = 𝑜𝑜𝑜𝑜𝑜𝑜����� + � 𝜎𝜎𝑜𝑜𝑏𝑏𝑠𝑠 𝜎𝜎𝑜𝑜𝑜𝑜𝑟𝑟� (𝑇𝑇𝑜𝑜𝑜𝑜𝑟𝑟 − 𝑟𝑟𝑟𝑟𝑟𝑟������ ) ), observed standard deviation ( are the daily uncorrected GCM ), uncorrected ) for the 1981-2014 period are used to bias 𝑇𝑇𝑜𝑜𝑜𝑜𝑟𝑟 𝜎𝜎𝑜𝑜𝑏𝑏𝑠𝑠 Daily precipitation is bias corrected using a power transform: 𝜎𝜎𝑜𝑜𝑜𝑜𝑟𝑟 𝑟𝑟𝑟𝑟𝑟𝑟������ (2) (3) 𝑏𝑏 are the daily bias corrected precipitation and 𝑃𝑃𝑏𝑏𝑏𝑏 = 𝑟𝑟(𝑃𝑃𝑜𝑜𝑜𝑜𝑟𝑟 − 𝑜𝑜) where precipitation. Values of a, b, and c are optimized for goodness of fit between over 1981-2014 for each GCM at each quadrangle per 2-month period (Jan-Feb, Mar-Apr, May- are the daily uncorrected GCM and PRISM 𝑃𝑃𝑜𝑜𝑜𝑜𝑟𝑟 𝑃𝑃𝑏𝑏𝑏𝑏 𝑃𝑃𝑏𝑏𝑏𝑏 D-31 DRAFT – Water Forward 2024 Appendix D Jun, Jul-Aug, Sep-Oct, Nov-Dec). Negative values created by subtracting b from Praw were set to zero. Variables: As part of this task, we investigate the following climate variables obtained from bias corrected GCM data: a. Annual average precipitation b. Annual average temperature c. Number of days in a year with precipitation below 0.01 inch (dry days) d. Number of days in a year with precipitation above 1 inch (wet days) e. Number of days in a year with precipitation above 2 inches (wet days) f. Number of days in a year with maximum temperature above 90°F (hot days) g. Number of days in a year with maximum temperature above 100°F (hot days) h. Number of days in a year with minimum temperature below 32°F (cold days/nights) i. Annual maximum 5-day total precipitation Given that different GCMs used different calendars (Gregorian or 360-day), when analyzing threshold-based variables (items c. to i. in the list above), we use percentage (% of the year) instead of days to track occurrence. The range of climate variables projected by the five GCMs for three SSPs are summarized over three future periods: 2021-2040 (near-term), 2041-2060 (mid-century) and 2081-2100 (end of century). These summaries are illustrated using bar charts (Figures 4, 5, S3 and S4), where the length of the bars represents the mean value and error bars show the range across the model projections. Different colors in the bar chart denote different emission scenarios. Trend Analysis We perform a trend analysis of the climate variables discussed in the above discussion over the period from 2015 to 2100 using ordinary least squares linear regression as shown below: 𝑌𝑌𝑠𝑠 = 𝑜𝑜1𝑋𝑋𝑠𝑠 + 𝑜𝑜𝑜𝑜 𝑜𝑜1 = 𝑛𝑛 𝑠𝑠=1 ∑ (𝑌𝑌𝑠𝑠 − 𝑌𝑌�)(𝑋𝑋𝑠𝑠 − 𝑋𝑋�) ∑ (𝑋𝑋𝑠𝑠 − 𝑋𝑋�) 𝑛𝑛 𝑠𝑠=1 2 (4) (5) (6) 𝑛𝑛 𝑠𝑠=1 𝑟𝑟 = Positive (negative) value of slope with progressing years, relationship, Corresponding to each ∑ (𝑌𝑌𝑠𝑠 − 𝑌𝑌�)(𝑋𝑋𝑠𝑠 − 𝑋𝑋�) 𝑛𝑛 𝑛𝑛 2 indicates that variable of interest √(∑ (𝑋𝑋𝑠𝑠 − 𝑋𝑋�) 𝑠𝑠=1 𝑠𝑠=1 . Linear correlation coefficient, 𝑜𝑜1 2 ∑ (𝑌𝑌𝑠𝑠 − 𝑌𝑌�) indicates the strength of the linear are the mean values of the variables. 𝑌𝑌𝑠𝑠 , a P-value is obtained using a lookup table. P-value less than 0.1 is is the theoretical intercept and increases (decreases) and 𝑋𝑋𝑠𝑠 𝑟𝑟 ) 𝑜𝑜𝑜𝑜 𝑟𝑟 𝑌𝑌� 𝑋𝑋� D-32 DRAFT – Water Forward 2024 Appendix D considered as the indicator of statistically significant trend. P-value greater than 0.1, indicator of statistically non-significant trend is denoted with * in Tables 3 and S1. D-33 DRAFT – Water Forward 2024 Appendix D Results Our selection of GCMs and future emission scenarios covers a wide range of end-of-century warming levels over the Colorado River Basin (Figure 2 and Table 2). Overall, all models and scenarios investigated in this study show increasing temperature by the end of the century. These results are illustrated in Figure 2 and Table 2. The values shown are the 20-year moving average of temperature anomaly with respect to the 1850 -1900 mean. Figure 3: Temperature anomalies over Colorado River Basin with respect to 1850-1900 mean. D-34 DRAFT – Water Forward 2024 Appendix D Table 2. End of century (2081-2100) global and Colorado River Basin warming levels Emission Scenario Model End of Century CRB WL (°F) Average End of Century CRB WL (°F) SSP1-2.6 EC-Earth3 SSP2-4.5 EC-Earth3 ACCESS-CM2 CNRM-CM6-1 KACE-1-0-G UKESM1-0-LL ACCESS-CM2 CNRM-CM6-1 KACE-1-0-G UKESM1-0-LL ACCESS-CM2 CNRM-CM6-1 KACE-1-0-G UKESM1-0-LL 4.96 3.97 3.06 6.63 4.65 7.32 6.76 5.81 7.77 7.15 11.87 12.34 10.89 12.58 12.18 4.66 6.96 SSP5-8.5 EC-Earth3 11.97 *Bold numbers indicate maximum and minimum end-of-century warming level Before investigating the impact of these different potential warming scenarios on local climate variables over the CRB, we compared bias-corrected data from the GCMs to observations from the period of 1991-2010 (Figure 3) for some key variables. Although models did underestimate and overestimate the observed variables, in most cases the observation falls within the overall range described by the modeled outputs. D-35 DRAFT – Water Forward 2024 Appendix D (a) Mean Temperature (b) Number of days with precipitation below 0.01 inch (c) Mean Precipitation (d) Maximum 5 day Rainfall Figure 3: Comparison between observed (PRISM) and modeled annual (a) Mean temperature, (b) Number of days with precipitation below 0.01 inch, (c) Mean precipitation and (d) Maximum 5-day rainfall for the period 1991-2010 over the Colorado River Basin. The GCMs projected that both mean and extreme values over the CRB would change by the end of the century for both temperature (Figure 4) and precipitation (Figure 5) variables. Average annual temperature and hot weather extremes as indicated by number of days in a year with maximum temperature above 100°F are projected to increase rapidly. Under the scenario with higher GHG emissions, SSP5-8.5, annual mean temperature over the CRB increases by about 11.5°F by the end of the century as compared to the current period (1991- 2010). In the same scenario, about 30% of days in a year might exceed 100°F, or about 110 days a year with temperatures in excess of 100°F. On the other hand, under higher-GHG emission scenarios extreme cold temperatures decrease, with less than 2% of the year having minimum temperatures below 32°F by the end of the century. In depth investigation of the underlying processes is required to understand the impact of warming on winter storms over this region. Under the scenario with higher GHG emissions, the frequency and magnitude of precipitation extremes increase, as indicated by both annual maximum 5-day rainfall and number of days in a year with precipitation above 2 inches. This increase is higher towards the end of century and D-36 DRAFT – Water Forward 2024 Appendix D under higher emissions scenarios. Similar conclusions are indicated for the dry extremes, as projections for the number of days below 0.01 inch increases. (a) Mean Temperature (b) Annual days with minimum temperature below (c) Annual days with maximum temperature above (d) Annual days with maximum temperature above Figure 4: Range of annual (a) Mean temperature (b) Number of days with temperature below 32°F, (c) Number of days with temperature above 90°F and (d) Number of days with maximum temperature above 100°F projected by GCMs over the Colorado River Basin. (The length of the bars represents the mean value and error bars show the range across the model projections). D-37 DRAFT – Water Forward 2024 Appendix D Figure 5: Range of annual (a) Mean precipitation, (b) Maximum 5-day precipitation, (c) Number of days with precipitation below 0.01 in, (d) Number of days with precipitation above 1 in and (e) Number of days D-38 DRAFT – Water Forward 2024 Appendix D (a) Mean Precipitation (b) Annual Maximum 5 day Precipitation (c) Annual days with precipitation below 0 01 in (d) Annual days with precipitation above 1 in (e) Annual days with precipitation above 2 in with precipitation above 2 in over the Colorado River Basin projected by GCMs. (The length of the bars represents the mean value and error bars show the range across the model projections) D-39 DRAFT – Water Forward 2024 Appendix D The 85-year (2015 to 2100) trend analysis (Time series shown in Figure S2 – S3) of the variables aligns with the above-mentioned findings inferred from the bar charts of the projections of the GCMs (Table 3). For example, the number of days in a year with maximum temperature exceeding 100°F increases at a rate of 0.29 to 0.37 % per year under the SSP5-8.5 scenario according to the GCMs. Considering 365 days in a year, that would mean it is increasing at a rate of 1.1 to 1.4 days/year. Disagreement among the models about trends in precipitation related variables are higher than in temperature related variables. Also, trends in precipitation variables are found to be statistically not significant more often than temperature related variables. This can be because we assessed linear trends in these variables, whereas the trend can be non-linear and non-monotonic. The projections of most of these variables over Austin are similar to those over CRB (Figure S4 – S9 and Table S1 in appendix). Table 3. Summary of Trend Analysis of climate variables over Colorado River Basin (2015-2100). Numbers show the minimum and maximum trends from individual GCM Variable Emission Scenario SSP1-2.6 SSP2-4.5 SSP5-8.5 Unit Mean Annual Temperature 0.02 to 0.03 0.05 to 0.07 0.12 to 0.15 °F /Year Annual Number of Days with Maximum Temperature above 90°F Annual Number of Days with Maximum Temperature above 100°F Annual Number of Days with Minimum Temperature below 32°F 0.05 to 0.09 0.11 to 0.18 0.29 to 0.38 %/ Year 0.02 to 0.08 0.07 to 0.17 0.29 to 0.37 %/ Year -0.04 to -0.01* -0.08 to -0.03 -0.10 to -0.07 %/ Year Mean Annual Precipitation 0.004 to 0.03* -0.05 to 0.03* -0.05 to 0.08* Annual Number of Days with Precipitation below 0.01" Annual Number of Days with Precipitation above 1" Annual Number of Days with Precipitation above 2" -0.006 to 0.02* -0.01 to 0.03* -0.03 to 0.06 %/ Year 0.0003 to 0.003* -0.0003 to 0.002* -0.001 to 0.007* 0.001 to 0.003 -0.005 to 0.004* -0.001 to 0.002* 0.0002 to 0.01* Annual Maximum 5-day Precipitation -0.005 to 0.01* 0.004 to 0.02* *Not statistically significant at α = 10% Inch/ Year %/ Year %/ Year Inch/ Year D-40 DRAFT – Water Forward 2024 Appendix D Conclusions In this task, we analyzed future projections of climate variables for five GCMs under three emission scenarios which cover a wide range of end-of-century warming levels over the Colorado River Basin. We investigated the impact of these different potential warming scenarios on local climate variables over the CRB area. Our analysis shows that over the CRB, through the end of the century: • Annual temperature is increasing • Number of hot days (with temperature above 100°F) are increasing • Number of cold days (with temperature below 32°F) are decreasing • Frequency and magnitude of heavy rainfall events (as indicated by number of days with precipitation above 2” and annual maximum 5-day rainfall) increases under higher emissions. • Number of dry days (with rainfall less than 0.01”) are increasing • Overall annual precipitation does not change significantly Change in frequency distribution of rainfall with shift towards more heavy rainfall as well as a decrease in overall rainfall frequency could explain the disparate changes in variables associated with rainfall (Trenberth, 1999). The magnitude of these changes varies across GCMs as well as across different emission scenarios. The changes over the Austin area are consistent with the changes over the CRB area. How these changes impact the water availability over this region will be assessed in later tasks of this project. Temperature •Annual Mean Temperature projected to increase •Number of hot days with temperature > 100°F projected to increase •Rainfall distribution projected to change •Less frequent and more intense rainfall events projected Rainfall Dry Days •Number of dry days with precipitation < 0.01 in projected to increase Figure 6: Summary of trend analysis over the Colorado River Basin D-41 DRAFT – Water Forward 2024 Appendix D D-42 DRAFT – Water Forward 2024 Appendix D References Accadia, C., Mariani, S., Casaioli, M., Lavagnini, A., & Speranza, A. (2003). Sensitivity of Precipitation Forecast Skill Scores to Bilinear Interpolation and a Simple Nearest-Neighbor Average Method on High-Resolution Verification Grids. Weather and Forecasting, 18(5), 918–932. Austin Water (2018). A Water Plan for the Next 100 year. https://austintexas.gov/sites/default/files/files/Water/WaterForward/Water_Forward_Plan_Re port_-_A_Water_Plan_for_the_Next_100_Years.pdf Daly, C., Halbleib, M., Smith, J.I., Gibson, W.P., Doggett, M.K., Taylor, G.H., Curtis, J., Pasteris, P.P., 2008. Physiographically sensitive mapping of climatological temperature and precipitation across the conterminous United States. Int. J. Climatol. 28, 2031–2064. https://doi.org/10.1002/joc.1688 Eyring, V., Bony, S., Meehl, G. A., Senior, C. A., Stevens, B., Stouffer, R. J., & Taylor, K. E. (2016). Overview of the Coupled Model Intercomparison Project Phase 6 (CMIP6) experimental design and organization. Geoscientific Model Development, 9(5), 1937–1958. Hargraves, G.H. and Z.A. Samani, Estimating (1982). PET, Tech Note, J. Irrig. Drain Eng., vo. 108, no. 3 pp. 225-230. Hargraves, G.H. and Z.A. Samani, (1985). Reference Crop Evapotranspiration from Temperature, Appl. Eng. Agric., vol 1, no. 2, pp. 96-99. IPCC, 2021: Summary for Policymakers. In: Climate Change 2021: The Physical Science Basis. Contribution of Working Group I to the Sixth Assessment Report of the Intergovernmental Panel on Climate Change [Masson-Delmotte, V., P. Zhai, A. Pirani, S. L. Connors, C. Péan, S. Berger, N. Caud, Y. Chen, L. Goldfarb, M. I. Gomis, M. Huang, K. Leitzell, E. Lonnoy, J.B.R. Matthews, T. K. Maycock, T. Waterfield, O. Yelekçi, R. Yu and B. Zhou (eds.)]. Cambridge University Press. Jones, P. W. (1999). First- and Second-Order Conservative Remapping Schemes for Grids in Spherical Coordinates. Monthly Weather Review, 127(9), 2204–2210. Taylor, K. E., Stouffer, R. J., & Meehl, G. A. (2012). An Overview of CMIP5 and the Experiment Design. Bulletin of the American Meteorological Society, 93(4), 485–498. https://doi.org/10.1175/BAMS-D-11-00094.1 Trenberth, K. E., (1999). Conceptual framework for changes of extremes of the hydrological cycle with climate change. Climatic Change, 42, 327–339, doi:10.1023/A:1005488920935. TWDB (2020). The Texas Water Development Board Lake Evaporation and Precipitation dataset. https://waterdatafortexas.org/lake-evaporation-rainfall Meehl, G. A., Moss, R., Taylor, K. E., Eyring, V., Stouffer, R. J., Bony, S., & Stevens, B. (2014). Climate Model Intercomparisons: Preparing for the Next Phase. Eos, Transactions American Geophysical Union, 95(9), 77–78. https://doi.org/10.1002/2014EO090001 Nielsen-Gammon, J., S. Holman, A. Buley, S. Jorgensen, J. Escobedo, C. Ott, J. Dedrick, and A. Van Fleet, 2021: Assessment of Historic and Future Trends of Extreme Weather in Texas, 1900-2036: 2021 Update. Document OSC-202101, Office of the State Climatologist, Texas A&M University, College Station, 44 pp. D-43 DRAFT – Water Forward 2024 Appendix D Nielsen Gammon, J. W., Banner, J. L., Cook, B. I., Tremaine, D. M., Wong, C. I., Mace, R. E., et al. (2020). Unprecedented drought challenges for Texas water resources in a changing climate: What do researchers and stakeholders need to know? Earth's Future, 8, e2020EF001552. ‐ USGCRP (2017). Climate Science Special Report: Fourth National Climate Assessment, Volume I Wuebbles, D.J., D.W. Fahey, K.A. Hibbard, D.J. Dokken, B.C. Stewart, & T.K. Maycock (eds.). Washington, DC: U.S. Global Change Research Program. D-44 DRAFT – Water Forward 2024 Appendix D Task 3 Appendix 709 710 809 810 Figure S1: 27 quadrangles that cover the Colorado River Basin. The yellow rectangle encompasses the four quadrangles closest to Austin. D-45 DRAFT – Water Forward 2024 Appendix D (a) Mean Temperature (b) Annual days with minimum temperature below 32°F (c) Annual days with maximum temperature above (d) Annual days with maximum temperature above Figure S2: Time series of annual (a) Mean temperature (b) Number of days with temperature below 32°F, (c) Number of days with temperature above 90°F and (d) Number of days with maximum temperature above 100°F from 2015 to 2100 projected by GCMs over the Colorado River Basin. (Thick lines depict the mean of the projections given by the 5 GCMs for each emission scenario). D-46 DRAFT – Water Forward 2024 Appendix D (a) Mean Precipitation (b) Annual Maximum 5 day Precipitation (c) Annual days with precipitation below 0 01 (d) Annual days with precipitation above 1 in (e) Annual days with precipitation above 2 in Figure S3: Time series of annual (a) Mean precipitation, (b) Maximum 5-day precipitation, (c) Number of days with precipitation below 0.01 in, (d) Number of days with precipitation above 1 in and (e) Number of D-47 DRAFT – Water Forward 2024 Appendix D days with precipitation above 2 in from 2015 to 2100 projected by GCMs over the Colorado River Basin. (Thick lines depict the mean of the projections given by the 5 GCMs for each emission scenario). D-48 DRAFT – Water Forward 2024 Appendix D (a) Mean Temperature (b) Number of days with precipitation below (c) Mean Precipitation (d) Maximum 5 day Rainfall Figure S4: Comparison between observed (PRISM) and model annual (a) Mean temperature, (b) Number of days with precipitation below 0.01 inch, (c) Mean precipitation and (d) Maximum 5-day rainfall for the period 1991-2010 over Austin D-49 DRAFT – Water Forward 2024 Appendix D (a) Mean Temperature (b) Annual days with minimum temperature below (c) Annual days with maximum temperature above (d) Annual days with maximum temperature above Figure S5: Range of annual (a) Mean temperature (b) Number of days with temperature below 32°F, (c) Number of days with temperature above 90°F and (d) Number of days with maximum temperature above 100°F projected by GCMs over Austin. (The length of the bars represents the mean value and error bars show the range across the model projections) D-50 DRAFT – Water Forward 2024 Appendix D (a) Mean Precipitation (b) A l M i 5 d (c) Annual days with precipitation below 0 01 (e) Annual days with precipitation above 1 in (f) Annual days with precipitation above 2 in D-51 DRAFT – Water Forward 2024 Appendix D Figure S6: Range of annual (a) Mean precipitation, (b) Maximum 5-day precipitation, (c) Number of days with precipitation below 0.01 in, (d) Number of days with precipitation above 1 in and (e) Number of days with precipitation above 2 in over Austin projected by CMIP6 GCMs. (The length of the bars represents the mean value and error bars show the range across the model projections) (a) Mean Temperature (b) Annual days with minimum temperature below (c) Annual days with maximum temperature above (d) Annual days with maximum temperature above Figure S7: Time series of annual (a) Mean temperature (b) Number of days with temperature below 32°F, (c) Number of days with temperature above 90°F and (d) Number of days with maximum temperature D-52 DRAFT – Water Forward 2024 Appendix D above 100°F from 2015 to 2100 projected by GCMs over Austin. (Thick lines depict the mean of the projections given by the 5 GCMs for each emission scenario). D-53 DRAFT – Water Forward 2024 Appendix D (a) Mean Precipitation (b) Annual Maximum 5 day Precipitation (c) Annual days with precipitation below 0 01 (d) Annual days with precipitation above 1 in (e) Annual days with precipitation above 2 in Figure S8: Time series of annual (a) Mean precipitation, (b) Maximum 5-day precipitation, (c) Number of days with precipitation below 0.01 in, (d) Number of days with precipitation above 1 in and (e) Number of D-54 DRAFT – Water Forward 2024 Appendix D days with precipitation above 2 in from 2015 to 2100 projected by GCMs over Austin. (Thick lines depict the mean of the projections given by the 5 GCMs for each emission scenario). Table S1. Summary of trend analysis of climate variables over Austin (2015-2100) Variable Emission Scenario SSP1-2.6 SSP2-4.5 SSP5-8.5 Unit Mean Annual Temperature 0.02 to 0.03 0.04 to 0.07 0.11 to 0.14 °F /Year Annual Number of Days with Maximum Temperature above 90°F Annual Number of Days with Maximum Temperature above 100°F Annual Number of Days with Minimum Temperature below 32°F 0.05 to 0.10 0.10 to 0.18 0.26 to 0.37 %/ Year 0.05 to 0.10 0.08 to 0.21 0.30 to 0.39 %/ Year -0.03 to -0.02* -0.06 to -0.02 -0.06 to -0.04 %/ Year Mean Annual Precipitation -0.01 to 0.04* -0.05 to 0.04* -0.05 to 0.08* Annual Number of Days with Precipitation below 0.01" Annual Number of Days with Precipitation above 1" Annual Number of Days with Precipitation above 2" -0.004 to 0.02* 0.03 to 0.04 0.06 to 0.07 %/ Year -0.001 to 0.003* -0.002 to 0.002* -0.008 to 0.006 %/ Year -0.001 to 0.007* -0.002 to 0.004* 0.003 to 0.004 %/ Year Annual Maximum 5-day Precipitation -0.01 to 0.01* -0.01 to 0.03* -0.01 to 0.02* *Not statistically significant at α = 10% Inch/ Year Inch/ Year D-55 DRAFT – Water Forward 2024 Appendix D Task 4: Hydrologic Modeling January 2023 Prepared by: Hoffpauir Consulting, with support from The University of Texas at Austin Summary The goal of this task is to produce a set of projected naturalized flows at pertinent locations throughout the Colorado River Basin that can be used within Water Forward’s water availability model (WAM). Here we develop a naturalized flow modeling framework using inputs derived from precipitation, temperature, and evaporation data. To develop projected naturalized flows, a hydrologic model is developed and trained to replicate observed naturalized flows over the historical period of record contained in the Texas Commission on Environmental Quality (TCEQ) WAM. After the hydrologic model for the historical period is established, simulated projections of future precipitation and temperature from general circulation models (GCMs) are used as inputs to produce projections of naturalized flows through the end of the 21st century. We find a high degree of agreement between the modeled and the WAM naturalized flow for the mean, standard deviation, and median values at most control points for the 1983–2016 observational period. The future flow projections indicate an overall declining flow trend across the basin with the potential for flood events exceeding those in the observational record, which is consistent with the expected rainfall-runoff response to rising temperatures and changes in the frequency and magnitude of rain events. The projections of future naturalized flow conditions under various climate conditions are a key input to the Water Forward WAM analyses. D-56 DRAFT – Water Forward 2024 Appendix D SSP TCEQ TWDB USGS WAM WRAP List of Acronyms ANN Artificial Neural Network CRB Colorado River Basin GCM General Circulation Model (or Global Climate Model) PRISM Parameter-Elevation Regressions on Independent Slopes Model Shared Socioeconomic Pathway Texas Commission on Environmental Quality Texas Water Development Board United States Geological Survey Water Availability Model Water Rights Analysis Package D-57 DRAFT – Water Forward 2024 Appendix D Introduction In response to global warming, the hydrologic cycle is intensifying (Huntington, 2006). A warmer atmosphere has an increased capacity of holding moisture, which, in turn, leads to more evaporation and precipitation (Held & Soden, 2006). As a result, the frequency and severity of hydrological extreme events such as floods and droughts are increasing (Trenberth et al., 2003, Allan & Soden, 2008, Sheffield & Wood, 2008). Texas is characterized by extreme hydrologic variability both spatially and temporally. Historically, drought and flood events in Texas tend to follow each other. For instance, both the 1950s and the 2010-2015 droughts, and many other shorter duration drought events, ended in intense rainfall leading to flooding (Texas’ Extreme Weather, 2016). Colorado river basin (CRB), one of the largest river basins by area within Texas, is prone to both floods and droughts. Many communities, including the City of Austin, rely on the Colorado River for nearly all of their municipal water. Any change in the hydrology of this river directly affects the water availability in these communities. In Task 3 of this project, we analyzed future projections of climate variables for five GCMs under three emission scenarios (SSP1-2.6, SSP2-4.5 and SSP5-8.5) over the CRB. Our analysis shows an increase in temperature as well as a shift in precipitation pattern in this region. Both heavy rainfall days and dry days are projected to increase over the CRB. Rising temperatures and changes in the frequency and magnitude of rain events will likely impact the hydrology of the CRB. The TCEQ WAM (TCEQ, 2022) is a collection of model input files covering all state-granted water rights across all river basins in Texas and a generalized computer modeling platform called the Water Rights Analysis Package (WRAP) (Wurbs, 2021). The WAM is used by state agencies, water right holders, and stakeholders to simulate water availability for a specified water management scenario through a repeat of historical hydrology. Historical hydrology is comprised of monthly naturalized stream flows and net evaporation, generally covering the past 60 to 80 years. Naturalized stream flows are a computed amount of monthly flow volume that would have been present in the river in the absence of state-granted water rights. The Colorado River Basin WAM is used in the City of Austin’s Water Forward planning process. Simulations are comprised of basin-wide demands at future time horizons paired with either historical hydrology or projections of future hydrologic conditions. The Colorado WAM simulations are used to evaluate portfolios of water management strategies for the Water Forward planning process, especially during drought events contained in the input hydrologic sequences. The projections of future naturalized flow conditions under various climate conditions are a key input to the Water Forward WAM analyses. Precipitation and temperature projections of GCMs are used as inputs to produce projections of naturalized flows through the end of the 21st century. The future flow projections will be paired with future basin-wide demand projections in the Water Forward WAM simulations. The second hydrologic input to the WAM, net evaporation (mentioned above), can be developed directly from the precipitation and temperature outputs of the GCMs. Literature Review A model is a simplified representation of real-world system (Moradkhani & Sorooshian, 2008). Hydrological models are mainly used for understanding various hydrological processes and estimating runoff as a function of various parameters used for describing watershed characteristics (Devia et al., 2015). Hydrologic models can be broadly divided into two types: (i) process or physically based models and (ii) data-driven or statistical models. Physically based D-58 DRAFT – Water Forward 2024 Appendix D models require in-depth knowledge of catchment characteristics and have extensive data requirements (Ni et al., 2020; Yuan et al., 2018). Physical models, although deterministic and reliable, do not always perform and scale well due to their intrinsic limitations (Islam, 2011). On the other hand, statistical models extract information from existing data without considering the features and processes of hydrological system (Ni et al., 2020; Yuan et al., 2018). Statistical models can be developed quickly but have limitations in terms of accuracy and non-stationarity. In recent years progress has been made in developing hybrid statistical-physical models (Wegayehu & Behulu, 2021; Slater et al., 2018). Statistical models for streamflow simulation range from simple regression to sophisticated machine learning algorithms such as an artificial neural network (ANN) (Das Bhowmik et al., 2018). Regression, one of the simplest and most widely used statistical model, establishes a functional relationship between two variables, referred to as the dependent variable and the independent variable. Multiple linear regression (MLR), also known as multivariate regression model, is an extension of simple linear regression and establishes relationship between a dependent variable and a set of independent variables (Asati & Rathore, 2012). ANNs are analogous in application to MLR, with the added advantage that they are inherently non-linear, and particularly robust in finding and representing relationships in the presence of noisy data (Akhter, 2017). ANN-based hydrological models are widely documented in literature. Poff et al. (1996) used ANNs to evaluate the changes in stream hydrograph from speculative climate change scenarios based on precipitation and temperature changes. A comprehensive review of the application of ANNs to hydrology can be found in American Society of Civil Engineers (ASCE) Task Committee on Application of Artificial Neural Networks in Hydrology (2000a, b), in Maier and Dandy (2000) and in Akhter (2017). ANNs have become popular in the last decade for hydrological forecasting such as rainfall-runoff modeling, streamflow forecasting, groundwater and precipitation forecasting, and water quality issues (Sethi et al., 2010; Banerjee et al., 2009; Sahoo and Ray, 2006; Kisi, 2004). The most widely used neural network is the multilayer perceptron (MLP) (Akhter, 2017). Methodology Overview The initial Water Forward Plan (WF18), delivered in 2018, used a multivariate flow modeling approach which is described in Chapter 5 and Appendix D of the WF18 Report. Historical precipitation and temperature data were used to create weather variables that were used as inputs to multivariate models at stream gaging locations across the Colorado River Basin to model historical naturalized flows. The multivariate models were then given future projections of precipitation and temperature from GCMs to project future stream flows. For the 2024 Water Forward (WF24) plan update, similar multivariate models are adopted with improved selection of features and inclusion of neural networks for better model performances. The methodology followed for modeling naturalized flows can be categorized into 4 four general tasks: • Assembling and preprocessing input data. • Associating locations of weather data to the naturalized flow control points. • Selecting precipitation and temperature variables for each control point. • Creating flow model architecture and performing model training. D-59 DRAFT – Water Forward 2024 Appendix D Input Data Assembly The input data used in this study were either historical naturalized flow and historical weather or future projections of weather. The weather variables used in this study were daily values of precipitation, maximum surface air temperature, and minimum surface air temperature. Several sources were used for historical observations and future weather projections. The input data for the following categories are described further in this section: • Historical naturalized stream flow. • Historical gaged spring flow. • Historical precipitation, temperature, and evaporation. • GCM projections of future precipitation and temperature. The Colorado WAM contains 43 locations (control points) where naturalized stream flows are provided as input. Several control points also provide spring flow discharge in the WAM, but only two control points are derived from gage measurements; the other spring flow control points are estimated using equations. In total, there are 45 control points in the Colorado WAM with either naturalized stream flow or gaged spring flow inputs. The flows are monthly volumes, in acre-feet, covering a 1940–2016 period of record. Figures 2.1 through 2.3 show the geographic locations of the WAM control points, their control point identifiers, and the connectivity between control points. Within the figures, the naturalized streamflow control points along the main stem of the Colorado River are shown in blue. Naturalized flow control points along tributaries are shown in gray. Spring flow control points are shown in green. Table 2.1 lists WAM control points, USGS gage association, and drainage area. D-60 DRAFT – Water Forward 2024 Appendix D Figure 2.1 Colorado River Basin and WAM control points. D-61 DRAFT – Water Forward 2024 Appendix D Figure 2.2 Colorado WAM control point identifiers. D-62 DRAFT – Water Forward 2024 Appendix D Figure 2.3 Colorado WAM control point connectivity. (Extraneous zeroes were removed from the stream flow control point identifiers. The figure is not shown to scale.) Table 2.1 WAM control points, USGS gage association, and drainage area. WAM Control Point Identifier Description USGS Gage WAM Contributing Drainage Area, sq. mi. A30000 Colorado Rv. near Ira 08119500 1,074 A20000 Deep Creek near Dunn 08120500 193 A10000 Colorado Rv. at Colorado City 08121000 1,575 D-63 DRAFT – Water Forward 2024 Appendix D B40000 Champion Creek Reservoir 08123600 B30000 Beals Creek near Westbrook 08123800 B20000 Colorado River above Silver 08123850 B10000 Colorado River at Robert Lee 08124000 C70000 North Concho River near Carlsbad 08134000 C60000 Middle Concho River above Tankersley 08128400 C50000 Spring Creek above Tankersley 08129300 C40000 Dove Creek at Knickerbocker 08130500 C40130 Dove Creek Spring near Knickerbocker 08129500 C30000 South Concho River at Christoval 08128000 C20000 Concho River at San Angelo 08136000 C10000 Concho River at Paint Rock 08136500 D40000 Colorado River at Ballinger 08126380 D30000 Elm Creek at Ballinger 08127000 D20000 Colorado River near Stacy 08136700 12,548 D10000 Colorado River at Winchell 08138000 13,788 E40000 San Saba River at Menard 08144500 E30000 San Saba River near Brady 08144600 E20000 Brady Creek at Brady 08145000 E10000 San Saba River at San Saba 08146000 F30000 Pecan Bayou at Brownwood 08143500 F20000 Pecan Bayou near Mullin 08143500 F10000 Colorado River near San Saba 08147000 19,830 G50000 North Llano River near Junction 08148500 G40000 Llano River near Junction G30000 Llano River near Mason 08150000 08150700 176 1,974 4,559 5,046 1,202 1,613 340 164 na* 258 4,139 5,185 6,090 464 1,137 1,636 589 3,048 1,654 2,074 897 1,859 3,251 D-64 DRAFT – Water Forward 2024 Appendix D G20000 Beaver Creek near Mason 08150800 215 G10000 Llano River at Llano 08151500 4,201 H20000 Pedernales River near Fredericksburg 08152900 H10000 Pedernales River near Johnson City 08153500 I40000 I30000 I20000 I10330 I10000 Lake Buchanan near Burnet 08148000 20,521 Sand Creek near Kingsland 08152000 346 Lake Travis near Austin 08154500 27,357 Barton Springs at Austin 08155500 na Colorado River at Austin 08158000 27,606 J50000 Onion Creek at Driftwood 08158700 J40000 Onion Creek at US Hwy 183 08159000 J30000 Colorado River at Bastrop 08159200 28,576 J20000 Colorado River at Smithville 08159500 28,968 J10000 Colorado River at Columbus 08161000 30,327 K20000 Colorado River at Wharton 08162000 30,600 K10000 Colorado River at Bay City 08162500 30,837 370 901 124 321 *Drainage area estimates not applicable to spring flow gages. The Texas Water Development Board (TWDB) publishes monthly values of precipitation and lake evaporation covering the state in a 1° latitude by 1° longitude grid, hereafter referred to as quadrangles (TWDB, 2022). The monthly precipitation is subtracted from evaporation to calculate net evaporation, which is used in the WAM for modeling water loss or gain directly on the water surfaces of reservoirs. A total of 27 quadrangles, shown in Figure 2.4, were identified for this study based upon their location within or surrounding the Colorado River Basin. TWDB monthly precipitation data are updated annually and are available from 1940 through present. The monthly evaporation data are also updated annually and are available from 1954 through present. The data, which are provided in units of inches, were downloaded for the 27 selected quadrangles. D-65 DRAFT – Water Forward 2024 Appendix D Figure 2.4 Quadrangle locations for Colorado River Basin weather data. Quadrangle centers are shown in red squares with the number quadrangle identifier. Daily historical weather data with a grid resolution of 4 kilometers from the Oregon State University Parameter-Elevation Regressions on Independent Slopes Model (PRISM) (Daly et al., 2008) are available for the United States. Daily precipitation, maximum surface air temperature, and minimum surface air temperature were downloaded at the location of the 27 selected quadrangle centers. The daily PRISM data have a period of record that begins January 1, 1981. The TWDB precipitation data were used to check the monthly totals of daily precipitation from the PRISM dataset. The TWDB evaporation data were used to calibrate an evaporation equation for each quadrangle. Historical projections of evaporation used in this study were calculated with a temperature-based Hargreaves equation (Hargreaves, 1982; Hargreaves et al., 1985). Hargreaves evaporation values were used in lieu of TWDB historical evaporation to maintain consistency across the historical and future modeling periods. The TWDB monthly evaporation data were used as a training set to calibrate the coefficient values of the Hargreaves equation for each quadrangle. Daily maximum and minimum surface air temperature, along with daily extraterrestrial solar radiation (Ra), are used by the Hargreaves equations to create daily evaporation. The daily evaporations were aggregated to monthly values that were used to adjust the equation coefficients for the best fit with the TWDB monthly evaporation. D-66 DRAFT – Water Forward 2024 Appendix D Precipitation, maximum surface air temperature, and minimum surface air temperature are available from 5 GCMs from a historical simulation and 3 future emission scenarios. The historical GCM simulations cover the period of 1850–2014, and the projection simulations cover 2015-2100 (Details provided in Task 3 Memo). The data were extracted from the GCMs with daily time steps and were converted to units of inches of precipitation and degrees Fahrenheit for temperature. Periods of Records The historical daily observed weather from PRISM was used in this study to associate locations of weather data to the naturalized flow control points and to select precipitation and temperature variables for each control point. PRISM daily weather data begin with 1981. However, as will be described in the following section, the first two years of daily weather data are needed for the creation of weather variables, hereafter referred to as features, which can span up to 24 months of averaging. Thus, to accommodate the feature averaging periods, the WAM naturalized flow period of record used in this study was 1983–2016. This time period provided 408 months of historical naturalized flows and weather feature values for associating weather locations and selecting features. The GCM historical simulations use forcings of historical greenhouse gas emissions, but the simulated weather outputs vary stochastically compared to historical precipitation and temperature. Thus, the GCM historical simulation data were not used for associating weather locations or selecting features based on the pattern of observed historical flows. However, once the weather locations and feature selections were established using observed weather data, the historical 1981–2014 GCM weather data were used as inputs to train the multivariate model to reproduce statistical measures of naturalized flow in the WAM historical period of 1983–2016. Finally, the weather data from the GCM future simulations were fed as inputs into the trained multivariate model to produce projected monthly naturalized flows through 2100. Preprocessing feature classes Baseline feature classes were calculated after assembling the daily weather data from the PRISM and GCM sources. The baseline feature classes are chosen to represent weather conditions that are potentially related to the production of naturalized flow across the watershed above each control point. The baseline feature classes are constructed by aggregating daily values of precipitation or evaporation to monthly totals, counting the days per month without precipitation, or averaging daily values of temperature or solar radiation across the month. An additional set of baseline precipitation features was created by subtracting daily evaporation, which was calculated from the Hargreaves equation, from daily precipitation. Days in which evaporation exceeded precipitation resulted in negative values and were reset to zero. The 25 baseline feature classes are: 1. Total monthly precipitation. 2. Total monthly precipitation only for days that exceed 0.25 inches. 3. Total monthly precipitation only for days that exceed 0.5 inches. 4. Total monthly precipitation only for days that exceed 1.0 inches. 5. Total monthly precipitation only for days that exceed 2.0 inches. 6. Maximum 3-day precipitation per month. 7. Maximum 7-day precipitation per month. 8. Number of days per month with less than 0.04 inches (1 mm) of precipitation (dry days). D-67 DRAFT – Water Forward 2024 Appendix D 9. Monthly total of daily precipitation minus evaporation (precip-evap). 10. Total monthly precip-evap only for days that exceed 0.25 inches. 11. Total monthly precip-evap only for days that exceed 0.5 inches. 12. Total monthly precip-evap only for days that exceed 1.0 inches. 13. Total monthly precip-evap only for days that exceed 2.0 inches. 14. Maximum 3-day precip-evap per month. 15. Maximum 7-day precip-evap per month. 16. Number of days per month with zero precip-evap (dry days). 17. Total monthly evaporation. 18. Monthly average of the daily extraterrestrial solar radiation. 19. Monthly average of the daily average air temperature per month (average of the daily maximum and minimum air temperature). 20. Monthly average of the daily maximum air temperature. 21. Hottest 7-day average of the maximum air temperature per month. 22. Monthly average of the daily minimum air temperature. 23. Coldest 7-day average of the minimum air temperature per month. 24. Monthly maximum of averaged daily precipitation. 25. Monthly maximum of averaged daily precip-evap. Feature Averaging Monthly naturalized flows are reflective of weather in the current month as well as previous months. Accordingly, the 25 baseline feature classes were expanded by applying moving averages to create monthly values indicative of weather events in prior months and longer-term conditions. An alternative approach to applying moving averages at this step in the framework is to use feedback connections within the flow model to internally lag weather inputs from previous months. This alternative will expand the complexity within the flow model and was not explored here. Feature classes 1 through 23 listed in the previous section are monthly values. Arithmetic moving averages of 2 to 24 months were used to create a total of 24 features when including the current month’s value as its own feature. For example, the total monthly precipitation at each quadrangle was expanded into 24 features that include the current month’s value, a 2- month average, a 3-month average, and so forth. Monthly precipitation features representing extremes, such as the monthly total precipitation for days exceeding 1 inch, are only arithmetically averaged up to 3 months and only exponentially averaged up to a smoothing term of 7, as described below. In addition to arithmetic moving averages, features were created with an exponential moving average. Exponential moving averages give more weight to more recent values than arithmetic averages in which all months are equally weighted. A smoothing term, N, was incremented with the exponential moving averages to create a range of smoothing. The weight of the current month was assigned using a value equal to 2/(N + 1). The previous value of the exponential moving average is assigned a weight equal to 1 minus the weight of the current month. For example, N = 7 applies 25% weight to the current month and 75% weight to the previous value of the exponential moving average. Figure 2.5 illustrates a sequence of monthly precipitation, 4- month arithmetic moving average in which all months are weighted 25%, and an exponential moving average in which only the current month is weighted 25%. For each class, up to 48 features are created at each quadrangle covering the current monthly value, 2 to 24 months of D-68 DRAFT – Water Forward 2024 Appendix D arithmetic moving averages, and up to 24 exponentially weighted moving averages with smoothing terms between 1.67 and 24. ) s e h c n i ( n o i t a t i p i c e r P 6 5 4 3 2 1 0 Current Month Only 4 Month Arithmetic Average Exponential Average, N=7 Figure 2.5 Example of moving averages. Feature classes 24 and 25 were derived directly from daily values of precipitation and precipitation minus evaporation. These data were first smoothed with an exponential moving average on a daily time step before creating a monthly feature value. Smoothed daily time series are calculated using exponential smoothing terms 4 to 96, incremented by 4. After smoothing on a daily time step, the maximum daily value per month is selected as the monthly feature value. A total of 24 monthly precipitation and 24 monthly precipitation minus evaporation features were created from the daily time series. A total of 830 features were created by expanding the initial 25 baseline feature classes with arithmetic and exponential moving averages. The features each consist of monthly values representing the weather variable with various levels of averaging. The process of creating features was applied to all 27 quadrangles in this study for a total of 22,410 features across the entire basin. Quadrangle Associations The 1° by 1° grid spacing of the weather data locations encompasses the basin but does not necessarily place locations within the watershed boundaries represented by the naturalized flow control points. Quadrangle locations were manually selected based on proximity to the watershed upstream of each control point to represent weather conditions that contribute to naturalized flow. Once a select set of quadrangles were assigned to each naturalized flow control point, each quadrangle was assigned a coefficient based on the estimated relationship to naturalized flow at the control point. The coefficients assigned to each quadrangle at each control point were values between 0.0 and 1.0, and the coefficients collectively sum to 1.0. Multiplying each feature at each quadrangle by the respective coefficient and adding them together created a single composite feature that reflects the estimated relationship of each quadrangle to naturalized flow. D-69 DRAFT – Water Forward 2024 Appendix D The goal of this step in the framework was to select the relevant quadrangles for each control point and to pre-calculate their coefficients. A fixed set of quadrangle coefficients is necessary for the next step in the framework, which involves selecting a set of composite features for modeling naturalized flow. An alternative approach is to allow the feature selection and the flow model training processes to optimize the quadrangle coefficients. However, this approach greatly increases the number of parameters being optimized in both processes. Thus, this alternative was not explored in this study. Selection of Quadrangles Visual selection was used to designate 2 to 5 quadrangles that are adjacent or within the incremental drainage area of each control point. Incremental drainage area refers to the watershed between control points if any upstream control points are present. Incremental drainage area is used because the flow model trained at each control point connects to the flow model at the next downstream control point. With this connectivity, the flow model for each control point will primarily be trained to produce the flows originating in the watershed between control points. Correlation of Flow and Precipitation Features The quadrangle coefficients were created by adjusting their values to maximize the correlation between composite precipitation features and the naturalized flow. Precipitation and flow have a nonlinear relationship, whereas the commonly used Pearson’s correlation coefficient measures the strength of a linear relationship. Thus, Spearman’s rank correlation was used to account for the nonlinearity and to measure the strength of the monotonic relationship between precipitation and naturalized flow, wherein monotonic refers to a relationship that is consistently increasing or decreasing. Spearman’s correlation was calculated as the correlation between the ranks of the two variables. Once the quadrangles were selected, 84 precipitation features at each quadrangle location were used in the correlation analysis. The selected features were chosen to represent a range of temporal and event-based relationships of precipitation to flow. The selected precipitation features include monthly totals as well as threshold measures, such as the monthly total of daily precipitation exceeding 1 inch. The selected features only cover the first 7 exponential averaging periods. Shorter-term exponential averaging periods were used since they were found to have the strongest relationship with naturalized flow, and the correlation of naturalized flow with longer-term precipitation and temperature features were observed to be less sensitive to changes in quadrangle coefficients. The quadrangle coefficients were optimized for maximization of the rank correlation of each composite feature with naturalized flow. Some quadrangles selected for a control point may have little or no coverage over the control point’s contributing watershed. Although proximity of the quadrangles to the control point’s drainage area was considered, no attempt was made to explicitly explain geographical or physical links between each quadrangle’s precipitation time series and naturalized flow. It was assumed that maximization of rank correlation between the composite feature and naturalized flow is a suitable method for determining the relative influence of each quadrangle’s precipitation time series on the observed naturalized flow signal. It was also assumed that the quadrangle coefficients of the selected shorter-term precipitation features are acceptable for creating composite features from the temperature and longer-term average precipitation features. D-70 DRAFT – Water Forward 2024 Appendix D Figure 3.1 shows Control Point I30000, Sandy Creek near Kingsland, and the center points of the two selected quadrangles for this control point. The approximate boundary of the watershed upstream of I30000 is outlined with a blue dashed line. As seen in the figure, most of the watershed that drains into I30000 lies between quadrangles 708 and 709. Since quadrangle 709 is closest to the center of the watershed, it is expected to have a stronger relationship than quadrangle 708 to the naturalized flow at I30000. However, quadrangle 708 is still considered in the analysis for its potential relationship to flow originating in the western portion of the watershed. Figure 3.1 Control Point I30000 and selected quadrangles. Figure 3.2 shows an example of the relationship between naturalized flow and two composite features of precipitation. The upper left graph shows monthly naturalized flow versus total monthly precipitation exponentially averaged with smoothing term 4 for the 1983–2016 period. The upper right graph is the scatter plot of the ranks, in ascending order, of the data presented in the upper left graph. The quadrangle coefficients were set to 0.06 for quadrangle 708 and 0.94 for quadrangle 709 to achieve a maximum correlation of 0.70, as calculated using the ranks shown in the upper right graph. The lower two graphs pertain to the monthly 7-day maximum of daily precipitation minus evaporation exponentially averaged with smoothing term 6. The quadrangle coefficients for this feature were set to 0.29 for quadrangle 708 and 0.71 for quadrangle 709 to achieve a maximum rank correlation of 0.66. The quadrangle weights of the remaining precipitation features were optimized in the same manner, each producing a unique set of quadrangle coefficients. D-71 DRAFT – Water Forward 2024 Appendix D 75,000 50,000 25,000 ) t f - c a ( l w o F y l h t n o M 0 0 0 0 75,000 50,000 25,000 ) t f - c a ( l w o F y l h t n o M l k n a R w o F y l h t n o M l k n a R w o F y l h t n o M 450 375 300 225 150 75 0 450 375 300 225 150 75 0 2 4 6 8 10 0 75 Monthly Precipitaiton (inches) 150 225 Monthly Precipitaiton Rank 300 375 450 1 2 Monthly 7-day Max of Precip minus Evap (inches) 3 4 0 75 225 Monthly 7-day Max of Precip minus Evap Rank 150 300 375 450 Figure 3.2 Monthly naturalized flow versus monthly precipitation features. Final Quadrangle Coefficients Many precipitation features were used to produce a range of quadrangle coefficients covering a range of temporal and event-based relationships of precipitation to flow. However, a single set of quadrangle coefficients was created to proceed with the next steps of feature selection and flow modeling. After the quadrangle coefficients for the selected composite precipitation features were optimized for rank correlation with naturalized flow, the coefficients for each composite precipitation feature were averaged. A weighted average of the coefficients at each quadrangle was calculated based on the rank correlation value. Composite precipitation features with greater correlation with naturalized flow had more influence on the final set of quadrangle coefficients. Only the coefficients belonging to composite features that had rank correlation with naturalized flow of 0.6 or greater were averaged. If less than 10 composite features met the threshold, then the coefficients of the features with the top 10 highest correlations were averaged. D-72 DRAFT – Water Forward 2024 Appendix D Figure 3.3 Control Points I4 through I1 and associated quadrangles. Figure 3.3 shows an example of 4 control points in the Highland Lakes area of the basin and the 5 quadrangles that were selected for these control points. Only 2 to 3 quadrangles were associated with any of the control points in the figure. The final set of quadrangle coefficients for these control points are shown in Table 3.1. Table 3.1 Final quadrangle coefficients for Control Points I4 through I1. Control Point Quad 609 Quad 708 Quad 709 Quad 710 Quad 809 I30000 0.11 I40000 0.78 I20000 0.16 I10000 0.22 0.89 0.69 0.11 0.15 0.64 0.25 Feature Selection D-73 DRAFT – Water Forward 2024 Appendix D As discussed previously, 830 features were created from the weather data at each quadrangle location. The features from multiple locations were combined into composite features using the quadrangle weightings associated with each control point. Next, a set of 10 features were identified from the overall set of 830 composite features to be used for modeling naturalized flow. The goal of feature selection is to create a grouping of features that are highly relevant and minimally redundant. Relevancy is measured by the strength of the feature’s relationship to naturalized flow. Redundancy is measured by the strength of the relationship between features. A limit of 10 was placed on the number of selected features so that the flow model could remain simple yet have sufficient diversity of weather inputs for modeling across low to high flow conditions. Other numbers of features were explored, but the choice of 10 produced satisfactory results while minimizing the number of low inputs. Within the total number of features, there were 416 precipitation features, 96 dry day features, 240 temperature features, 48 evaporation features, and 30 solar radiation features. Filter Algorithm A filter algorithm was used to select the top 10 features for each control point. The filter used in this study was adapted from a forward selection process proposed by Battiti (1994) and further described by May et al. (2011). The filter involves selecting an initial feature that most closely correlates to naturalized flow. Next, each candidate feature is assigned three scores: relevancy, redundancy, and partial relevancy. The filter algorithm proceeds as follows: i. ii. Initial selection. For each remaining feature: a. Candidate score = Relevancy – Redundancy + Partial Relevancy. b. Select the candidate feature with the highest score. iii. Move the candidate feature from step ii. b to the set of selected features. iv. Repeat steps ii and iii until the desired number of selected features is reached. Relevancy, redundancy, and partial relevancy scores are calculated from a rescaling of Spearman’s rank correlation. In the process proposed by Battiti (1994), a measure of dependency between variables known as mutual information was used to calculate the scores. However, formal computation of mutual information is complex, especially for continuous variables like flow and weather, and the algorithm for the feature selection being applied only requires a relative measure of the relationship strength between variables. Therefore, mutual information for this study was calculated using a simplified form that only requires the correlation coefficient ρ (Darbellay and Vajda, 1999; Tsanas et al., 2010; May et al. 2011). Eq. 4.1 1 2 ln(1 − 𝜌𝜌 2 ) 𝑅𝑅𝑀𝑀 = − Spearman rank correlation was used for ρ in this study. Although the weather features developed in this study have various degrees of nonlinear relationships with naturalized flow and with each other, the relationships are monotonic. Rank correlation is a value between −1 and 1. Equation 4.1 rescales ρ into a value of zero if ρ is zero and approaches infinity as ρ approaches 1. Using Equation 4.1, relevancy was calculated as the mutual information based on rank correlation calculated between the candidate feature and naturalized flow. Redundancy was calculated using the highest absolute value of rank correlation between the candidate feature and any of the previously selected features. Partial relevancy was calculated with the partial rank correlation between the candidate feature and naturalized flow while controlling for the relationship between all previously selected features. Partial relevancy was included as D-74 DRAFT – Water Forward 2024 Appendix D modification to the filter algorithm to reflect the gain in relationship, if any, between a candidate feature and naturalized flow when combined with the previously selected features (Tsanas et al., 2010). Initialization of the filter algorithm was modified from selecting a single feature to selecting a pair of features. Through trials, it was found that selecting a single initial feature based on the highest relevancy score always resulted in the second feature being selected based on a low redundancy and high partial relevancy score. In almost all cases, a precipitation feature is selected first, followed by a feature from the temperature or evaporation classes. These first two selections represent a pair of features with low correlation to each other, but together have a stronger relationship with naturalized flow. Initialization of the filter algorithm was changed to select two initial features using rank-based multiple linear regression analysis of naturalized flow with all precipitation features paired with all temperature, evaporation, and solar radiation features. The pair of features with the highest coefficient of determination was selected as the initial two features. The forward selection filter algorithm proceeded to select the remaining features sequentially. The filter algorithm was modified to enforce a set of rules on the number and diversity of features being selected in the top 10. Through experimentation, it was found that selecting more precipitation than non-precipitation features improved the flow modeling results. This result was achieved by limiting the non-precipitation feature selections to no more than four. Additionally, requiring at least one extreme precipitation feature likewise improved flow modeling results. The top 10 feature selections by the filter algorithm at each control point were constrained by the following rules: • No more than nine precipitation features. • At least one extreme precipitation feature. • No more than four non-precipitation features. • At least one non-precipitation feature selected from temperature, evaporation, or solar radiation (satisfied by the initialization process). The top 10 features selected were unique to each control point. However, other assemblages of features could be considered highly relevant to naturalized flow while maintaining low redundancy between the selected features. Changes to any of the previous steps could result in a different set of top 10 features. Such changes include the creation of different feature classes, application of different feature averages, use of a different metric for weighting the quadrangles to create composite features, or changing any of the rules or criteria in the selection algorithm. Examples of Selected Features Table 4.1 shows the top 10 selected features at the control points shown in Figure 3.3. The features are listed by the weather variable name followed by the averaging method below. The arithmetic moving average is denoted by AMA in the table, followed by the number of months in the average. The exponential moving average is denoted by EMA, followed by the smoothing term N. The exponential moving average is discussed in Section 2.3 of this study. In Table 4.1, the top feature listed is total monthly precipitation, with the application of an exponential moving average using a smoothing term of either 4 or 5. The top two features were selected as a pair, and the feature listed first in Table 4.1 is the member of the pair with the highest rank correlation with naturalized flow. The remaining eight features in the table are shown in the order in which they were selected by the filter algorithm. D-75 DRAFT – Water Forward 2024 Appendix D D-76 DRAFT – Water Forward 2024 Appendix D Table 4.1 Examples of the top 10 features at selected control points. I40000 I30000 I20000 I10000 Precipitation EMA, N=5 Evaporation AMA, 6 months Precipitation EMA, N=4 Evaporation EMA, N=19 Precipitation EMA, N=4 Evaporation EMA, N=21 Precipitation EMA, N=4 Minimum Temperature AMA, 11 months Dry Days of Precip-Evap Dry Days of Precip-Evap Dry Days of Precip-Evap Dry Days of Precip-Evap AMA, 2 months EMA, N=4 EMA, N=6 EMA, N=24 Evaporation AMA, 11 months Precip-Evap EMA, N=23 Precip-Evap Dry Days of Precipitation AMA, 9 months No averaging Dry Days of Precip-Evap Dry Days of Precip-Evap Dry Days of Precipitation Dry Days of Precipitation EMA, N=16 EMA, N=21 No averaging AMA, 4 months Precip-Evap > 0.5 in/day Precip-Evap Evaporation Precipitation > 2.0 in/day AMA, 13 months No averaging AMA, 11 months No averaging Daily Precip-Evap Precip-Evap Daily Precip-Evap Precip-Evap EMA, N=4 AMA, 6 months EMA, N=4 AMA, 12 months Precip-Evap > 1.0 in/day Precip-Evap > 2.0 in/day Precipitation Precipitation > 1.0 in/day AMA, 2 months AMA, 2 months AMA, 24 months No averaging Precip-Evap Evaporation Precip-Evap Precip-Evap > 2.0 in/day AMA, 8 months AMA, 13 months AMA, 5 months AMA, 3 months Daily Precip-Evap Daily Precip-Evap Precip-Evap > 2.0 in/day Precipitation EMA, N=32 EMA, N=28 AMA, 2 months AMA, 7 months Flow Modeling The framework described in this study began with assembling observed and simulated weather data and creating many features at each quadrangle location. Next, a set of weightings were optimized for maximizing the relationship between precipitation features at quadrangle locations and the observed naturalized flow at each control point. The weightings were used to create composite features that represent the weather conditions across the incremental watersheds that drain into the control point outlets. The composite features were then ranked to produce a top 10 feature selection that seeks to maximize the relationships with naturalized flow while minimizing the relationships between features. The final step in the framework is the integration of the selected composite input features into a model that produces naturalized flows. D-77 DRAFT – Water Forward 2024 Appendix D A separate flow model was created at each control point for its unique set of 10 input features. The flow models were arranged with the same connectivity within the river basin, as shown in Figure 2.3. The modeled naturalized flow outputs of each control point were used as inputs for modeling naturalized flows at downstream control points. This process created a collection of interconnected flow models across the river basin and allowed individual flow models to focus on the local naturalized flows from smaller watersheds between control points. The relationship between weather features and naturalized flow for the 1983–2016 observed record was the basis for training the flow models. The applicability of the trained flow models to future weather conditions is a key assumption for the modeling framework. It is assumed that the weather to flow relationship captured by the trained flow models for the observational period will be representative of the relationship in the future under potentially different climate conditions. The 34-year observational period contains a diverse range of flow conditions across the basin, from extreme flooding events to the multi-year drought of record for the basin. Once the flow models were trained with weather from each GCM’s historical simulation period for a best statistical fit with the observed naturalized flows, the models were then given new sequences of the weather features, which were built from each GCM’s future projection period. Flow Model Architecture The multivariate flow models in this study are in the form of neural networks, which have a wide range of information-processing applications, including hydrologic modeling (ASCE, 2000a, 2000b). The flow models consist of a single layer feed-forward neural network. Single layer refers to a network with input features connected to a single layer of activation functions that produce the output. In this study, however, there was only one activation function. Feed-forward refers to a neural network that does not create feedback within the network. In essence, each month of input from the weather features are used once to create one month of naturalized flow output. Figure 5.1 diagrams the components and processing steps of the neural network used to model naturalized flow. Scaled Input Features Feature Weights Scaled Outputs from Upstream Models of Naturalized Flow Feature 1 Feature 2 Feature 3 Feature 4 Feature 5 Feature 6 Feature 7 Feature 8 Feature 9 Feature 10 Bias Upstream Model Weights Σ Sum Softplus Function Σ Sum Model Output Scale Back to Acre-Feet Figure 5.1 Neural network architecture. D-78 DRAFT – Water Forward 2024 Appendix D All inputs, including the observed naturalized flow, are scaled into a range of approximately 0 to 1 before processing within the neural network occurs. Scaling is performed because the activation functions within a neural network are typically centered around a net input value of zero. In this study, the activation function was the Softplus function (Dugas et al., 2000). The output of the neural network was scaled back into the units of naturalized flow once the monthly value was calculated. The top 10 weather features are shown on the left side of Figure 5.1. One additional input known as a bias is included and consists of a sequence that only has the value of 1 for each month. The bias feature is used within a neural network to improve the output fitness by shifting the summation of inputs up or down along the activation function response. Training the flow model consists of adjusting the weights, which are shown as gray circles in the figure. The weights are typically small positive or negative values that serve as multipliers. The scaled inputs, multiplied by their respective weights, are summed. The summed value is the net input of the activation function. The generalized Softplus function was used by the neural network to transform the sum of the weighted inputs. This particular activation function was selected for naturalized flow modeling because it provides a nonlinear response in drier conditions, represented by zero or negative values of the summed inputs, and transitions to a linear response in wetter conditions, represented by positive values of the summed inputs. This nonlinear response is common for rainfall-runoff relationships, as seen in the upper and lower graphs on the left side of Figure 3.2. A generalized form of the Softplus function was used in this study and has the following form: 𝐵𝐵𝐵𝐵 1 𝐵𝐵 ln(𝐴𝐴 + 𝑒𝑒 𝑆𝑆𝑜𝑜𝑆𝑆𝑆𝑆𝑆𝑆𝑆𝑆𝑆𝑆𝑜𝑜(𝑥𝑥) = The sum of the weighted inputs is shown in the equation above as x. The parameters A and B are adjusted during the neural network training process. The value of A is limited to a range of 0.7 to 1, and B is limited to a range of 1 to 15. These parameters change the degree of nonlinearity in the functional response to the net inputs. Values of A less than 1 also can result in negative function outputs when the net inputs are negative. Any negative outputs are set to reset to zero. Figure 5.2 shows some examples of the generalized Softplus function for various values of A and B, which create a range of nonlinear to near-linear responses to the net input. Zero output values of the Softplus function have been set to zero. ) D-79 DRAFT – Water Forward 2024 Appendix D , t u p t u O s u p t f o S l l w o F d e z i l a r u t a N d e a c S l 2.00 1.50 1.00 0.50 0.00 -2.00 -1.50 -1.00 1.00 1.50 2.00 -0.50 0.00 Net Input of Weather Features 0.50 A = 1, B = 1 A = 0.8, B = 1 A = 0.8, B = 2 A = 0.7, B = 15 Figure 5.2 Examples of Softplus function with various scaling parameters. For any upstream control points, the modeled naturalized flow from the upstream control points was added to the output from the Softplus function. As shown in Figure 5.1, the upstream modeled naturalized flows are scaled down from acre-feet to the approximate 0 to 1 range used for naturalized flow at the control point under consideration. The model adjusts the multiplier weights of the upstream modeled naturalized flows, representing various levels of acceptance of the upstream model outputs. The multiplier weights are values between 0 and 1, where 1 represents complete acceptance of the upstream modeled naturalized flow. The multiplier weights were also limited to not exceed the channel loss factor between control points in the WAM, which is typically between 0.9 and 1. The outputs of the flow model are scaled back into units of acre-feet per month for assessment of the goodness-of-fit with the observed naturalized flow. For this study, the Kling-Gupta Efficiency (KGE) score (Gupta et al., 2009) was used as the objective function to train the model for best fit. KGE is a dimensionless value with a range of negative infinity to 1, where 1 represents perfect agreement between the modeled and observed data. KGE has the form: 2 where r is the Pearson linear correlation coefficient between modeled and observed data, α is 𝐾𝐾𝐾𝐾𝐾𝐾 = 1 − �(1 − 𝑟𝑟) the coefficient of variation of the modeled data divided by the coefficient of variation of the observed data, and β is the mean of the modeled data divided by the mean of the observed data. In this study, only the term calculated by the square root was used during the training process, which represented a score ranging from positive infinity to a perfect score of zero. This allowed for minimization rather than maximization of the objective function. 2 + (1 − 𝛽𝛽) 2 + (1 − 𝛼𝛼) The ratio of the coefficient of variation and the ratio of the means in the KGE formula are statistical measures and do not require direct comparison of individual months of flow between the model and the observed flow set. The GCM historical weather data are stochastic in nature, and consequently the naturalized flows produced by the model do not correspond to the monthly sequence of observed naturalized flows. The mismatch in monthly flows between the model and the observed set precludes the use of the Pearson linear correlation coefficient in the KGE formula above. The KGE formula was modified to replace the correlation coefficient with a D-80 DRAFT – Water Forward 2024 Appendix D measure of similarity between the probability density functions (pdfs) of the modeled and observed naturalized flow. Specifically, the empirical probability density functions of the observed and modeled flows were estimated using histograms of the natural logarithm of flows. The absolute differences in densities per histogram bin were summed using the “city block” method and subtracted from a perfect score of 1 (Cha, 2007). The new value of r based on pdf similarity in the KGE formula ranges between 1 and -1, with 1 being equal to perfect agreement between the modeled and observed flow empirical pdfs. The KGE was calculated twice: once using the actual flows in acre-feet, and once using the inverse of flows. These two KGE scores were weighted to produce a composite KGE. A small positive value was added to the inverse flows to avoid division by zero (Garcia et al., 2017). The inverse KGE score is used so that the model is trained for low flows. The KGE score for the actual flows is most sensitive to higher flows. Trials were conducted to assess the weightings of the KGE scores and their effect on model fitness. The objective function was weighted for 80% contribution from the KGE score calculated using the actual flows and 20% weighted for the KGE score calculated with the inverse flows. Model training was performed using differential evolution (Storn and Price, 1997), which is an evolutionary-based search algorithm. Neural networks, especially with simple architectures such as the case in this study, have been shown to be suitably trained using evolutionary-based algorithms (Jain and Srinivasulu, 2004; Chau, 2006; Piotrowski and Napiorkowski, 2013). Differential evolution was performed using the R programming language with the RcppDE package (Eddelbuettel, 2018). Overfitting and Generalization The input feature weights, Softplus parameters, and upstream model weights, hereafter collectively referred to as the model’s parameters, were adjusted iteratively during the training process to improve goodness-of-fit with the observed naturalized flow as measured by the objective function. The training process proceeds until no further improvement in fitness is found. Because many parameters are trained, it is possible for improvements to the objective function value to be achieved by producing model outputs that replicate random fluctuations or noise in observed flows. Improving fitness by training on the noise is known as overfitting and is more likely to occur as the number of model parameters increases. Overfitting reduces the model’s ability to generalize, which is the ability to produce accurate outputs when given new inputs that differ from those that were used to train the model (ASCE, 2000a; Hawkins, 2004). Conversely, underfitting can occur when a model is too simple or not given enough training iterations. Underfitting will also result in poor model performance when given new input data. Striking a balance between overfitting and underfitting is therefore an important consideration in model construction and training. There are many techniques available to reduce overfitting and improve generalization, which is a more common problem than underfitting in models with sufficient inputs and architecture to map the relationship between inputs and outputs. Three techniques to reduce the potential of overfitting and improve generalization were used in this study and are categorized as follows: • Favoring a lower number of input features. • Favoring models with smaller weights for the input features. • Averaging the output of many trained models. D-81 DRAFT – Water Forward 2024 Appendix D Number of Input Features Increasing the number of weather feature inputs is one possible way to improve the flow model’s performance in reproducing the observed naturalized flow. However, adding additional weather features, and therefore the number of model parameters, also increases the likelihood that the model will be overfit to noise in the weather and flow data over the observational period. Additionally, maximizing the ability to reproduce the observed naturalized flow does not necessarily indicate that the model will accurately produce flows outside of the period used for training. Reducing the number of inputs while maintaining high model performance over the training period was achieved by trial and evaluation. In this study, a low number of parameters, varying between 13 and 18 depending on the number of upstream connections, was maintained relative to the 408 observations used for training. Smaller Weights The input feature weights can potentially assume an infinite number of combinations while producing similar model outputs. Within those combinations, however, smaller weight values are desirable because they represent less complex models and reduce the chance for model overfitting to the noise in the training data. Smaller weights also are less likely to produce volatile outputs when the model is given new information outside of the training period. Penalizing larger weight values during the training process is a method to favor combinations of weights with smaller values. Two penalty functions were calculated and added to the objective function during training. The penalty functions were based on the magnitude of the input feature weights. The first penalty was equal to the sum of the absolute value of the weights and favored shrinking the smallest weight values toward zero. The second penalty was equal to the sum of the squared weights and favored shrinking the magnitude of the largest weights. Combinations of larger input feature weights produced larger penalties and thus had less chance of being selected in the iterative training process. Ensemble Averaging Training the model requires initial conditions of the model parameters. These initial conditions will affect convergence of the neural network during training and result in different sets of the final parameters and produce different outputs. Each trained model, differing by only the initial conditions, will vary in the amount of fit to the observations. By training the model many times with different initial conditions, an ensemble of model outputs can be created by averaging the individual models. Ensembling is a technique to improve generalization by reducing the variance or inconsistencies of individual months of flow outputs which may be caused by overfitting to noise in the data by any single model. The model at each control point was trained 100 times with different initial conditions of the model parameters and the results were averaged to create an ensemble time series of flows. Results This section presents model results for the historical training period and the GCM projection period. As described in Section 5.2.3, ensembles of model outputs were created from 408 D-82 DRAFT – Water Forward 2024 Appendix D months of data within the GCM historical simulation that coincide with historical period of naturalized flows at each control point. The ensemble flows at each control point were used for assessing the model’s agreement with the observed naturalized flows. The agreement was based on the models ability to reproduce the mean, coefficient of variation, and empirical pdfs of observed flows. The goal of this study was to produce projected naturalized flows at locations throughout the Colorado River Basin which can be used within Water Forward’s water availability model. The monthly flow projections cover the GCM projection period of 2015–2100 for three different future greenhouse gas emission scenarios. Five GCMs were selected, and each was paired with the three emission scenarios, creating fifteen different projections. The modeled naturalized flows for the projection periods were compared to the historical period for changes over time. Historical Period Figure 6.1 shows four metrics of the modeled versus WAM naturalized flows at all 45 control points within the Colorado River Basin. The metrics of modeled naturalized flows for each control point are shown in the y-axes, and the metrics for the WAM naturalized flows from the historical period are shown on the x-axes. The dotted black lines in Figure 6.1 are 1:1 lines and not linear regression lines. Points which fall along or near the 1:1 lines indicate better agreement between the model and WAM naturalized flow metric than points further from the lines. The mean, standard deviation, and median flow metrics were plotted with logarithmic axes so that low and high flows are visible on the same graph. Within Figure 6.1, only the mean and coefficient of variation were used to train the models. The standard deviation and median are shown for informational purposes. The distribution of points in Figure 6.1 shows there is a high degree of agreement between the modeled and the WAM naturalized flow for the mean, standard deviation, and median values at most control points for the historical period. Some control points have a lower coefficient of variation, calculated as the standard deviation divided by the mean, for the modeled flows compared to the WAM flows and are indicated by points falling below the 1:1 line. These control points are labeled in Figure 6.1 and correspond to locations with higher variability of flows relative to their mean value. These control points are also located in areas of the upper portion of the basin where flows are changing over time (Kennedy, 2017; Furnans and Kennedy, 2019). Non-stationarity of historical observed flows increases the difficulty for model training. Refer to Figures 2.2 and 2.3 for the locations of the control points and Table 2.1 for the USGS gage names. D-83 DRAFT – Water Forward 2024 Appendix D 100 100 1,000,000 100,000 10,000 1,000 1,000,000 100,000 10,000 1,000 ) t f - c a ( l w o F y l h t n o M n a e M l e d o M ) t f - c a ( l w o F y l h t n o M n a d e M i l e d o M C4,C5,C6 100 10 E4 10 1,000,000 100,000 10,000 1,000 ) t f - c a ( l w o F y l h t n o M . v e D . S l e d o M 100 100 l w o F y l h t n o M . r a V . f e o C l e d o M 6.0 5.0 4.0 3.0 2.0 1.0 0.0 1,000 10,000 100,000 WAM Mean Monthly Flow (ac-ft) 1,000,000 1,000 100,000 10,000 WAM S.Dev. Monthly Flow (ac-ft) 1,000,000 E4 E3 C6 C7 C3 B4 1,000 100 WAM Median Monthly Flow (ac-ft) 10,000 100,000 1,000,000 2.0 3.0 WAM Coef.Var. Monthly Flow 4.0 5.0 6.0 Springs 0.0 1.0 ACCESS-CM2 CNRM-CM6-1 EC-Earth3 KACE-1-0-G UKESM1-0-LL Figure 6.1 Mean, standard deviation, coefficient of variation, and median (clockwise starting from upper left) of model versus historical naturalized flow Figure 6.2 shows the measure of similarity between the empirical pdfs of the modeled and WAM naturalized flow for each control point. The similarity metric, as described in Section 5.1, is unitless and can vary between 1 and -1, with a value of 1 indicating perfect agreement. While higher values of similarity are associated with better agreement between the statistical distribution of modeled and observed flows, there are no prescribed levels of similarity that differentiate between good and poor models. Overall, the similarity metrics indicate that the models can replicate the distributions of observed flows. Figures 6.3 and 6.4 show examples of the of the empirical pdfs at the main stem Colorado River at Austin and Sand Creek near Kingsland control points to illustrate a range of flow characteristics covering large to small drainage areas, respectively. Similarity metrics are provided for each histogram. The x-axes labels in the figures indicate the upper range of the histogram bins. For Sandy Creek near Kingsland, the lowest bin covers months of zero flow. D-84 DRAFT – Water Forward 2024 Appendix D y t i r a l i i m S F D P l a c i r e p m E 1.0 0.8 0.6 0.4 0.2 0.0 y t i s n e D l a c i r e p m E 0.14 0.12 0.10 0.08 0.06 0.04 0.02 0.00 y t i s n e D l a c i r e p m E 0.14 0.12 0.10 0.08 0.06 0.04 0.02 0.00 0 0 0 0 3 A 0 0 0 0 2 A 0 0 0 0 1 A 0 0 0 0 4 B 0 0 0 0 3 B 0 0 0 0 2 B 0 0 0 0 1 B 0 0 0 0 7 C 0 0 0 0 6 C 0 0 0 0 5 C 0 0 0 0 4 C 0 0 0 0 3 C 0 0 0 0 2 C 0 0 0 0 1 C 0 0 0 0 4 D 0 0 0 0 3 D 0 0 0 0 2 D 0 0 0 0 1 D 0 0 0 0 4 E 0 0 0 0 3 E 0 0 0 0 2 E 0 0 0 0 1 E 0 0 0 0 3 F 0 0 0 0 2 F 0 0 0 0 1 F 0 0 0 0 5 G 0 0 0 0 4 G 0 0 0 0 3 G 0 0 0 0 2 G 0 0 0 0 1 G 0 0 0 0 2 H 0 0 0 0 1 H 0 0 0 0 4 I 0 0 0 0 3 I 0 0 0 0 2 I 0 0 0 0 1 I 0 0 0 0 5 J 0 0 0 0 4 J 0 0 0 0 3 J 0 0 0 0 2 J 0 0 0 0 1 J 0 0 0 0 2 K 0 0 0 0 1 K 0 3 3 0 1 I 0 3 1 0 4 C ACCESS-CM2 CNRM-CM6-1 EC-EARTH3 KACE-1-0-G UKESM1-0-LL Figure 6.2 Emperical pdf similarity between model and historical naturalized flow S = 0.80 S = 0.86 9.5 10.0 10.5 11.0 11.5 12.0 12.5 13.0 13.5 14.0 LN(flow+1) 9.5 10.0 10.5 11.0 11.5 12.0 12.5 13.0 13.5 14.0 LN(flow+1) WAM ACCESS-CM2 WAM CNRM-CM6-1 S = 0.86 S = 0.88 9.5 10.0 10.5 11.0 11.5 12.0 12.5 13.0 13.5 14.0 LN(flow+1) 9.5 10.0 10.5 11.0 11.5 12.0 12.5 13.0 13.5 14.0 LN(flow+1) WAM EC-EARTH3 WAM KACE-1-0-G y t i s n e D l a c i r e p m E 0.14 0.12 0.10 0.08 0.06 0.04 0.02 0.00 y t i s n e D l a c i r e p m E 0.14 0.12 0.10 0.08 0.06 0.04 0.02 0.00 S = 0.85 y t i s n e D l a c i r e p m E 0.14 0.12 0.10 0.08 0.06 0.04 0.02 0.00 9.5 10.0 10.5 11.0 11.5 12.0 12.5 13.0 13.5 14.0 LN(flow+1) WAM UKESM1-0-LL Figure 6.3 Empirical pdfs of modeled and WAM observed natural log flows at control point I10000, Colorado River at Austin D-85 DRAFT – Water Forward 2024 Appendix D y t i s n e D l a c i r e p m E 0.14 0.12 0.10 0.08 0.06 0.04 0.02 0.00 y t i s n e D l a c i r e p m E 0.14 0.12 0.10 0.08 0.06 0.04 0.02 0.00 y t i s n e D l a c i r e p m E 0.14 0.12 0.10 0.08 0.06 0.04 0.02 0.00 y t i s n e D l a c i r e p m E 0.14 0.12 0.10 0.08 0.06 0.04 0.02 0.00 S = 0.84 S = 0.82 0.6 1.7 2.8 3.9 7.2 8.3 9.4 10.5 0.6 1.7 2.8 3.9 7.2 8.3 9.4 10.5 6.1 5.0 LN(flow+1) 6.1 5.0 LN(flow+1) WAM ACCESS-CM2 WAM CNRM-CM6-1 S = 0.84 S = 0.79 0.6 1.7 2.8 3.9 7.2 8.3 9.4 10.5 0.6 1.7 2.8 3.9 7.2 8.3 9.4 10.5 6.1 5.0 LN(flow+1) WAM EC-EARTH3 WAM KACE-1-0-G 6.1 5.0 LN(flow+1) y t i s n e D l a c i r e p m E 0.14 0.12 0.10 0.08 0.06 0.04 0.02 0.00 S = 0.82 0.6 1.7 2.8 3.9 7.2 8.3 9.4 10.5 6.1 5.0 LN(flow+1) WAM UKESM1-0-LL Figure 6.4 Empirical pdfs of modeled and WAM observed natural log flows at control point I30000, Sandy Creek near Kingsland GCM Projection Period Each GCM has a projection period that spans 2015–2100. During the projection periods, the climatological conditions diverge over time from the GCM historical simulation period according to the level of greenhouse gas emissions. The different greenhouse gas emission scenarios are denoted by the Shared Socioeconomic Pathway (SSP) naming convention (Details in Task 3 memo). Greenhouse gas emissions are highest in SSP5-8.5, and SSP2-4.5 represents higher D-86 DRAFT – Water Forward 2024 Appendix D emissions than SSP1-2.6. Temperature increases and changes to the distribution of precipitation occur across the basin and across time according to the level of emission. Figure 6.5 shows the rolling mean of monthly flows over a 30-year period per GCM and per SSP at the Colorado River at Austin. A long averaging period was selected to smooth the variability of monthly flows and illustrate the trends across time. The horizontal dashed line in each graph indicates the mean observed naturalized flow for the 1983-2016 historical period used to train the models. The rolling means shown in the graphs begin in 1980 and cover model flows generated from GCM weather inputs prior to the historical training period. Many of the 30-year rolling mean traces over the future projection period fall near or below the horizontal dashed line and indicate the tendency for flow projections to trend lower than measured in the recent historical period. Figure 6.6 provides the same set of graphs for Sandy Creek near Kingsland. The trends over the projection period at the small watershed represented by Sandy Creek are very similar to those for the main stem of the Colorado River at Austin. ACCESS-CM2 CNRM-CM6-1 250,000 200,000 150,000 100,000 50,000 0 1980 250,000 200,000 150,000 100,000 50,000 0 1980 ) t f - c a ( l w o F M C G n a e M r a e y - 0 3 ) t f - c a ( l w o F M C G n a e M r a e y - 0 3 250,000 200,000 150,000 100,000 50,000 0 1980 250,000 200,000 150,000 100,000 50,000 0 1980 ) t f - c a ( l w o F M C G n a e M r a e y - 0 3 ) t f - c a ( l w o F M C G n a e M r a e y - 0 3 2000 2020 2040 2060 2080 2100 2000 2020 2040 2060 2080 2100 SSP1-2.6 SSP2-4.5 SSP5-8.5 SSP1-2.6 SSP2-4.5 SSP5-8.5 EC-Earth3 KACE-1-0-G 2000 2020 2040 2060 2080 2100 2000 2020 2040 2060 2080 2100 SSP1-2.6 SSP2-4.5 SSP5-8.5 SSP1-2.6 SSP2-4.5 SSP5-8.5 UKESM1-0-LL 250,000 200,000 150,000 100,000 50,000 ) t f - c a ( l w o F M C G n a e M r a e y - 0 3 0 1980 2000 2020 2040 2060 2080 2100 SSP1-2.6 SSP2-4.5 SSP5-8.5 Figure 6.5 Rolling means of monthly flow for the Colorado River at Austin D-87 8,000 6,000 4,000 2,000 8,000 6,000 4,000 2,000 ) t f - c a ( l w o F M C G n a e M r a e y - 0 3 ) t f - c a ( l w o F M C G n a e M r a e y - 0 3 0 1980 0 1980 DRAFT – Water Forward 2024 Appendix D ACCESS-CM2 CNRM-CM6-1 8,000 6,000 4,000 2,000 8,000 6,000 4,000 2,000 ) t f - c a ( l w o F M C G n a e M r a e y - 0 3 ) t f - c a ( l w o F M C G n a e M r a e y - 0 3 0 1980 0 1980 2000 2020 2040 2060 2080 2100 2000 2020 2040 2060 2080 2100 SSP1-2.6 SSP2-4.5 SSP5-8.5 SSP1-2.6 SSP2-4.5 SSP5-8.5 EC-Earth3 KACE-1-0-G 2000 2020 2040 2060 2080 2100 2000 2020 2040 2060 2080 2100 SSP1-2.6 SSP2-4.5 SSP5-8.5 SSP1-2.6 SSP2-4.5 SSP5-8.5 UKESM1-0-LL 8,000 6,000 4,000 2,000 ) t f - c a ( l w o F M C G n a e M r a e y - 0 3 0 1980 2000 2020 2040 2060 2080 2100 SSP1-2.6 SSP2-4.5 SSP5-8.5 Figure 6.6 Rolling means of monthly flow for Sandy Creek near Kingsland Figures 6.7 and 6.8 show box-whisker comparisons by combining the five sets of flows derived from GCM weather inputs and separating them by SSP and non-overlapping 20-year windows that span the projection period from 2020 through 2100. The historical observed WAM naturalized flows and the combined set of five GCM derived flows for the historical period are replicated on each graph for comparative purposes. The box-whisker graphs show the mean monthly flow denoted with an X. The body of the box indicates the lower quartile (25th percentile) and upper quartile (75th percentile) of monthly flow along the top bottom and top edges of the box, respectively. The distance between the upper and lower quartiles is known as the innerquartile range (IQR) and represents the range where the middle 50% of the data are located. The line through the box indicates the median or 50th D-88 DRAFT – Water Forward 2024 Appendix D percentile flow value. The length of the upper whisker is equal to the lesser of the maximum value or a distance of 1.5 times the IQR above the upper quartile. Similarly, the length of the lower whisker is equal to the greater of the minimum value of a distance of 1.5 times the IQR below the lower quartile. Flows outside of the whiskers, if any, are considered outliers. The outlier values are not shown for the purposes of maintaining legible box sizes. Figures 6.7 and 6.8 illustrate a general trend towards lower flows with increasing greenhouse emissions as the projection period advances towards the end of the century. This is indicated by the declining body of the boxes and the heights of the whiskers. The mean flow is more sensitive to a small number of random high flow events during the 20-year periods as seen by the placement of the X in the graphs. SSPs 2021-2040 SSPs 2041-2060 SSPs 2061-2080 SSPs 2081-2100 Figure 6.7 Box-whisker plots of combined GCM monthly flows at Control Point I10000, Colorado River at Austin D-89 DRAFT – Water Forward 2024 Appendix D SSPs 2021-2040 SSPs 2041-2060 SSPs 2061-2080 SSPs 2081-2100 Figure 6.8 Box-whisker plots of combined GCM monthly flows at Control Point I30000, Sandy Creek near Kingsland Figures 6.5 through 6.8 provide examples that characterize the trend in flow projections for two control points with respect to emission scenarios and time. Control Point I30000 is a tributary with approximately 350 square miles of drainage area, whereas Control Point I10000 resides on the main stem of the Colorado River with a drainage area of approximately 27,600 square miles. The changes in flow distribution for the other control points, both at small tributaries and at large main stem locations, were found to exhibit similar behavior. The flow distributions across the basin trend towards mostly lower flows with increasing greenhouse emissions and time. Concurrently, a small number of high flow events increase in magnitude as compared with the WAM naturalized flows. Figures 6.9 and 6.10 show a high flow metric, the 99th percentile of monthly flows, for Control Points I10000 and I30000. The graphs in each are arranged with the lower greenhouse gas emission scenario, SSP1-2.6, at the top and the highest emission scenario, SSP5-8.5, at the bottom of the figure. The left side of each graph provides the 99th percentile flow for the historical period. Columns in the historical period include the WAM naturalized flows and the modeled naturalized flows as trained with weather inputs derived from the five GCMs. The columns in the historical period are repeated in each graph for comparative purposes with the projection periods. The four groupings of columns to the right of the historical period are 20-year non-overlapping periods from 2020 through 2100. The 99th percentile was selected to illustrate the potential for extreme rain events in the projection period to produce high flow events that exceed the historical observations. Several events exceeding the historical observations are shown in the figures at both locations. These D-90 DRAFT – Water Forward 2024 Appendix D figures do not show a trend in the frequency or magnitude of higher flow events with regard to SSP or period of time in the projections, other than there generally is potential for future rainfall and flow events to exceed those in the historical record under global warming. Previous studies also suggest higher possibilities of more intense heavy rainfall events in the future over Texas (WF18; Nielsen-Gammon et al., 2021). Historical Period 2021-2040 2041-2060 2061-2080 2081-2100 asdf SSP1-2.6 asdf SSP2-4.5 SSP5-8.5 M A W 2 M C - S S E C C A 1 - 6 M C - M R N C 3 h t r a E - C E G - 0 - 1 - E C A K L L - 0 - 1 M S E K U 2 M C - S S E C C A 1 - 6 M C - M R N C 3 h t r a E - C E G - 0 - 1 - E C A K L L - 0 - 1 M S E K U 2 M C - S S E C C A 1 - 6 M C - M R N C 3 h t r a E - C E G - 0 - 1 - E C A K L L - 0 - 1 M S E K U 2 M C - S S E C C A 1 - 6 M C - M R N C 3 h t r a E - C E G - 0 - 1 - E C A K L L - 0 - 1 M S E K U 2 M C - S S E C C A 1 - 6 M C - M R N C 3 h t r a E - C E G - 0 - 1 - E C A K L L - 0 - 1 M S E K U Historical Period 2021-2040 2041-2060 2061-2080 2081-2100 M A W 2 M C - S S E C C A 1 - 6 M C - M R N C 3 h t r a E - C E G - 0 - 1 - E C A K L L - 0 - 1 M S E K U 2 M C - S S E C C A 1 - 6 M C - M R N C 3 h t r a E - C E G - 0 - 1 - E C A K L L - 0 - 1 M S E K U 2 M C - S S E C C A 1 - 6 M C - M R N C 3 h t r a E - C E G - 0 - 1 - E C A K L L - 0 - 1 M S E K U 2 M C - S S E C C A 1 - 6 M C - M R N C 3 h t r a E - C E G - 0 - 1 - E C A K L L - 0 - 1 M S E K U 2 M C - S S E C C A 1 - 6 M C - M R N C 3 h t r a E - C E G - 0 - 1 - E C A K L L - 0 - 1 M S E K U Historical Period 2021-2040 2041-2060 2061-2080 2081-2100 asdf M A W 2 M C - S S E C C A 1 - 6 M C - M R N C 3 h t r a E - C E G - 0 - 1 - E C A K L L - 0 - 1 M S E K U 2 M C - S S E C C A 1 - 6 M C - M R N C 3 h t r a E - C E G - 0 - 1 - E C A K L L - 0 - 1 M S E K U 2 M C - S S E C C A 1 - 6 M C - M R N C 3 h t r a E - C E G - 0 - 1 - E C A K L L - 0 - 1 M S E K U 2 M C - S S E C C A 1 - 6 M C - M R N C 3 h t r a E - C E G - 0 - 1 - E C A K L L - 0 - 1 M S E K U 2 M C - S S E C C A 1 - 6 M C - M R N C 3 h t r a E - C E G - 0 - 1 - E C A K L L - 0 - 1 M S E K U Figure 6.9 Ninety-ninth percentile flows at Control Point I10000, Colorado River at Austin 3.5 3.0 2.5 2.0 1.5 1.0 0.5 0.0 3.5 3.0 2.5 2.0 1.5 1.0 0.5 0.0 3.5 3.0 2.5 2.0 1.5 1.0 0.5 0.0 ) t f - c a M ( l w o F y l h t n o M e l i % 9 9 ) t f - c a M ( l w o F y l h t n o M e l i % 9 9 ) t f - c a M ( l w o F y l h t n o M e l i % 9 9 D-91 DRAFT – Water Forward 2024 Appendix D 100 80 60 40 20 0 100 80 60 40 20 0 100 80 60 40 20 0 ) t f - c a M ( l w o F y l h t n o M e l i % 9 9 ) t f - c a M ( l w o F y l h t n o M e l i % 9 9 ) t f - c a M ( l w o F y l h t n o M e l i % 9 9 Historical Period 2021-2040 2041-2060 2061-2080 2081-2100 asdf M A W 2 M C - S S E C C A 1 - 6 M C - M R N C 3 h t r a E - C E G - 0 - 1 - E C A K L L - 0 - 1 M S E K U 2 M C - S S E C C A 1 - 6 M C - M R N C 3 h t r a E - C E G - 0 - 1 - E C A K L L - 0 - 1 M S E K U 2 M C - S S E C C A 1 - 6 M C - M R N C 3 h t r a E - C E G - 0 - 1 - E C A K L L - 0 - 1 M S E K U 2 M C - S S E C C A 1 - 6 M C - M R N C 3 h t r a E - C E G - 0 - 1 - E C A K L L - 0 - 1 M S E K U 2 M C - S S E C C A 1 - 6 M C - M R N C 3 h t r a E - C E G - 0 - 1 - E C A K L L - 0 - 1 M S E K U Historical Period 2021-2040 2041-2060 2061-2080 2081-2100 M A W 2 M C - S S E C C A 1 - 6 M C - M R N C 3 h t r a E - C E G - 0 - 1 - E C A K L L - 0 - 1 M S E K U 2 M C - S S E C C A 1 - 6 M C - M R N C 3 h t r a E - C E G - 0 - 1 - E C A K L L - 0 - 1 M S E K U 2 M C - S S E C C A 1 - 6 M C - M R N C 3 h t r a E - C E G - 0 - 1 - E C A K L L - 0 - 1 M S E K U 2 M C - S S E C C A 1 - 6 M C - M R N C 3 h t r a E - C E G - 0 - 1 - E C A K L L - 0 - 1 M S E K U 2 M C - S S E C C A 1 - 6 M C - M R N C 3 h t r a E - C E G - 0 - 1 - E C A K L L - 0 - 1 M S E K U Historical Period 2021-2040 2041-2060 2061-2080 2081-2100 asdf SSP1-2.6 asdf SSP2-4.5 SSP5-8.5 M A W 2 M C - S S E C C A 1 - 6 M C - M R N C 3 h t r a E - C E G - 0 - 1 - E C A K L L - 0 - 1 M S E K U 2 M C - S S E C C A 1 - 6 M C - M R N C 3 h t r a E - C E G - 0 - 1 - E C A K L L - 0 - 1 M S E K U 2 M C - S S E C C A 1 - 6 M C - M R N C 3 h t r a E - C E G - 0 - 1 - E C A K L L - 0 - 1 M S E K U 2 M C - S S E C C A 1 - 6 M C - M R N C 3 h t r a E - C E G - 0 - 1 - E C A K L L - 0 - 1 M S E K U 2 M C - S S E C C A 1 - 6 M C - M R N C 3 h t r a E - C E G - 0 - 1 - E C A K L L - 0 - 1 M S E K U Figure 6.10 Ninety-ninth percentile flows at Control Point I30000, Sandy Creek near Kingsland D-92 DRAFT – Water Forward 2024 Appendix D Conclusion In this task, multivariate hydrological models using a neural network architecture were developed for locations across the Colorado River Basin and the naturalized flow outputs of these models derived from GCM simulations of precipitation, temperature, and evaporation data were analyzed. The naturalized flows from the models for the GCM historical period were compared to the observed naturalized flows. High degree of agreement was achieved between the modeled and the WAM naturalized flows for statistical measures of flow at most control points for the observational period. Conventional measures of fit for individual months, such as correlation coefficient or mean squared error, between the modeled and observed flows was not possible because the GCM simulations of weather are not synchronized to the timing of historical observations of weather. However, the models were able to replicate the observed flow distributions as measured by empirical probability density functions. The future flow projections show an overall drying trend across SSPs and time with increased frequency of lower flows. Increases in the number of dry days as well as rising temperature projected over this region explains this increased probability of low flow events. On the other hand, the magnitude of extreme high flows may increase as indicated by the potential for flood events exceeding those in the observational record. This is consistent with the response to increase in the frequency and magnitude of heavy rainfall events projected over the CRB. In an alternative approach, using a water balance based hydrological model, streamflows are produced at the gauges covering the CRB utilizing monthly runoff data from the GCMs. Comparison between the flow projections derived from these two approaches provided in the Appendix. The multivariate approach will be used for the update to the Water Forward Plan, but more research is planned to investigate the feasibility and accuracy of the physically based approach. D-93 DRAFT – Water Forward 2024 Appendix D References AKHTER, M. (2017). Application of ANN for the Hydrological Modeling. International Journal for Research in Applied Science & Engineering Technology, v. 5, n. 7, p. 203-213 Allan, R.P., Soden, B.J. (2008) Atmospheric warming and the amplification of precipitation extremes. Science 321(5895):1481–1484 Asati, S., & Rathore, S. (2012). Comparative study of stream flow prediction model. International Journal of sciences ASCE Task Committee on Application of Artificial Neural Networks in Hydrology (2000a). Artificial Neural Networks in Hydrology. I: Preliminary Concepts. Journal of Hydrologic Engineering. Vol. 5, Issue 2. pp. 115-123. doi:10.1061/(ASCE)1084-0699(2000)5:2(115) ASCE Task Committee on Application of Artificial Neural Networks in Hydrology (2000b). Artificial Neural Networks in Hydrology. II: Hydrologic Applications. Journal of Hydrologic Engineering. Vol. 5, Issue 2. pp. 124-137. doi: 10.1061/(ASCE)1084-0699(2000)5:2(124) Austin Water (2018). A Water Plan for the Next 100 year. https://austintexas.gov/sites/default/files/files/Water/WaterForward/Water_Forward_Plan_Re port_-_A_Water_Plan_for_the_Next_100_Years.pdf Banerjee, P., R. K. Prasad, and V. S. Singh (2009), Forecasting of groundwater level in hard rock region using artificial neural network, Environ. Geol., 58(6), 1239–1246, doi:10.1007/s00254-008-1619-z. Battiti, R. (1994). Using mutual information for selecting features in supervised neural net learning. IEEE Transactions on Neural Networks, Vol 5, Issue 4, pp. 537-550. doi:10.1109/72.298224 Biotechnology and Pharma Research, 1, 139-151 Cha, S-H. (2007). Comprehensive Survey on Distance/Similarity Measures between Probability Density Functions. International Journal of Mathematical Models and Methods in Applied Sciences. Vol. 1, Issue 4, pp. 300-307. Chau, K.W. (2006). Particle swarm optimization training algorithm for ANNs in stage prediction of Shing Mun River. Journal of Hydrology. Vol. 329. pp. 363-367. doi: 10.1016/j.jhydrol.2006.02.025 Daly, C., Halbleib, M., Smith, J.I., Gibson, W.P., Doggett, M.K., Taylor, G.H., Curtis, J., Pasteris, P.P. (2008). Physiographically sensitive mapping of climatological temperature and precipitation across the conterminous United States. International Journal of Climatology. Vol. 28, Issue 15, pp. 2031–2064. doi:10.1002/joc.1688 Darbellay, G.A., Vajda, I. (1999). Estimation of the Information by an Adaptive Partitioning of the Observation Space. IEEE Transactions on Information Theory, Vol 45, pp. 1315-1321. doi:10.1109/18.761290 Das Bhowmik R, Seo SB, Sahoo S (2018) Streamflow simulation using Bayesian regression with multivariate linear spline to estimate future changes. Water 10:875–893. https://doi.org/10.3390/w10070875 D-94 DRAFT – Water Forward 2024 Appendix D Devia, G. K., Ganasri, B. P., & Dwarakish, G. S. (2015). A review on hydrological models. Aquatic Procedia, 4, 1001– 1007. https://doi.org/10.1016/j.aqpro.2015.02.126 Dugas, C., Bengio, Y., Bélisle, F., Nadeau, C., Garcia, R. (2000). Incorporating second-order functional knowledge for better option pricing. Advances in Neural Information Processing Systems 13. Proceedings of the 2000 Neural Information Processing Systems Conference. doi:10.5555/3008751.3008817 Eddelbuettel, D. (2018). RcppDE: Global Optimization by Differential Evolution in C++. R package version 0.1.6 Furnans, J., Kennedy, K. (2019). Evaluation of rainfall-runoff trends in the upper Colorado River Basin. Texas Water Development Board. Contract No. 1800012283. Garcia, F., Folton, N., Oudin, L. (2017). Which objective function to calibrate rainfall–runoff models for low-flow index simulations? Hydrological Sciences Journal. Vol 62, Issue 7, pp. 1149-1166. doi:10.1080/02626667.2017.1308511 Gupta, H. V., Kling, H., Yilmaz, K. K., Martinez, G. F. (2009). Decomposition of the mean squared error and NSE performance criteria: Implications for improving hydrological modelling. Journal of Hydrology, Vol. 377, Issues 1-2, pp. 80-91. doi:10.1016/j.jhydrol.2009.08.003 Hargreaves, G.H., Samani, Z.A. (1982). Estimating Potential Evapotranspiration, Journal of the Irrigation and Drainage Division, Vol. 108, Issue 3, pp. 225-230. doi: 10.1061/JRCEA4.0001390 Hargreaves, G.H., Samani, Z.A. (1985). Reference Crop Evapotranspiration from Temperature, Applied Engineering in Agriculture, Vol 1, No. 2, pp. 96-99. doi: 10.13031/2013.26773 Hawkins, D.M. (2004). The problem of overfitting. Journal of Chemical Information and Computer Sciences. Vol. 44, No. 1. pp. 1-12. doi:10.1021/ci0342472 Held, I. M. & Soden, B. J. (2006), Robust responses of the hydrological cycle to global warming. J. Climate, 19, 5686–5699. Huntington, T. G. (2006), Evidence for intensification of the global water cycle: review and synthesis, Journal of Hydrology, 319(1), 83–95. Islam Z. 2011 A Review on Physically Based Hydrologic Modeling. University of Alberta, Edmonton, AB, Canada. doi:10.13140/2.1.4544.5924. Jain, A., Srinivasulu, S. (2004). Development of effective and efficient rainfall–runoff models using integration of deterministic, real-coded genetic algorithms and artificial neural network techniques. Water Resources Research. Vol 40. Issue 4. doi:W04302APR222004 Kennedy, K. (2017). Evaluation of rainfall/runoff patterns in the upper Colorado River Basin. Texas Water Development Board. Contract No. 1600012011. Kisi, O. (2004), River flow modeling using artificial neural networks, J.Hydrol. Eng., 9(1), 60–63, doi:10.1061/(ASCE)1084-0699(2004)9:1(60). Maier, H.R. and Dandy, G.C. (2000) Neural Networks for the Prediction and Forecasting of Water Resources Variables: A Review of Modelling Issues and Applications. Environmental D-95 DRAFT – Water Forward 2024 Appendix D Modelling & Software, 15, 101-124. https://doi.org/10.1016/S1364-8152(99)00007-9 May, R., Dandy, G., Maier, H. (2011). Review of input variable selection methods for artificial neural networks. Artificial neural networks-methodological advances and biomedical applications, 10(1), pp. 19-45. doi:10.5772/16004 Moradkhani, H. and Sorooshian, S. (2008). General review of rainfall-runoff modeling: model calibration, data assimilation, and uncertainty analysis, in Hydrological Modeling and Water Cycle, Coupling of the Atmospheric and Hydrological Models, Springer, Water Science and Technology Library, 63, 1–24, https://doi.org/10.1007/978-3-540-77843-1_1 Ni L. Wang D. Singh V. P. Wu J. Wang Y. Tao Y. Zhang J. 2019 Streamflow and rainfall forecasting by two long short-term memory-based models. Journal of Hydrology 583, 124296 Nielsen-Gammon, J., S. Holman, A. Buley, S. Jorgensen, J. Escobedo, C. Ott, J. Dedrick, and A. Van Fleet, 2021: Assessment of Historic and Future Trends of Extreme Weather in Texas, 1900-2036: 2021 Update. Document OSC-202101, Office of the State Climatologist, Texas A&M University, College Station, 44 pp. Piotrowski, A., Napiorkowski, J. (2013). A comparison of methods to avoid overfitting in neural networks training in the case of catchment runoff modelling. Journal of Hydrology. Vol. 476. pp. 97-111. doi:10.1016/j.jhydrol.2012.10.019 Sahoo, G. B., and C. Ray (2006), Flow forecasting for a Hawaii stream using rating curves and neural networks, J. Hydrol., 317, 63–80, doi:10.1016/j.jhydrol.2005.05.008 Sethi, R. R., A. Kumar, S. P. Sharma, and H. C. Verma (2010), Prediction of water table depth in a hard rock basin by using artificial neural network, Int. J. Water Resour. Environ. Eng., 2(4), 95–102. Sheffield, J. and Wood, E.F. (2008) Projected Changes in Drought Occurrence under Future Global Warming from Multi-Model, Multi-Scenario, IPCC AR4 Simulations. Climate Dynamics, 31, 79-105. Slater, L. J., & Villarini, G. (2018). Enhancing the predictability of seasonal streamflow with a statistical-dynamical approach. Geophysical Research Letters, 45, 6504–6513. Storn, R., Price, K. (1997). Differential evolution – a simple and efficient heuristic for global optimization over continuous spaces. Journal of Global Optimization. Vol 11. pp. 341-359. doi:10.1023/A:1008202821328 TCEQ (2022). Water Availability Models – Texas Commission on Environmental Quality. https://www.tceq.texas.gov/permitting/water_rights/wr_technical-resources/wam.html Texas’ Extreme Weather, txH2O, TWRI, Fall 2016: https://twri.tamu.edu/publications/txh2o/2016/fall-2016/ Trenberth, K.E., Dai, A., Rasmussen, R.M., Parsons, D.B. (2003). The changing character of precipitation. Bull. Am. Meteorol. Soc. 84, 1205–1218. Tsanas, A., Little, M. A., & McSharry, P. E. (2010). A simple filter benchmark for feature selection. Journal of Machine Learning Research, 1(1-24). TWDB (2022). The Texas Water Development Board Lake Evaporation and Precipitation dataset. https://waterdatafortexas.org/lake-evaporation-rainfall D-96 DRAFT – Water Forward 2024 Appendix D Wegayehu, E.B., & Muluneh, F.B. (2021). "Multivariate Streamflow Simulation Using Hybrid Deep Learning Models", Computational Intelligence and Neuroscience, vol. 2021, Article ID 5172658, 16 pages. https://doi.org/10.1155/2021/5172658 Wurbs, R.A., (2021). Water Rights Analysis Package (WRAP) modeling system reference manual. 13th Edition. College Station, Texas: Texas Water Resources Institute. Technical Report 256. 273 p. Yuan X. Chen C. Lei X. Yuan Y. Adnan R. M. 2018 Monthly runoff forecasting based on LSTM– ALO model. Stochastic Environmental Research and Risk Assessment 32 (8), 2199–2212. D-97 DRAFT – Water Forward 2024 Appendix D Task 5: Climate-Adjusted Hydrology for Water Availability Modeling Prepared by: Hoffpauir Consulting Summary This memo documents the methodology employed to modify the existing Water Availability Model (WAM) historical hydrologic record, which includes sequences of naturalized flows and reservoir net evaporation at pertinent locations throughout the Colorado River Basin (CRB), to reflect the hydrology of various future climate scenarios. The climate scenarios are based on five Global Climate Models (GCMs) and three greenhouse gas emission scenarios, known as Shared Socioeconomic Pathways (SSPs), as described in the Task 3 memo. Seven future time horizons are considered from 2030 to the end of the GCM projections in 2100. The goal of Task 5 is to adjust the CRB WAM’s historical hydrologic record in a manner that maintains the same pattern of wet and dry months as observed in the original data, but accounts for the range of flows and net evaporation amounts in the future projection periods of the climate scenarios. The methods for developing stream flow projections using precipitation and temperature outputs from the GCMs, as well as the evaporation time series derived from GCM outputs, are detailed in the Task 4 memo. Analysis of the modified historical hydrologic records are consistent with the findings of the trend analysis of the Task 3 memo and the flow modeling of the Task 4 memo, which indicate a trend towards hotter and more frequent drier hydrologic conditions across the basin, but with a potential for higher peak flows during short-term extreme precipitation events. Adjustment of the hydrologic records according to individual GCM-SSP-time horizon groupings reflects various levels of change relative to historical conditions. An ensemble was also created for each time horizon by averaging the results of the five GCM-derived hydrologic adjustments. In total, 126 sets of adjusted hydrologic input files were created for the CRB WAM. The adjusted hydrologic inputs files, as well as the unadjusted historical hydrologic input files, will be used in water availability modeling analyses for Water Forward 2024 (WF24) and provide a diversity of conditions for evaluating water management strategies. D-98 DRAFT – Water Forward 2024 Appendix D EVA FAD FLO GCM MFM SSP TCEQ TWDB USGS WAM WF24 WRAP List of Acronyms CRB Colorado River Basin Texas Commission on Environmental Quality WAM Net Evaporation File WAM Spring Flow Adjustments File WAM Naturalized Flow File Global Climate Model Multivariate Flow Model (Task 4) Shared Socioeconomic Pathways Texas Water Development Board United States Geological Survey Water Availability Model Water Forward 2023 Water Rights Analysis Package D-99 DRAFT – Water Forward 2024 Appendix D Background The hydrologic input data of the CRB WAM consists of four sets of monthly time series organized into three files: the FLO file, which contains naturalized stream flows at 43 control points; the FAD file, which provides adjustments to naturalized flows for spring discharges at 13 control points; and the EVA file, which accounts for evaporation minus precipitation, or net evaporation, at 48 control points. All hydrologic data are provided as input to the WAM using monthly time steps. The FLO file contains naturalized flow data for 43 locations within the CRB and 2 additional locations for coastal streams that are not connected to the Colorado River and are not part of this study. The FAD file addresses adjustments to naturalized flows at 13 locations, representing 14 springs. Of these, only 2 springs, Barton Springs and Dove Creek Spring, have United States Geological Survey (USGS) gage measurements and were modeled in the Multivariate Flow Model (MFM) described in Task 4. The discharges from the other 12 springs (11 control points) are derived from linear regression equations based on the flow at the two gaged springs. The EVA file contains net evaporation data and is calculated at the locations of major reservoirs. This calculation involves weighting measurements of evaporation and precipitation from nearby quadrangles in the Texas Water Development Board (TWDB) data set. Precipitation is subtracted from evaporation to create a net evaporation depth in units of feet per month. The WAM uses net evaporation, in conjunction with a surface area and volume relationship, to calculate the net loss or gain from the reservoir surface area. Net evaporation is negative when precipitation exceeds evaporation in a month. Methods and Data This section presents the methods used for generating the hydrologic projections for the CRB WAM, along with the data sources and preprocessing steps involved. The process utilizes the WAM’s historical hydrologic time series and projections of hydrology derived from GCMs and the MFM described in Task 4. The adjustment process culminates in sets of WAM hydrologic input files that reflect future conditions in the basin under the various climate scenarios. Data The data used in this study consist of monthly historical hydrologic time series of stream flow at 43 control points, spring flow at two control points, as well as lake evaporation and precipitation time series at 48 control points within CRB WAM. The historical period of record spans from 1940 to 2022. The official historical period of record included with the Texas Commission on Environmental Quality (TCEQ) CRB WAM is from 1940 to 2016. Hydrologic data for the period of 2017-2022 were developed using the same methods described in the Task 4 memo, which employs observed precipitation, temperature, and evaporation data for this period. Monthly time series of potential future stream flow, spring flow, lake evaporation, and precipitation at the same control points were developed using the methods described in the Task 4 memo for each GCM and SSP pairing, covering 2015 to 2100 projection period. The method for adjusting the historical hydrologic record is organized into three general steps: • selecting future periods in the climate scenario projections, D-100 DRAFT – Water Forward 2024 Appendix D • adjusting the historical hydrologic sequences of flow, evaporation, and precipitation to • reflect the range of hydrology in the future periods, and formatting the adjusted hydrologic sequences according to the conventions used in the WAM input files. Future Periods The next step in the methodology involves the selection of future periods from the hydrologic projections. These future periods are groupings of 31-years centered on seven decadal time horizons: 2030, 2040, 2050, 2060, 2070, 2080, and 2100. The time horizons correspond to decadal water demand projections used in Water Forward for evaluation of water availability, needs, and water management strategies. Due to the GCM projections ending in 2100, it is not possible to center a 31-year period on 2100. Instead, the last 31 years of GCM projection data are used for this time horizon. WF24 will evaluate water management strategies for the year 2120 using the farthest GCM projection, referred to as the 2100 time horizon in this memo. The choice of 31-year periods is based on the common use of 30-year periods used to evaluate periodic averages of climate variables, referred to as climate normals (WMO, 2007). The choice of 31-year periods in this study is intended to provide longer-term representative samples of the flow and weather variables and to reduce the influence of short-term variations. Quantile Mapping Quantile mapping is a bias correction technique that adjusts values in an input or control data set based on the corresponding values in a target or simulated data set that share the same probability or relative position. The quantile mapping method employed in this study is the empirical cumulative distribution approach. Values in the control and simulated data set that fall between percentiles are approximated by linear interpolation. Any values outside of the percentiles, due to differing lengths of the control and simulated data sets, are adjusted using the last available percentile (Boe et al., 2007). This process is performed on a per month, per control point, per GCM, and per SSP basis. An additional output is generated as the average across all five GCM results, referred to as an ensemble, for each 31-year time horizon. The historical inputs for quantile mapping include: • Naturalized Flow at 43 control points, consisting of the TCEQ WAM naturalized flows for 1940-2016 and the Multivariate Flow Model for 2017-2021. • Spring discharge at two control points, using USGS gage flow data, with extension using the MFM outputs. The two control points correspond to Barton Springs and Dove Creek Springs. • Lake evaporation, comprising the Texas Water Development Board (TWDB) quadrangle data for 1954-2021, and the TWDB 1940-1953 bias-corrected lake evaporation data. The 1940-1953 data are unpublished due to inconsistencies in data collection methods compared to the 1954-present data. The 1940-1953 data were bias-corrected using a mean and standard deviation correction technique to align with the 1954-present data set. • Precipitation, consisting of the TWDB 1940-2021 quadrangle data. At the time of the work on the MFM, inflows in 2022 for the two water supply reservoirs in the Lower Colorado River basin, lakes Buchanan and Travis, were lower than recorded in 2011. D-101 DRAFT – Water Forward 2024 Appendix D The weather data were not available for the whole year to extend the historical hydrology for the 2022 calendar year. Therefore, historical flows, evaporation, and precipitation for year 2011 were appended to the end historical hydrologic files as a proxy for 2022. The projection inputs for quantile mapping include the following data which are extracted for the 31-year periods centered on the future time horizons as previously discussed: • stream flow • spring flow • evaporation, and • precipitation data. The future period data were derived from GCM outputs of precipitation, temperature, and evaporation. Stream flow and spring discharge were taken from MFM outputs. Evaporation was derived from the temperature-based Hargreaves equation (Hargreaves, 1982; Hargreaves et al., 1985) using daily GCM minimum and maximum temperatures. The evaporation data were aggregated to monthly values. WAM Input Files The Water Rights Analysis Package (WRAP) (Wurbs, 2021) serves as the generalized modeling platform for the TCEQ WAM. WRAP input files are comprised of fixed column width text files. Following the quantile mapping process, WRAP input files are created for use in the CRB WAM, incorporating the period of record hydrology as modified through quantile mapping. The WRAP input files include data for stream flow, net lake evaporation, and spring discharge, each of which are adjusted to reflect future hydrologic conditions based on the climate scenarios. The following sections describe the process of generating WRAP input files for the WAM that incorporate the modified hydrology data obtained through the quantile mapping process. Naturalized Flow File – FLO The FLO file contains data for the 43 control points in the CRB, which are the same control points used in the MFM. Two additional control points outside the CRB are included in the FLO files, but they are assigned zero-value placeholders since they do not contribute to the hydrology of the basin. The results from the quantile mapping process are directly formatted into the FLO file. Net Evaporation File – EVA The EVA file contains net evaporation data for the reservoirs in the CRB. GCM outputs of precipitation and maximum and minimum temperature were re-gridded to 27 quadrangles, as described in the Task 3 memo, to provide inputs for modeling the relationship of flow to weather. The WAM reservoir net evaporation equations require data from 21 quadrangles. The additional 6 quadrangles were used in the MFM process. The method to create the net evaporation inputs for the WAM EVA file is described in the report by Pauls et al. (2013) and is based on work to develop the initial version of the CRB WAM as described in the report by the R.J. Brandes Company (2001). The method to create the net evaporation inputs consists of two general steps: D-102 DRAFT – Water Forward 2024 Appendix D reservoir site. Precipitation * Multiplier. 1. Calculate weighted evaporation and weighted precipitation from quadrangles near the 2. Calculate reservoir net evaporation using the formula: Weighted Evaporation – Weighted The weights used in step 1 were developed using inverse distances from the reservoir to the adjacent quadrangle centers. The multipliers used in step 2 consist of a set of monthly values, which are less than 1.0, based on runoff coefficients which represent the runoff which would have occurred from the reservoir area in the absence of the reservoir. The quadrangle weighting equations and the multipliers are contained within the report by Pauls et al. (2013). Flow Adjustments File – FAD Spring flow discharges in the WAM are modeled as point sources that enter the stream network at a specific control point and flow downstream. The spring discharge volumes are known as “flow adjustments” to the naturalized surface stream flow in the WAM and are input using the FAD file. The monthly CRB WAM spring discharge volumes are based on USGS gage measurements at two springs: Barton Springs near Austin (USGS No. 08155500) and Dove Creek Springs near Knickerbocker (USGS No. 08129500). The FAD file also includes 12 additional springs in the basin, with 11 deriving their discharges from Dove Creek Springs and only Cold/Deep Eddy spring deriving its discharge from Barton Springs using a multiplier factor equal to 4%. Other springs located within the basin are not separated from the historical naturalized flows. Thus, the springs that are not explicitly included in the FAD file are not modeled separately from the surface naturalized flows included in the FLO file. The original WAM linear regression equations (R.J. Brandes Co., 2001; Pauls et al., 2013) for the discharges based on Dove Creek Springs contain constants, also known as the linear regression intercept parameter, which represent constant spring discharge volumes. Extended periods of near-zero discharges of Dove Creek Springs are not observed in the historical record and thus did not influence fitting of the slope and intercept parameters of the linear regression equations. As a result, in some climate scenario outputs from the MFM in which Dove Creek Springs exhibits zero or near-zero monthly discharges, the constant flows produced by the original linear regression equations will not be realistic. To address this issue of low flow discharges at the 12 springs (target springs) for which discharge is based on a regression equation with Dove Creek Springs, an alternative set of equations was created. The alternative set of equations gradually reduces the target spring discharges as Dove Creek approaches zero discharge. The general form of the alternative set of equations for the target spring is as follows: • • • If Dove Creek Springs discharge is zero, the target spring discharge is zero. If Dove Creek Springs discharge multiplied by the target spring’s slope parameter is greater than the intercept parameter in the original linear regression equation, then target spring discharge is set equal to the value of the linear regression equation. If neither of the above are true, then the target spring discharge is equal to a fitted non- linear equation that gradually reduces the discharge towards zero. This approach allows the flow adjustments for the springs to reflect the full range of projected hydrologic conditions, including those with zero or near-zero monthly discharges. Figure 1 below shows the spring discharge at Main/Gov’t Springs and Dove Creek Springs. Main/Gov’t D-103 DRAFT – Water Forward 2024 Appendix D Springs is included in the FAD file at control point E40260. The WAM’s linear regression equation for Main/Gov’t Springs is shown as the orange line. Intermittent USGS observations for Main/Gov’t Springs versus coinciding observations at Dove Creek Springs from the early 1950s through 2016 are shown as hollow circles in the figure. The WAM’s linear regression equation was fit to the observations. During this observational period, the lowest monthly average discharge at Dove Creek Springs was approximately 3.3. cfs. The alterative set of equations for Main/Gov’t Springs is shown as the blue line in the figure. In the flow projections based on climate scenarios, the discharge of Main/Gov’t Springs is gradually reduced towards zero as the discharge of Dove Creek Springs reduces towards zero. The alternative sets of equations for the remaining springs which are based on Dove Creek Springs discharge follow the same format as shown in the figure. s f c , e g r a h c s i D g n i r p S t ' v o G / n a M i 45 40 35 30 25 20 15 10 5 0 0 5 10 15 20 Dove Creek Springs Discharge, cfs 25 30 35 40 Original Equation Alternative Equations Observations Figure 1. Main/Gov’t Springs and Dove Creek Springs Results This section presents the results of creating adjusted hydrologic input files for the 1940-2022 period of record. As described in Section 2, selected periods of the flows, precipitation, and evaporation derived from GCM projections were mapped onto the sequences in the WAM’s historical hydrologic input files. The selected periods from the GCM projections correspond to seven future time horizons being evaluated in WF24. After mapping the hydrologic variables from the GCM projections onto the historical sequences, input files were developed in a format that is accessible by the WAM’s generalized computer model, WRAP. D-104 DRAFT – Water Forward 2024 Appendix D An example of the adjustment to naturalized stream flow for the Colorado River at Austin control point is shown in the next several figures. The MFM naturalized stream flows for the GCM 2015- 2100 projection period using SSP1-2.6 are shown in a cumulative format in Figure 2. The flows derived from each GCM are shown as colored lines and the mean monthly historical flow from the historical WAM FLO file is represented as a dotted line. The 2040 time horizon, corresponding to the 31-year centered period of 2025-2055, is labeled with a grey box. The projected GCM-based monthly flows falling within the grey box are used in the quantile mapping procedure to adjust the historical sequence of flows at this control point. ) t e e f - e r c a M ( l w o F e v i t a u m u C l 160 140 120 100 80 60 40 20 0 2040 Horizon 2010 2020 2030 2040 2050 2060 2070 2080 2090 2100 2110 WAM Mean ACCESS CNRM EARTH KACE UKESM Figure 2. Cumulative projected monthly flows for the Colorado River at Austin, SSP1-2.6 The results of the quantile mapping process are shown in Figure 3. The flows in the figure are presented in annual volumes for display purposes, but the underlying data are monthly time steps. The historical flows for the Colorado River at Austin are given in the upper left bar chart of the figure. The mean annual flow for the 1940-2022 period of record, the standard deviation of annual flows for the 1940-2022 period of record, and the mean annual flows for 2011-2014 are displayed on the bar chart. The mean annual flow for 2011-2014 is given to highlight the lowest inflow years within the historical drought of record. The quantile mapping results for each GCM are shown within the figure with the same color coding from Figure 2. A wide range of results are visible in Figure 3, ranging from higher mean annual flow and higher maximum annual flow in the ACCESS, KACE, and UKESM bar charts to lower mean annual flow for the CRNM and EARTH bar charts. In this example, all of the adjusted annual flow bar charts indicate a lower mean annual flow for the 2011-2014 period. D-105 DRAFT – Water Forward 2024 Appendix D WAM Historical POR ACCESS-CM2 Mean = 1.56M af StDev = 0.91M af Mean '11-'14 = 0.54M af Mean = 1.67M af StDev = 1.20M af Mean '11-'14 = 0.46 af 0 4 9 1 4 4 9 1 8 4 9 1 2 5 9 1 6 5 9 1 0 6 9 1 4 6 9 1 8 6 9 1 2 7 9 1 6 7 9 1 0 8 9 1 4 8 9 1 8 8 9 1 2 9 9 1 6 9 9 1 0 0 0 2 4 0 0 2 8 0 0 2 2 1 0 2 6 1 0 2 0 2 0 2 0 4 9 1 4 4 9 1 8 4 9 1 2 5 9 1 6 5 9 1 0 6 9 1 4 6 9 1 8 6 9 1 2 7 9 1 6 7 9 1 0 8 9 1 4 8 9 1 8 8 9 1 2 9 9 1 6 9 9 1 0 0 0 2 4 0 0 2 8 0 0 2 2 1 0 2 6 1 0 2 0 2 0 2 CNRM-CM6-1 EC-Earth3 Mean = 1.48M ac-ft StDev = 0.88M ac-ft Mean '11-'14 = 0.39M af Mean = 1.03M ac-ft StDev = 0.58M ac-ft Mean '11-'14 = 0.34M af 0 4 9 1 4 4 9 1 8 4 9 1 2 5 9 1 6 5 9 1 0 6 9 1 4 6 9 1 8 6 9 1 2 7 9 1 6 7 9 1 0 8 9 1 4 8 9 1 8 8 9 1 2 9 9 1 6 9 9 1 0 0 0 2 4 0 0 2 8 0 0 2 2 1 0 2 6 1 0 2 0 2 0 2 0 4 9 1 4 4 9 1 8 4 9 1 2 5 9 1 6 5 9 1 0 6 9 1 4 6 9 1 8 6 9 1 2 7 9 1 6 7 9 1 0 8 9 1 4 8 9 1 8 8 9 1 2 9 9 1 6 9 9 1 0 0 0 2 4 0 0 2 8 0 0 2 2 1 0 2 6 1 0 2 0 2 0 2 KACE-1-0-G Mean = 1.94M ac-ft StDev = 1.57M ac-ft Mean '11-'14 = 0.34M af UKESM1-0-LL Mean = 1.60M ac-ft StDev = 1.22M ac-ft Mean '11-'14 = 0.47M af ) t f - c a M ( w o F l l a u n n A ) t f - c a M ( w o F l l a u n n A ) t f - c a M ( w o F l l a u n n A 8 7 6 5 4 3 2 1 0 8 7 6 5 4 3 2 1 0 8 7 6 5 4 3 2 1 0 ) t f - c a M ( w o F l l a u n n A ) t f - c a M ( w o F l l a u n n A ) t f - c a M ( w o F l l a u n n A 8 7 6 5 4 3 2 1 0 8 7 6 5 4 3 2 1 0 8 7 6 5 4 3 2 1 0 0 4 9 1 4 4 9 1 8 4 9 1 2 5 9 1 6 5 9 1 0 6 9 1 4 6 9 1 8 6 9 1 2 7 9 1 6 7 9 1 0 8 9 1 4 8 9 1 8 8 9 1 2 9 9 1 6 9 9 1 0 0 0 2 4 0 0 2 8 0 0 2 2 1 0 2 6 1 0 2 0 2 0 2 0 4 9 1 4 4 9 1 8 4 9 1 2 5 9 1 6 5 9 1 0 6 9 1 4 6 9 1 8 6 9 1 2 7 9 1 6 7 9 1 0 8 9 1 4 8 9 1 8 8 9 1 2 9 9 1 6 9 9 1 0 0 0 2 4 0 0 2 8 0 0 2 2 1 0 2 6 1 0 2 0 2 0 2 Figure 3. Historical (top left) and adjusted annual flow for the Colorado River at Austin An additional set of adjusted hydrologic input files was generated as the average across all five GCM results, referred to as an ensemble. The monthly flows corresponding to Figure 3 were averaged and the ensemble results are shown in Figure 4. The historical flow bar chart from D-106 ) t f - c a M ( w o F l l a u n n A 8 7 6 5 4 3 2 1 0 DRAFT – Water Forward 2024 Appendix D Figure 3 is replicated in Figure 4 for comparative purposes. The averaging process to create the ensemble smooths over individual monthly values, lowering peak flows and raising minimum flows. The intent of creating the ensemble was to develop a set of WAM hydrologic inputs that reflects the overall trend across the time horizons and emission scenarios as temperatures increase towards the end of century. WAM Historical POR Ensemble Mean = 1.56M af StDev = 0.91M af Mean '11-'14 = 0.54M af Mean = 1.54M ac-ft StDev = 0.97M ac-ft Mean '11-'14 = 0.40M af ) t f - c a M ( w o F l l a u n n A 8 7 6 5 4 3 2 1 0 0 4 9 1 4 4 9 1 8 4 9 1 2 5 9 1 6 5 9 1 0 6 9 1 4 6 9 1 8 6 9 1 2 7 9 1 6 7 9 1 0 8 9 1 4 8 9 1 8 8 9 1 2 9 9 1 6 9 9 1 0 0 0 2 4 0 0 2 8 0 0 2 2 1 0 2 6 1 0 2 0 2 0 2 0 4 9 1 4 4 9 1 8 4 9 1 2 5 9 1 6 5 9 1 0 6 9 1 4 6 9 1 8 6 9 1 2 7 9 1 6 7 9 1 0 8 9 1 4 8 9 1 8 8 9 1 2 9 9 1 6 9 9 1 0 0 0 2 4 0 0 2 8 0 0 2 2 1 0 2 6 1 0 2 0 2 0 2 Figure 4. Historical and the ensemble of adjusted annual flow for the Colorado River at Austin Reservoir storage in lakes Buchanan and Travis is a key variable in WF24 water availability modeling. Both lakes are located upstream of the control point for the Colorado River at Austin. Using the ensemble results of total naturalized flow at control points upstream of Austin, an estimate was created of potential reservoir inflows by subtracting the naturalized flow at control points for the Colorado River near Stacy and Pecan Bayou at Brownwood from the naturalized flow at the control point for the Colorado River at Lake Travis. The remaining flow after the subtraction is similar to the “cutoff” assumption used in TWDB Region K water planning and other water availability studies of the Lower Colorado River basin, including WF24. Figure 5 shows the estimated potential annual reservoir inflows and reservoir net evaporation for the ensembled results for the seven future time horizons and for each SSP. The two periods shown in the figure correspond to the entire observational record used in this study and the 2010s drought. The flow data shown in Figure 5 are only estimates and are based on the subtraction method discussed above and are presented here for highlighting overall trends. The WAM simulated reservoir inflow volumes will vary based on simulated water right utilization in the drainage area upstream of lakes Buchanan and Travis. The WAM historical mean annual value is shown in each graph of Figure 5 for comparative purposes. Graphs on the top row of the figure show results for the entire historical and adjusted historical period of record. Graphs on the bottom row of the figure show results for the critical reservoir drawdown period of the 2010s drought. The ensemble results of reservoir inflow and reservoir net evaporation for the critical drawdown period are presented here to illustrate the trend over time and across emission scenarios. The overall trend shown in Figure 5 indicates decreasing reservoir inflows and increasing net evaporation as the GCM projections progress towards the end of the century. The least D-107 DRAFT – Water Forward 2024 Appendix D reduction in reservoir inflow and least increase in reservoir net evaporation can be seen in the results for the lower emission scenario, SSP1-2.6. The ensemble results for SSP1-2.6 also contain the only increase in the 1940-2022 adjusted period of record mean annual inflows, occurring in the earlier to mid-century portion of the projection. Flow, 1940-2022 Net Evap, 1940-2022 3.5 3.0 2.5 2.0 1.5 1.0 0.5 0.0 ) r a e y / t f ( h t p e D l a u n n A n a e M 3.5 3.0 2.5 2.0 1.5 1.0 0.5 0.0 ) r a e y / t f ( h t p e D l a u n n A n a e M Historical SSP1-2.6 SSP2-4.5 SSP5-8.5 Historical SSP1-2.6 SSP2-4.5 SSP5-8.5 Flow, Oct. 2007 - Apr. 2015 Net Evap, Oct. 2007 - Apr. 2015 Historical SSP1-2.6 SSP2-4.5 SSP5-8.5 Historical SSP1-2.6 SSP2-4.5 SSP5-8.5 Figure 5. Historical and ensemble mean annual reservoir inflow and net evaporation 1.4 1.2 1.0 0.8 0.6 0.4 0.2 0.0 ) r a e y / t f - c a M ( w o F l l a u n n A n a e M 1.4 1.2 1.0 0.8 0.6 0.4 0.2 0.0 ) r a e y / t f - c a M ( w o F l l a u n n A n a e M D-108 DRAFT – Water Forward 2024 Appendix D Summary The primary objective of this study was to generate adjusted hydrologic input files for the CRB WAM, reflecting future conditions for stream flow, net evaporation, and spring flow. The files were derived from adjustments to the historical hydrologic sequences spanning 1940-2022, based on the projections of five GCMs paired with three emission scenarios, known as SSPs, and at seven different time horizons. The adjusted hydrologic input files provide a diversity of future conditions for evaluating water availability in WF24. In addition to the adjusted hydrologic inputs derived from the five GCMs, an ensemble set of input files was created by averaging the results across all five GCMs. This ensemble approach provided further insights into the trends of the future projections. Generally, the trend in hydrologic conditions within this study indicates a shift towards decreasing surface water stream flows and increasing net evaporation towards the end of the century compared to historical conditions. On an individual GCM, SSP, and time horizon basis, the adjusted hydrologic input files contain various levels of change around the overall trend. The findings of this study highlight the diversity of the possible outcomes for hydrologic conditions over the WF24 planning horizons. Use of multiple climate models, emission scenarios, and time horizons provides a basis for robust evaluation of water management strategies in WF24 that can accommodate a range of potential future conditions. D-109 DRAFT – Water Forward 2024 Appendix D References Boé, J., Terray, L., Habets, F. and Martin, E. (2007). Statistical and dynamical downscaling of the Seine basin climate for hydro-meteorological studies. Int. J. Climatol., 27: 1643-1655. doi:10.1002/joc.1602 Hargreaves, G.H., Samani, Z.A. (1982). Estimating Potential Evapotranspiration, Journal of the Irrigation and Drainage Division, Vol. 108, Issue 3, pp. 225-230. doi: 10.1061/JRCEA4.0001390 Hargreaves, G.H., Samani, Z.A. (1985). Reference Crop Evapotranspiration from Temperature, Applied Engineering in Agriculture, Vol 1, No. 2, pp. 96-99. doi: 10.13031/2013.26773 Pauls, M.A, Hoffpauir, R.J., Wurbs, R.A., (2013). Hydrologic Period-of-Analysis Extension for the Colorado River Basin and Brazos-Colorado Coast Basin Water Availability Model. Texas A&M University. 455 p. R.J. Brandes Company (2001). Water Availability Modeling for the Colorado and Brazos Colorado Basin Water Availability Assessment – Final Report, Prepared for Texas Natural Resource Conservation Commission. WMO, World Meteorological Organization (2007). The role of climatological normals in a changing climate. WMO/TD-1377, 130 p., https://library.wmo.int/index.php?lvl=notice_display&id=16659 Wurbs, R.A., (2021). Water Rights Analysis Package (WRAP) modeling system reference manual. 13th Edition. College Station, Texas: Texas Water Resources Institute. Technical Report 256. 273 p. D-110 DRAFT – Water Forward 2024 Appendix D Task 6: Stochastic Drought Sequences Prepared by: Hoffpauir Consulting Summary This memo documents the creation of stochastic sequences of naturalized flow and to the selection of drought events within those sequences which are different and worse than the drought sequence of the 2010s, also known as the drought of record (DOR). Both historical and climate change adjusted naturalized flows are used. The goal of Task 6 within the Water Forward 2024 (WF24) Climate and Hydrology Analysis is to create hydrologic inputs files for the WF24 Colorado River Basin (CRB) Water Availability Model (WAM) which will be used for additional simulation analyses of water supply needs and water management strategies (WMS) under historical and climate adjusted conditions. Drought events for the CRB occur over multi-year periods, and each event represents a unique pattern of drying and relief episodes within the overall drought. The historical hydrologic record contains two major droughts, known as the 1950s and 2010s droughts, with the 2010s drought being recognized as the worst drought event with respect to water availability since the construction of the Highland Lakes. By applying climate adjustments to the period of record hydrology, as documented in the Task 5 memo, the 1950s and 2010s droughts are re- characterized for future periods under differing climate change conditions. However, the sequential pattern of drying and relief episodes within the 1950s and 2010s droughts are the same, whether using historical or climate adjusted conditions. Stochastic sampling of the historical and climate adjusted hydrology is performed to develop potential drought events that differ from the patterns of those within the observed record. Drought events within the stochastically sampled hydrology are identified and characterized for duration and cumulative streamflow deficit. A selection process is then performed on the overall set of stochastic drought events to choose a small number of events which are estimated to be worse than the 2010s sequence. The selected stochastic droughts will be used in WF24 WAM simulations to examine the effects on water availability. The stochastic droughts along with the historical and climate adjusted historical hydrology are needed to estimate water supply needs and to test the performance of WF24 recommended WMS over a wide range of hydrologic conditions. Task 5 resulted in climate adjusted hydrology for three greenhouse gas emission scenarios at seven time horizons from year 2030 through 2100. The Task 6 work produced 10 stochastic drought events for each of the climate adjusted hydrologies and the historical hydrology. Thus, Task 6 increases the number of hydrologic sequences for WF WAM analyses by 40 at each time horizon. D-111 DRAFT – Water Forward 2024 Appendix D GCM SSP TCEQ TWDB USGS WAM WF24 WMS List of Acronyms CRB Colorado River Basin Global Climate Model Shared Socioeconomic Pathways Texas Commission on Environmental Quality Texas Water Development Board United States Geological Survey Water Availability Model Water Forward 2024 Water Management Strategies D-112 DRAFT – Water Forward 2024 Appendix D Background The Water Forward Climate and Hydrology Analysis is a study designed to produce projected climate data and time series of hydrologic conditions for use in simulation analyses. The technical work of the Climate and Hydrology Analysis is organized in several Tasks and documented in technical memoranda. Task 2 involved the selection of general circulation models (GCMs) from Phase 6 of the Coupled Model Intercomparison Project (CMIP6) and future greenhouse gas emission scenarios from the Shared Socio-Economic Pathways (SSPs) framework. Task 3 applied methods to downscale weather variables from the selected GCMs to locations within and surrounding the Colorado River Basin in Texas. Task 4 then used these downscaled weather variables to create a model that generates naturalized streamflow for the Colorado River Basin under historical and projected climate change conditions. In Task 5, historical hydrologic records were adjusted to reflect specific future time horizons within the climate projections from Task 4. Task 5 produced climate-adjusted hydrology for 5 GCMs, 3 SSPs, and 7 time horizons and an ensemble of climate-adjusted hydrologic records by averaging hydrologic conditions according to SSP and time horizon. Task 6, the focus of this memorandum, builds on the previous tasks and develops stochastic drought sequences using both the historical hydrologic records and the ensemble of climate-adjusted hydrology. This study develops time series of unique drought events under various climate scenarios, which will be used within the Water Availability Model (WAM) to assess water supply needs and to test water management strategies (WMS) within the WF24 Plan. Assessing needs and water management strategies against drought sequences that differ from historical observations is important for evaluating their robustness and adaptability under potential future conditions, both with and without climate change. This study does not evaluate the likelihood of these stochastic drought sequences but rather generates a range of plausible scenarios. This expands the hydrological context for planning without assigning specific probabilities to individual events. One of the major uncertainties for characterizing water availability in the Colorado River Basin (CRB) involves droughts worse than the drought of record (DWDOR). DWDOR events, by definition, represent droughts that have not yet occurred and are not part of the observational record. With only two major multi-year droughts in the observed CRB record – the 1950s and 2010s droughts – the small sample size limits the understanding of the possible range of hydrological extremes. The 2010s drought, the most severe in the historical record, provides a benchmark, but stochastic methods allow for testing scenarios that may exceed this drought's severity. To explore the range of possible hydrological extremes, the WF24 Plan uses synthetic hydrological sequences using a stochastic technique known as Markov chain simulation. This methodology stochastically re-sequences the 1940–2022 period of record by selecting and connecting whole calendar years of hydrology in a new and non-sequential order. Each sampled year is conditioned based on the observed frequency of transitions between wet and dry years, ensuring that the new sequence retains the same transition dynamics and long-term statistical properties as the underlying observed record. A long sequence of extended synthetic hydrology that preserves the statistical characteristics of the observed period is a common technique to augment limited historical data for river and reservoir analyses of water availability (Wurbs, 1991). The extended synthetic hydrology generated in this study preserves the statistical characteristics of the historical and climate adjusted historical record while introducing new multi-year events. The new sequence allows for random occurrences of events that are wetter or drier than those observed historically. Multi- D-113 DRAFT – Water Forward 2024 Appendix D year droughts generated through this method can result in droughts that are more severe than the 2010s drought sequence. Data WAM monthly naturalized stream flows and reservoir net evaporation for historical and climate adjusted conditions are used in this study. In total, 22 sets of WAM hydrology are used in this study and consist of 1 historical and 21 ensemble climate adjusted conditions. The monthly hydrologic data was extended to cover the 1940-2022 period of record as described in the Climate and Hydrology Analysis Task 4 memo. The creation of an ensemble climate adjusted 1940-2022 WAM hydrology is described in the Climate and Hydrology Analysis Task 5 memo. In essence, the 1940-2022 pattern of monthly stream flow and reservoir net evaporation is retained, but the values are replaced with hydrologic projections from each SSP and each time horizon using quantile mapping. Markov Chains A Markov chain is a type of stochastic modeling process that assigns the probability of an event based on the state of the prior event (Maidment, 1993). In the case of streamflows, a Markov chain model assigns the probability for a designated flow state to be followed by the same or different flow state. A key feature of a Markov chain is its memoryless property, meaning the probability of transitioning to a new state depends only on the current state and not on the sequence of states that preceded it. Markov chains are useful when simulating systems that can be represented as transitioning over time in a step-by-step manner. The dependency of the future state based only on the prior state is known as a first-order Markov process. Stochastic streamflow generation is commonly performed as a first-order Markov process (Maidment, 1993; Yeh, 1985) and is used in this study. The states are defined on a yearly basis and are based on the total annual naturalized flow being below or above the 1940-2022 median flow. Nominally, the years are designated as “dry” or “wet”. Two locations are chosen in the Lower CRB, near the outlet at the Bay City gage and the location of Lake Travis. Two locations are used to capture the spatial variability of wet and dry cycles between the upper and lower portions of the Lower CRB. With two locations and two states at each location, there are a total of four states defined for the Markov chain. When the annual flow is below the respective medians at both locations, the whole year is designated as a “dry-dry” state. When the annual flow is above median flow at Lake Travis and below median flow at Bay City, the whole year is designated as a “wet-dry” state. The first descriptor in each state is a reference to Lake Travis and the second descriptor references flows at Bay City. Figure 1 illustrates the annual state categorization process of WAM naturalized flows under historical conditions. The two bar charts on the left side of Figure 1 are the annual naturalized flows at Lake Travis and Bay City. The bars are colored blue if the annual flow is above the median at the respective location, and colored orange if the annual flow is below the median. The right portion of Figure 1 shows the state designation for each year. The number of each state is shown in the legend. For example, there are 34 years that are designated as dry-dry. D-114 DRAFT – Water Forward 2024 Appendix D ) t f - c a M ( l w o F s i v a r T e k a L 4 3 2 1 0 ) t f - c a M ( l w o F y t i C y a B 10 8 6 4 2 0 0 4 9 1 4 4 9 1 8 4 9 1 2 5 9 1 6 5 9 1 0 6 9 1 4 6 9 1 8 6 9 1 2 7 9 1 6 7 9 1 0 8 9 1 4 8 9 1 8 8 9 1 2 9 9 1 6 9 9 1 0 0 0 2 4 0 0 2 8 0 0 2 2 1 0 2 6 1 0 2 0 2 0 2 Dry Wet Median 0 4 9 1 5 4 9 1 0 5 9 1 5 5 9 1 0 6 9 1 5 6 9 1 0 7 9 1 5 7 9 1 0 8 9 1 5 8 9 1 0 9 9 1 5 9 9 1 0 0 0 2 5 0 0 2 0 1 0 2 5 1 0 2 0 2 0 2 Dry-Dry (34) Dry-Wet (7) Wet-Dry (7) Wet-Wet (35) 0 4 9 1 4 4 9 1 8 4 9 1 2 5 9 1 6 5 9 1 0 6 9 1 4 6 9 1 8 6 9 1 2 7 9 1 6 7 9 1 0 8 9 1 4 8 9 1 8 8 9 1 2 9 9 1 6 9 9 1 0 0 0 2 4 0 0 2 8 0 0 2 2 1 0 2 6 1 0 2 0 2 0 2 Dry Wet Median Figure 1. Historical annual naturalized flows at Lake Travis and Bay City and the associated annual states The transition probabilities between states are calculated once the states for each year are characterized. Figure 2 shows the states and transition probabilities for the annual flows. Within the left figure, if the current year is in a dry-dry state, then the next year will remain dry-dry with a 46% chance, or transition to dry-wet with a 3% chance, or wet-wet with a 36% chance, or wet- dry with a 15% chance. The chances are calculated from the sequential progression of each year of states in the 1940-2022 record. In this example, the wet-dry state does not have a probability to remain wet-dry in the following year and must transition to either wet-wet or dry- dry. Neither example shown in Figure 2 contains a transition between dry-wet and wet-dry states. Each of the 22 hydrologic scenarios in this study have a unique set of transition probabilities. The R package markovchain (Spedicato, 2017) for handling Discrete Time Markov Chains was used to calculate the transition probabilities between the four states: dry-dry, dry- wet, wet-dry, and wet-wet. D-115 DRAFT – Water Forward 2024 Appendix D Figure 2. Markov transition probabilities for historical conditions (left) and SSP5-8.5 for the 2100 time horizon (right) Using the transition probabilities, the R package markovchain was used again to stochastically simulate 1.1 million annual states for each of the 22 hydrologic scenarios. The first 10% of the simulation is discarded as a “burn in” sequence, leaving 1 million annual states to proceed with year assignments. The long simulation length is intended to provide, as discussed later, a large sample of multi-year droughts to be analyzed. Figure 3 illustrates the first 500 years of the 1 million simulated states under historical conditions. 1 2 1 3 2 4 3 5 4 6 5 7 6 8 7 9 8 0 0 1 1 1 1 2 2 1 3 3 1 4 4 1 5 5 1 6 6 1 7 7 1 8 8 1 9 9 1 0 1 2 1 2 2 2 3 2 3 4 2 4 5 2 5 6 2 6 7 2 7 8 2 8 9 2 9 0 3 0 2 3 1 3 3 2 4 3 3 5 3 4 6 3 5 7 3 6 8 3 7 9 3 8 0 4 9 1 4 0 3 4 1 4 4 2 5 4 3 6 4 4 7 4 5 8 4 6 9 4 Dry-Dry Dry-Wet Wet-Dry Wet-Wet Figure 3. First 500 years of simulated annual states under historical conditions After generating 1 million stochastic annual states, the years from the 1940-2022 hydrologic record are randomly assigned to create a 1-million-year sequence. The assignment is not entirely random but is conditioned to evenly select of each years across the 1 million simulated states and further conditioned to maintain a low exceedance of multi-year low flow and high flow frequencies at the Lake Travis and Bay City locations from the original 1940-2022 data. Figure 4 shows the number of times each year is selected for the historical simulation. Some years are selected slightly less than others in Figure 4. For historical conditions, the simulation of the transition probabilities as shown on the left side of Figure 2 results in steady state occurrences of: 41.6% dry-dry, 8.4% dry-wet, 8.7% wet-dry, and 41.3% wet-wet. For comparison, there are 35 years in the 1940-2022 data that are designated as wet-wet, or 42.2% of the years. These small differences in the Markov chain stead state versus the observed occurrences in the data contribute to differences in the bar heights of Figure 4. D-116 DRAFT – Water Forward 2024 Appendix D l s n o i t c e e S f o r e b m u N 14000 12000 10000 8000 6000 4000 2000 0 0 4 9 1 4 4 9 1 8 4 9 1 2 5 9 1 6 5 9 1 0 6 9 1 4 6 9 1 8 6 9 1 2 7 9 1 6 7 9 1 0 8 9 1 4 8 9 1 8 8 9 1 2 9 9 1 6 9 9 1 0 0 0 2 4 0 0 2 8 0 0 2 2 1 0 2 6 1 0 2 0 2 0 2 Figure 4. Number of times each year is selected in the 1 million year historical sequence The WAM naturalized flows are time series of monthly volumes and provided at locations of United States Geological Survey (USGS) stream gages or major reservoir sites throughout the CRB. The process of selecting, rearranging, and building WAM hydrology input files based on whole calendar years does not change the monthly time step used by the WAM. The use of whole calendar years was used as a simplifying assumption for defining Markov chain states and for easing the selection and rearrangement of whole calendar years within the WAM’s hydrology input files for all locations throughout the basin. Rearrangement of the WAM hydrology input files on a whole calendar year basis is also supported in this study by the focus on multi-year drought events rather than short-term or sub-annual droughts. Drought Detection Within the 1-million-year sequence of stochastic hydrology created for the entire CRB WAM, the hydrologic conditions will fluctuate between wet and dry periods. Specifically of interest are the multi-year dry periods that can be characterized as water supply droughts. In this study, water supply droughts are the periods of time in which Austin’s primary source of water supply back up, Lakes Buchanan and Travis, will experience significant drawdowns during the WAM simulation. The Markov chain process described in the previous section was designed to capture spatial variability of wet and dry cycles between the upper and lower portions of the Lower CRB. The drought detection methodology of the study will focus on identifying and ranking droughts that may affect firm water supply and the key reservoir levels which initiate drought management responses for Austin during the simulation. The Sequent Peak Algorithm (SPA) was selected for this study as the water supply drought screening tool (Lele, 1987; Fleig et al., 2006) because it satisfied several criteria. It is a computationally efficient screening tool for detecting drought periods, particularly for this study which uses 1 million years of stochastic hydrology for each of 22 hydrologic scenarios. Secondly, the SPA outputs are directly interpretable as a time series of reservoir storage. Finally, SPA was found to produce similar results for reservoir storage drawdown and refilling D-117 ) t f - c a M ( e g a r o t S d e n b m o C i 2.5 2.0 1.5 1.0 0.5 0.0 DRAFT – Water Forward 2024 Appendix D cycles when compared to outputs of the WAM simulation. Figure 5 compares the simulated combined storage of Buchanan and Travis for WAM and SPA in which both models utilize the full firm yield of the lake system. As seen in Figure 5, SPA produces very similar results compared with the WAM simulated storage time series. 1940 1950 1960 1970 1980 1990 2000 2010 2020 WAM SPA Figure 5. Comparison of WAM and SPA results The SPA is essentially an automated version of the graphical Rippl mass curve method. The algorithm begins with a time series of inflows that are available for meeting demands and storing in a reservoir. The user selects a threshold, or yield, for delineating deficits or surplus to the reservoir. Deficits result in storage drawdown and surpluses result in reservoir filling. The deficits are accumulated in each time step, monthly in this study, until surpluses cancel out the previously accumulated deficits. Drought periods are therefore the periods of time between zero accumulated deficits, or full reservoir storage. The maximum accumulated deficit – the “peak” – is the amount of storage required to meet the yield without shortage during the drought period. The drought duration is the amount of time from the start of the drought until peak, also known as the critical period. Figure 6 shows the SPA concept. The available monthly streamflow is shown as the grey dotted line and fluctuates between dry and wet periods. When the streamflow falls below the yield, shown as the red dotted line, a deficit volume is calculated and accumulated in the black solid line. Three drought periods are shown in Figure 6 with differing durations and peak deficits. The second drought period is considered the most severe in the figure as its peak deficit is greater than the others. D-118 DRAFT – Water Forward 2024 Appendix D Peak Def. 2 Peak Def. 1 Peak Def. 3 20,000 15,000 10,000 5,000 ) t f - c a ( w o l f m a e r t S 0 0 20,000 15,000 10,000 5,000 ) t f - c a ( e m u l o V t i c i f e D 0 80 20 40 60 Duration 1 Duration 2 Duration 3 Time (months) Streamflow Deficit Volume Theshold Figure 6. Sequent Peak Algorithm example In this study, the conventional streamflow-only SPA method was modified to also consider evaporation from the simulated reservoir surface (Lele, 1987; Adeloye and Montaseri, 2001). Net evaporation time series data from the WAM hydrology input were used in addition to the available reservoir inflows to the Highland Lakes. A simplified storage volume and surface area relationship was calculated for the combined capacity of lakes Buchanan and Travis. The deficit volume accumulated by SPA was used to estimate reservoir surface area and the net evaporation volume. The net evaporation volume was added to the monthly SPA yield. The consideration of evaporation effects was found to improve the agreement between SPA and the WAM simulation results. The SPA yield for accumulating deficits was calculated for the 2010s drought-of-record in each of the hydrologic scenarios. Knowing that the 2010s is the worst drought in the 1940-2022 historical and climate adjusted hydrologic scenarios, the SPA yield was chosen to achieve approximately a 2M acre-feet maximum cumulative deficit for the 2010s drought. The 2M acre- feet deficit represents utilization of the entire combined storage capacity of lakes Buchanan and Travis during the drought-of-record and parallels the assumptions for calculation of the lake system’s firm yield in the WAM. Different SPA yields were calculated for each of the hydrologic scenarios which represent different climate conditions. The SPA was applied across each hydrologic scenario using the previously calculated yield and 1M years of lake inflows and reservoir net-evaporation as inputs. Drought events were identified and characterized according to their peak deficit and the duration from the onset of drought to the peak deficit. Figure 7 shows the SPA drought events (grey dots) identified in the 1M years of stochastic hydrology using historical conditions. Only drought events that achieved at least 12 months or more duration are included in the figure. The SPA duration and deficits for the 1950s and 2010s drought are also shown and included in the figure’s legend as major period-of-record (POR) droughts. Figure 8 shows the same scatter plot of data, but using the SSP5-8.5 climate adjusted hydrology for the end-of-century period. The yields used by SPA for the two figures are D-119 DRAFT – Water Forward 2024 Appendix D different, but set accordingly for a 2M acre-feet peak deficit of their respective 2010s drought sequences. Figure 7. Stochastic drought events for historical hydrologic conditions ) t f - c a M ( t i c i f e D Duration (months) Figure 8. Stochastic drought events for 2100 SSP5-8.5 hydrologic conditions Metrics from the SPA application to each of the hydrologic scenarios is given in Table 1. The yield used for setting a 2M ac-ft peak deficit for the 2010s drought under each hydrologic scenario is shown in the third column. In general, as the climate warms over time and with D-120 DRAFT – Water Forward 2024 Appendix D increasing warming intensity per SSP, the yield tends to decrease to achieve the same peak deficit for the 2010s drought sequence. The declining trend of SPA yield is shown in Figure 9 and is indicative of hotter and drier conditions during extreme droughts. The number of drought events identified during the 1M years of stochastic hydrology are provided along with the average time between events, as known as the mean interarrival time. The number of drought events and interarrival times are shown for all drought events with 12 months of more duration and for drought events with a peak deficit equal to or greater than 2M ac-ft. Figure 10 shows the mean intervariable time for droughts with peak deficit equal to or greater than 2M ac-ft. The decreasing trend of interarrival time for the warmer climate conditions indicates that major drought events become more frequent in the stochastic hydrology. Table 1. Metrics of stochastic drought events D-121 DRAFT – Water Forward 2024 Appendix D Droughts in 1M Year Stochastic Hydrology 12 months duration or more Deficit greater than 2M ac-ft Hydrologic Scenario Planning Horizon SPA Yield (ac-ft/year) Number of Events Mean Interarrival Time (years) Number of Events Mean Interarrival Time (years) Climate Condition Historical SSP1-2.6 SSP1-2.6 SSP1-2.6 SSP1-2.6 SSP1-2.6 SSP1-2.6 SSP1-2.6 SSP2-4.5 SSP2-4.5 SSP2-4.5 SSP2-4.5 SSP2-4.5 SSP2-4.5 SSP2-4.5 SSP5-8.5 SSP5-8.5 SSP5-8.5 SSP5-8.5 SSP5-8.5 SSP5-8.5 SSP5-8.5 457,700 434,900 430,700 438,300 469,900 435,000 396,800 412,600 410,700 401,300 399,500 370,100 356,800 367,900 377,600 409,600 408,800 394,300 416,000 379,200 352,200 334,500 na 2030 2040 2050 2060 2070 2080 2100 2030 2040 2050 2060 2070 2080 2100 2030 2040 2050 2060 2070 2080 2100 170,084 185,343 190,419 189,331 179,697 177,684 169,093 171,039 171,253 167,849 175,693 168,154 174,273 160,981 159,028 177,696 178,533 178,177 175,900 176,778 160,053 144,718 5.9 5.4 5.3 5.3 5.6 5.6 5.9 5.8 5.8 6.0 5.7 5.9 5.7 6.2 6.3 5.6 5.6 5.6 5.7 5.7 6.2 6.9 3,139 3,877 3,516 3,684 3,061 3,453 3,477 3,227 3,962 4,275 4,078 4,360 5,708 9,508 9,537 4,930 5,138 7,192 8,008 7,258 8,951 10,643 319 258 284 271 327 290 288 310 252 234 245 229 175 105 105 203 195 139 125 138 112 94 D-122 DRAFT – Water Forward 2024 Appendix D 400 300 200 100 ) s r a e y ( e m i T l a v i r r a r e t n I n a e M 0 2000 Figure 9. SPA yield of the 2010s drought sequence per hydrologic scenario 2020 2040 2060 2080 2100 2120 Planning Horizon Historical SSP1-2.6 SSP2-4.5 SSP5-8.5 Figure 10. Mean interarrival time between stochastic droughts with peak deficit equal to or greater than 2M ac-ft Drought Selection The next phase of this study involves reducing and then selecting from the entire set of drought events identified by SPA using the 1M-year sequence of stochastic hydrology. The goal of the drought selection process is to pick 10 drought sequences per hydrologic scenario that differ in duration and severity from the 2010s drought sequence. Additional and differing drought sequences will be used for robust testing of water supply needs and WMS. Table 1 and Figures D-123 DRAFT – Water Forward 2024 Appendix D 7 and 8 show that there are thousands of drought events in the stochastic hydrologic sequence that have a peak deficit, or severity, greater than the 2010s drought. However, randomly selecting 10 events from the entire set of droughts worse than the 2010s drought would result in the selection of droughts too severe for planning purposes. The selection of stochastic drought sequences should result in droughts potentially worse than the 2010s drought in terms of duration and severity, but within a reasonable range to avoid over-planning. Figure 11 shows the scatter of stochastic drought events identified as candidates for selection (grey circles) using the historical conditions scenario. Figure 11 is a subset of drought events shown in Figure 7 which have been narrowed according to duration and deficit. Judgement was involved in developing the criteria for the subset. The 2010s drought was characterized as having a critical-period duration of 91 months or 7.6 years. The SPA yield, as described previously, was chosen to produce a maximum deficit of 2M ac-ft. The candidate droughts in Figure 11 were identified as events with a duration of between 4 and 10 years and a deficit of up to 20% greater than the 2010s, or 2.4M ac-ft. The criteria for deficit was slightly relaxed for the identification of candidate droughts with duration greater than the 2010s drought. Finally, stochastic droughts nearest to the 2010s duration and deficit were excluded from the candidate set to avoid selection of events too similar to the 2010s drought and therefore may not provide new information in the water supply needs and WMS testing process. Figure 11. Candidate and selected stochastic droughts for historical hydrology The pink circles in Figure 11 are the selected stochastic droughts from the field of candidates. The selection process was performed by stratified random sampling across the range of candidate deficits and for maximizing the distance between selections in the candidate scatter plot. The selection process was performed for all 22 hydrologic scenarios and resulted in 220 stochastic droughts across the WF planning horizons and climate conditions. Figure 12 shows the candidates and selected stochastic drought events for the 2100 SPP5-8.5 hydrologic scenario. D-124 DRAFT – Water Forward 2024 Appendix D ) t f - c a M ( t i c i f e D Figure 12. Candidate and selected stochastic droughts for 2100 SSP5-8.5 hydrology Duration (months) After selecting stochastic droughts sequences for each hydrologic scenario, separate WAM hydrology input files were built for each drought event. For example, the pink dot farthest to the left in Figure 11 corresponds to WAM hydrologic inputs for the calendar years 2019, 2011, 2014, 2011, and 1971. This event has a maximum deficit of 2.09M ac-ft and a duration of four years. The period-of-record WAM input files for the entire basin are rearranged and reduced to include only those years for simulating the severe drought event. As another example, the middle pink dot farthest to the right in Figure 11 has a similar deficit to the previous example but longer duration. This event corresponds to the calendar years 1943, 1963, 1959, 2013, 1948, 1948, 2014, 1999, 1962, and 1952. The event has a deficit of 2.15M ac-ft and a duration of 9.7 years. Summary This technical memo outlines the development of stochastic drought sequences for the Colorado River Basin as part of the Water Forward 2024 Climate and Hydrology Analysis. The primary objective of Task 6 is to create hydrologic input files for the Water Availability Model, enabling the simulation of droughts that are more severe than the 2010s drought-of-record. By using both historical and climate-adjusted naturalized flow datasets, this task applies stochastic techniques to generate potential drought events, which are analyzed and characterized by duration and cumulative inflow deficit. These stochastic drought events, designed to be distinct from 2010s drought sequence, will be used for robust analyses of water supply needs and water management strategies. Task 6 builds on the climate-adjusted hydrology generated in previous tasks by developing 10 stochastic drought events for each of the historical and climate-adjusted scenario for each D-125 DRAFT – Water Forward 2024 Appendix D planning horizon. The selected droughts are intended to be more severe than the 2010s drought in terms of deficit and/or duration but within a range that supports water supply planning. The results of this approach expand the array of hydrologic conditions being considered and adds to the understanding of how needs develop and strategies perform under future drought scenarios. References Adeloye, A. J., Montaseri, M., & Garmann, C. (2001). Curing the misbehavior of reservoir capacity statistics by controlling shortfall during failures using the modified sequent peak algorithm. Water Resources Research, 37(1), pp. 73-82 Fleig, A.K., Tallaksen, L.M., Hisdal, H., Demuth, S. (2006). A global evaluation of streamflow drought characteristics. Hydrology and Earth System Sciences, 10(4), pp. 535-552. Spedicato, G. (2017). Discrete Time Markov Chains with R. The R Journal, 9(2), pp. 84–104. https://journal.r-project.org/archive/2017/RJ-2017-036/index.html Lele, S.M. (1987). Improved algorithms for reservoir capacity calculation incorporating storage- dependent losses and reliability norm. Water Resources Research, 23(10), pp. 1819-1823. Maidment, D. 1993. Handbook of Hydrology: Chapter 19, Analysis and Modeling of Hydrologic Time Series. McGraw-Hill, Inc., 1424 pages, Edition 1. Wurbs, R. 1991. Optimization of Multiple-Purpose Reservoir System Operations: A Review of Modeling and Analysis Approaches. Research Document 34, NTIS-AD-A236080/8INZ, USACE Hydrologic Engineering Center, Davis, CA, 87 pages. Yeh, W. 1985. Reservoir Management and Operations Models: A State of the Art Review. Water Resources Research, 21(12), 1797-1818. ‐ ‐ ‐ D-126